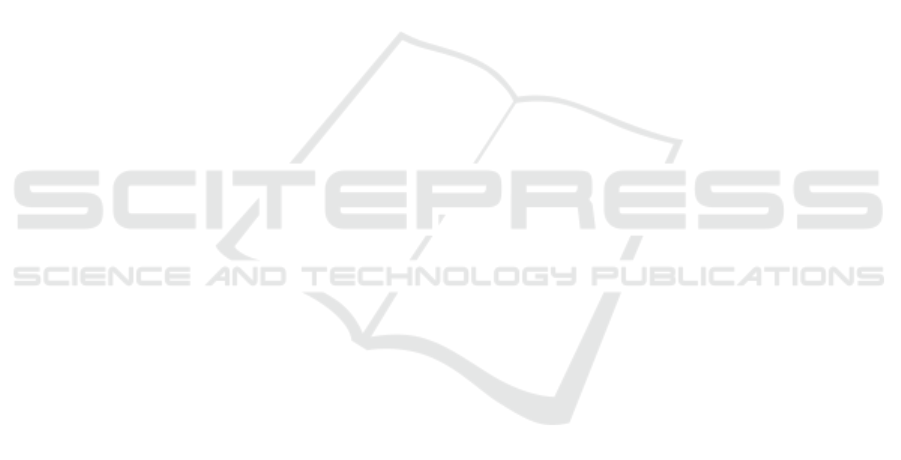
REFERENCES
Bos, D. O. et al. (2006). Eeg-based emotion recognition.
The Influence of Visual and Auditory Stimuli, 56(3):1–
17.
Chai, X., Wang, Q., Zhao, Y., Li, Y., Liu, D., Liu, X.,
and Bai, O. (2017). A fast, efficient domain adap-
tation technique for cross-domain electroencephalog-
raphy (eeg)-based emotion recognition. Sensors,
17(5):1014.
Coan, J. A. and Allen, J. J. (2004). Frontal eeg asymmetry
as a moderator and mediator of emotion. Biological
psychology, 67(1-2):7–50.
Duan, R.-N., Zhu, J.-Y., and Lu, B.-L. (2013). Differential
entropy feature for eeg-based emotion classification.
In 2013 6th International IEEE/EMBS Conference on
Neural Engineering (NER), pages 81–84. IEEE.
Ganin, Y., Ustinova, E., Ajakan, H., Germain, P.,
Larochelle, H., Laviolette, F., Marchand, M., and
Lempitsky, V. (2016). Domain-adversarial training of
neural networks. The Journal of Machine Learning
Research, 17(1):2096–2030.
Goodfellow, I., Pouget-Abadie, J., Mirza, M., Xu, B.,
Warde-Farley, D., Ozair, S., Courville, A., and Ben-
gio, Y. (2014). Generative adversarial nets. In
Advances in neural information processing systems,
pages 2672–2680.
Guger, C., Ramoser, H., and Pfurtscheller, G. (2000). Real-
time eeg analysis with subject-specific spatial patterns
for a brain-computer interface (bci). IEEE transac-
tions on rehabilitation engineering, 8(4):447–456.
Jayaram, V., Alamgir, M., Altun, Y., Scholkopf, B., and
Grosse-Wentrup, M. (2016). Transfer learning in
brain-computer interfaces. IEEE Computational In-
telligence Magazine, 11(1):20–31.
Kahou, S. E., Bouthillier, X., Lamblin, P., Gulcehre,
C., Michalski, V., Konda, K., Jean, S., Froumenty,
P., Dauphin, Y., Boulanger-Lewandowski, N., et al.
(2016). Emonets: Multimodal deep learning ap-
proaches for emotion recognition in video. Journal
on Multimodal User Interfaces, 10(2):99–111.
Koelstra, S., Muhl, C., Soleymani, M., Lee, J.-S., Yazdani,
A., Ebrahimi, T., Pun, T., Nijholt, A., and Patras, I.
(2011). Deap: A database for emotion analysis; using
physiological signals. IEEE transactions on affective
computing, 3(1):18–31.
Li, X., Song, D., Zhang, P., Zhang, Y., Hou, Y., and Hu, B.
(2018). Exploring eeg features in cross-subject emo-
tion recognition. Frontiers in neuroscience, 12:162.
Luo, Y., Zhang, S.-Y., Zheng, W.-L., and Lu, B.-L.
(2018). Wgan domain adaptation for eeg-based emo-
tion recognition. In International Conference on Neu-
ral Information Processing, pages 275–286. Springer.
Maaten, L. v. d. and Hinton, G. (2008). Visualizing data
using t-sne. Journal of machine learning research,
9(Nov):2579–2605.
Margolis, A. (2011). A literature review of domain adapta-
tion with unlabeled data. Tec. Report, pages 1–42.
Pan, S. J. and Yang, Q. (2009). A survey on transfer learn-
ing. IEEE Transactions on knowledge and data engi-
neering, 22(10):1345–1359.
Zhao, H., Zhang, S., Wu, G., Gordon, G. J., et al. (2018).
Multiple source domain adaptation with adversarial
learning.
Zheng, W.-L. and Lu, B.-L. (2015). Investigating criti-
cal frequency bands and channels for eeg-based emo-
tion recognition with deep neural networks. IEEE
Transactions on Autonomous Mental Development,
7(3):162–175.
Zheng, W.-L. and Lu, B.-L. (2016). Personalizing eeg-
based affective models with transfer learning. In Pro-
ceedings of the Twenty-Fifth International Joint Con-
ference on Artificial Intelligence, pages 2732–2738.
Cross-phase Emotion Recognition using Multiple Source Domain Adaptation
157