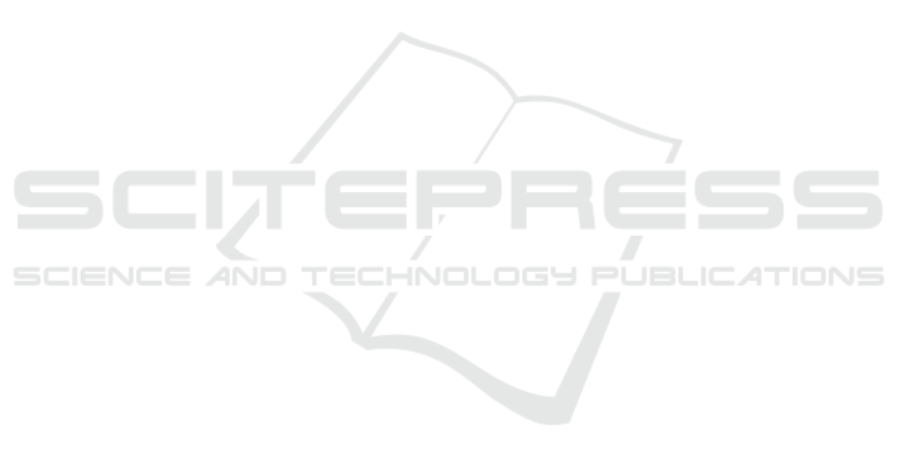
Dai, J., Li, Y., He, K., and Sun, J. (2016). R-FCN: Ob-
ject Detection via Region-based Fully Convolutional
Networks. arXiv:1605.06409 [cs]. arXiv: 1605.06409.
Duan, K., Bai, S., Xie, L., Qi, H., Huang, Q., and Tian, Q.
(2019). CenterNet: Keypoint Triplets for Object De-
tection. In 2019 IEEE/CVF International Conference
on Computer Vision (ICCV), pages 6568–6577, Seoul,
Korea (South). IEEE.
Duan, K., Xie, L., Qi, H., Bai, S., Huang, Q., and Tian, Q.
(2020a). Corner Proposal Network for Anchor-free,
Two-stage Object Detection. arXiv:2007.13816 [cs].
arXiv: 2007.13816.
Duan, Z., Ozan Tezcan, M., Nakamura, H., Ishwar, P., and
Konrad, J. (2020b). RAPiD: Rotation-Aware Peo-
ple Detection in Overhead Fisheye Images. In 2020
IEEE/CVF Conference on Computer Vision and Pat-
tern Recognition Workshops (CVPRW), pages 2700–
2709, Seattle, WA, USA. IEEE.
Fu, C.-Y., Liu, W., Ranga, A., Tyagi, A., and Berg, A. C.
(2017). DSSD : Deconvolutional Single Shot Detector.
arXiv:1701.06659 [cs]. arXiv: 1701.06659.
He, K., Zhang, X., Ren, S., and Sun, J. (2015).
Deep Residual Learning for Image Recognition.
arXiv:1512.03385 [cs]. arXiv: 1512.03385.
Hua, B.-S., Pham, Q.-H., Nguyen, D. T., Tran, M.-K., Yu,
L.-F., and Yeung, S.-K. (2016). SceneNN: A Scene
Meshes Dataset with aNNotations. In 2016 Fourth
International Conference on 3D Vision (3DV), pages
92–101, Stanford, CA, USA. IEEE.
Kong, T., Sun, F., Liu, H., Jiang, Y., Li, L., and Shi, J.
(2020). FoveaBox: Beyound Anchor-Based Object
Detection. IEEE Transactions on Image Processing,
29:7389–7398.
Law, H. and Deng, J. (2020). CornerNet: Detecting Ob-
jects as Paired Keypoints. International Journal of
Computer Vision, 128(3):642–656.
Li, W., Li, F., Luo, Y., and Wang, P. (2020). Deep
Domain Adaptive Object Detection: a Survey.
arXiv:2002.06797 [cs, eess]. arXiv: 2002.06797.
Lin, T.-Y., Goyal, P., Girshick, R., He, K., and Dollar, P.
(2017). Focal Loss for Dense Object Detection. In 2017
IEEE International Conference on Computer Vision
(ICCV), pages 2999–3007, Venice. IEEE.
