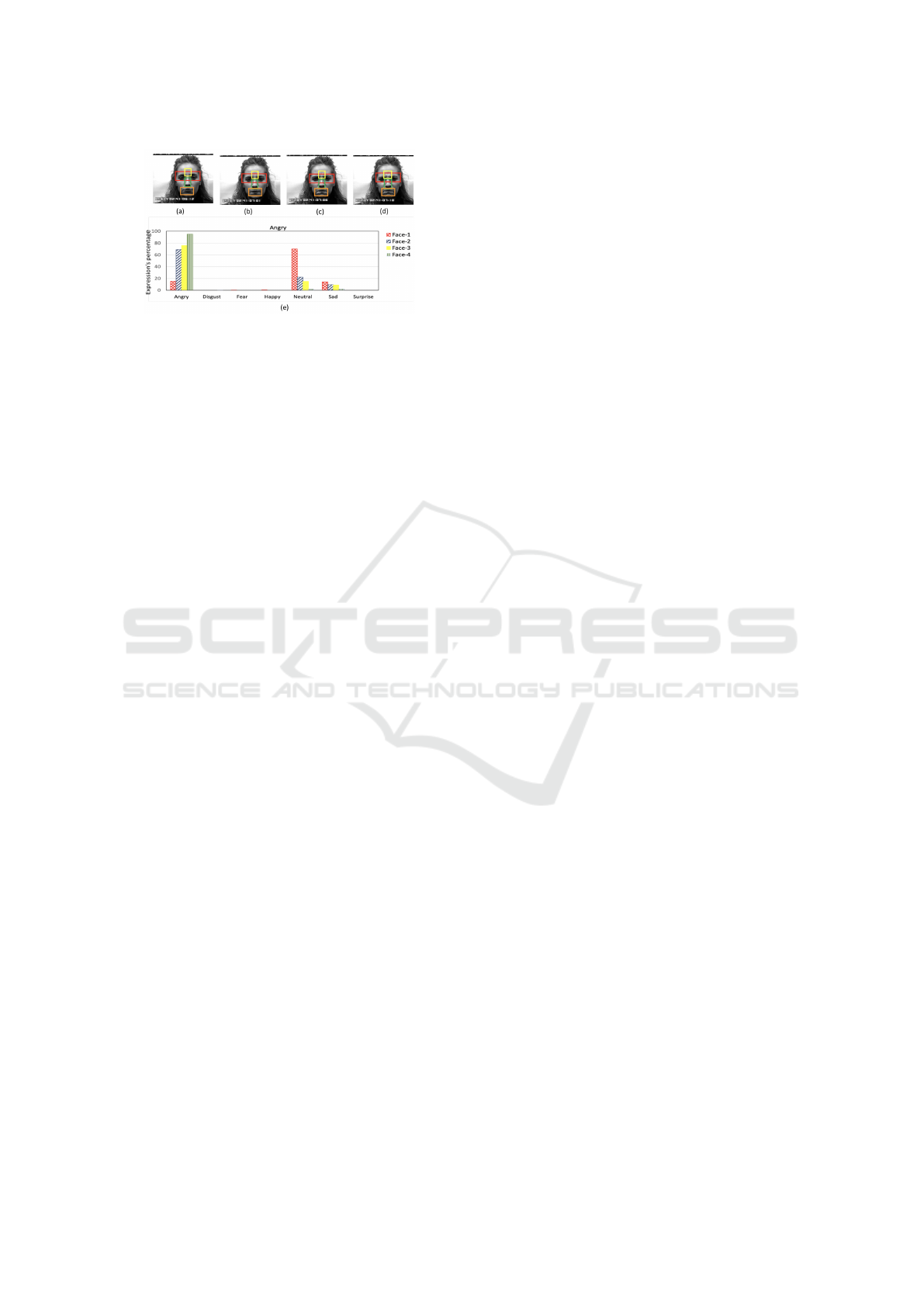
Figure 11: (a) (b) (c) (d) Shows the expression of the an-
gry face from the lower level to the extreme level, and
(e) Graphical representation of expression percentages and
how other expressions influence while the expression level
changes from low to high.