Lin, T.-Y., Maire, M., Belongie, S., Hays, J., Perona, P.,
Ramanan, D., Doll
´
ar, P., and Zitnick, C. L. (2014). Mi-
crosoft COCO: Common Objects in Context. In Fleet,
D., Pajdla, T., Schiele, B., and Tuytelaars, T., editors,
Computer Vision – ECCV 2014, volume 8693, pages
740–755. Springer International Publishing, Cham.
Liu, W., Anguelov, D., Erhan, D., Szegedy, C., Reed, S., Fu,
C.-Y., and Berg, A. C. (2016). SSD: Single Shot Multi-
Box Detector. In Leibe, B., Matas, J., Sebe, N., and
Welling, M., editors, Computer Vision – ECCV 2016,
volume 9905, pages 21–37. Springer International Pub-
lishing, Cham.
McCormac, J., Handa, A., Leutenegger, S., and Davison,
A. J. (2017). SceneNet RGB-D: Can 5M Synthetic
Images Beat Generic ImageNet Pre-training on Indoor
Segmentation? In 2017 IEEE International Conference
on Computer Vision (ICCV), pages 2697–2706, Venice.
IEEE.
Newell, A., Yang, K., and Deng, J. (2016). Stacked Hour-
glass Networks for Human Pose Estimation. In Leibe,
B., Matas, J., Sebe, N., and Welling, M., editors, Com-
puter Vision – ECCV 2016, volume 9912, pages 483–
499. Springer International Publishing, Cham. Series
Title: Lecture Notes in Computer Science.
Redmon, J. and Farhadi, A. (2016). YOLO9000: Bet-
ter, Faster, Stronger. arXiv:1612.08242 [cs]. arXiv:
1612.08242.
Ren, S., He, K., Girshick, R., and Sun, J. (2017). Faster
R-CNN: Towards Real-Time Object Detection with
Region Proposal Networks. IEEE Transactions on
Pattern Analysis and Machine Intelligence, 39(6):1137–
1149.
Richter, S. R., Vineet, V., Roth, S., and Koltun, V. (2016).
Playing for Data: Ground Truth from Computer Games.
In Leibe, B., Matas, J., Sebe, N., and Welling, M., edi-
tors, Computer Vision – ECCV 2016, volume 9906,
pages 102–118. Springer International Publishing,
Cham. Series Title: Lecture Notes in Computer Sci-
ence.
Ros, G., Sellart, L., Materzynska, J., Vazquez, D., and Lopez,
A. M. (2016). The SYNTHIA Dataset: A Large Col-
lection of Synthetic Images for Semantic Segmentation
of Urban Scenes. In 2016 IEEE Conference on Com-
puter Vision and Pattern Recognition (CVPR), pages
3234–3243, Las Vegas, NV, USA. IEEE.
Scheck, T., Seidel, R., and Hirtz, G. (2020). Learning from
THEODORE: A Synthetic Omnidirectional Top-View
Indoor Dataset for Deep Transfer Learning. In 2020
IEEE Winter Conference on Applications of Computer
Vision (WACV), pages 932–941, Snowmass Village,
CO, USA. IEEE.
Tian, Z., Shen, C., Chen, H., and He, T. (2019). FCOS:
Fully Convolutional One-Stage Object Detection.
arXiv:1904.01355 [cs]. arXiv: 1904.01355.
Varol, G., Romero, J., Martin, X., Mahmood, N., Black,
M. J., Laptev, I., and Schmid, C. (2017). Learning
from Synthetic Humans. In 2017 IEEE Conference
on Computer Vision and Pattern Recognition (CVPR),
pages 4627–4635, Honolulu, HI. IEEE.
Vu, T.-H., Jain, H., Bucher, M., Cord, M., and P
´
erez, P.
(2019). Advent: Adversarial entropy minimization for
domain adaptation in semantic segmentation. In Pro-
ceedings of the IEEE conference on computer vision
and pattern recognition, pages 2517–2526.
Yu, F., Wang, D., Shelhamer, E., and Darrell, T. (2019). Deep
Layer Aggregation. arXiv:1707.06484 [cs]. arXiv:
1707.06484.
Zhou, X., Wang, D., and Kr
¨
ahenb
¨
uhl, P. (2019a). Objects as
Points. arXiv:1904.07850 [cs]. arXiv: 1904.07850.
Zhou, X., Zhuo, J., and Kr
¨
ahenb
¨
uhl, P. (2019b). Bottom-
up Object Detection by Grouping Extreme and Center
Points. arXiv:1901.08043 [cs]. arXiv: 1901.08043.
Unsupervised Domain Adaptation from Synthetic to Real Images for Anchorless Object Detection
327