Capability of our proposed method is to recognize
a facial expression of a person using partial informa-
tion from the given whole face image. The proposed
method is applied to the most informative regions of
the face, i.e., forehead, eyes, nose, and lips. It is ob-
served that a combination of these regions is useful
enough to distinguish facial expressions of different
persons or the same persons in most of the cases. The
result obtained by the proposed method is comparable
with the most of the state of the art methods.
REFERENCES
Dalal, N. and Triggs, B. (2005). Histograms of oriented gra-
dients for human detection. In Computer Vision and
Pattern Recognition, 2005. CVPR 2005. IEEE Com-
puter Society Conference on, volume 1, pages 886–
893. IEEE.
King, D. E. (2009). Dlib-ml: A machine learning
toolkit. Journal of Machine Learning Research,
10(Jul):1755–1758.
Liu, C. and Wechsler, H. (2002). Gabor feature based classi-
fication using the enhanced fisher linear discriminant
model for face recognition. IEEE Transactions on Im-
age processing, 11(4):467–476.
Lucey, P., Cohn, J. F., Kanade, T., Saragih, J., Ambadar,
Z., and Matthews, I. (2010). The extended cohn-
kanade dataset (ck+): A complete dataset for action
unit and emotion-specified expression. In Computer
Vision and Pattern Recognition Workshops (CVPRW),
2010 IEEE Computer Society Conference on, pages
94–101. IEEE.
Lyons, M., Akamatsu, S., Kamachi, M., and Gyoba,
J. (1998). Coding facial expressions with gabor
wavelets. In Automatic Face and Gesture Recognition,
1998. Proceedings. Third IEEE International Confer-
ence on, pages 200–205. IEEE.
Mase, K. (1991). Recognition of facial expression from
optical flow. IEICE TRANSACTIONS on Information
and Systems, 74(10):3474–3483.
Shikkenawis, G. and Mitra, S. K. (2016). On some vari-
ants of locality preserving projection. Neurocomput-
ing, 173:196–211.
Simard, P. Y., Steinkraus, D., and Platt, J. C. (2003). Best
practices for convolutional neural networks applied to
visual document analysis. In null, page 958. IEEE.
Simonyan, K. and Zisserman, A. (2014). Very deep con-
volutional networks for large-scale image recognition.
arXiv preprint arXiv:1409.1556.
Sujata and Mitra, S. K. (2020). Dnnfg: Dnn based on fourier
transform followed by gabor filtering for the modular
fer. In ICPRAM, pages 212–219.
Sun, Y., Liang, D., Wang, X., and Tang, X. (2015).
Deepid3: Face recognition with very deep neural net-
works. arXiv preprint arXiv:1502.00873.
Valstar, M., Pantic, M., and Patras, I. (2004). Motion history
for facial action detection in video. In 2004 IEEE In-
ternational Conference on Systems, Man and Cyber-
netics (IEEE Cat. No. 04CH37583), volume 1, pages
635–640. IEEE.
Walecki, R., Rudovic, O., Pavlovic, V., and Pantic, M.
(2015). Variable-state latent conditional random fields
for facial expression recognition and action unit detec-
tion. In 2015 11th IEEE International Conference and
Workshops on Automatic Face and Gesture Recogni-
tion (FG), volume 1, pages 1–8. IEEE.
Zhang, T. (2017). Facial expression recognition based on
deep learning: A survey. In International Conference
on Intelligent and Interactive Systems and Applica-
tions, pages 345–352. Springer.
Zhang, W., Zhang, Y., Ma, L., Guan, J., and Gong, S.
(2015). Multimodal learning for facial expression
recognition. Pattern Recognition, 48(10):3191–3202.
Zhao, G., Huang, X., Taini, M., Li, S. Z., and Pietik
¨
aInen,
M. (2011). Facial expression recognition from
near-infrared videos. Image and Vision Computing,
29(9):607–619.
Zhong, L., Liu, Q., Yang, P., Huang, J., and Metaxas, D. N.
(2014). Learning multiscale active facial patches for
expression analysis. IEEE transactions on cybernet-
ics, 45(8):1499–1510.
VISAPP 2021 - 16th International Conference on Computer Vision Theory and Applications
284