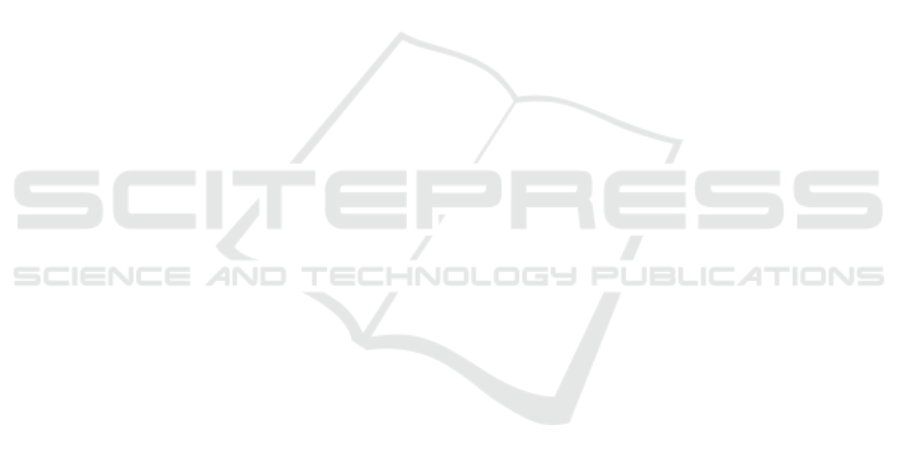
Fujiwara, T., Kwon, O.-H., and Ma, K.-L. (2019). Support-
ing analysis of dimensionality reduction results with
contrastive learning. IEEE transactions on visualiza-
tion and computer graphics, 26(1):45–55.
Gerych, W., Agu, E., and Rundensteiner, E. (2019). Clas-
sifying depression in imbalanced datasets using an
autoencoder-based anomaly detection approach. In
2019 IEEE 13th International Conference on Seman-
tic Computing (ICSC), pages 124–127. IEEE.
Harrower, M. and Brewer, C. A. (2003). Colorbrewer. org:
an online tool for selecting colour schemes for maps.
The Cartographic Journal, 40(1):27–37.
Hoque, E. and Carenini, G. (2015). Convisit: Interac-
tive topic modeling for exploring asynchronous on-
line conversations. In Proceedings of the 20th In-
ternational Conference on Intelligent User Interfaces,
pages 169–180.
Kwon, B. C., Eysenbach, B., Verma, J., Ng, K., De Filippi,
C., Stewart, W. F., and Perer, A. (2017). Clustervision:
Visual supervision of unsupervised clustering. IEEE
transactions on visualization and computer graphics,
24(1):142–151.
L’Yi, S., Ko, B., Shin, D., Cho, Y.-J., Lee, J., Kim, B.,
and Seo, J. (2015). Xclusim: a visual analytics
tool for interactively comparing multiple clustering
results of bioinformatics data. BMC bioinformatics,
16(S11):S5.
Maaten, L. v. d. and Hinton, G. (2008). Visualizing data
using t-sne. Journal of machine learning research,
9(Nov):2579–2605.
Madan, A., Cebrian, M., Moturu, S., Farrahi, K., et al.
(2011). Sensing the” health state” of a community.
IEEE Pervasive Computing, 11(4):36–45.
Mead, A. (1992). Review of the development of multidi-
mensional scaling methods. Journal of the Royal Sta-
tistical Society: Series D (The Statistician), 41(1):27–
39.
Mendes, E., Saad, L., and McGeeny, K. (2012).
https://news.gallup.com/poll/154685/stay-home-
moms-report-depression-sadness-anger.aspx.
Mohr, D. C., Zhang, M., and Schueller, S. M. (2017).
Personal sensing: understanding mental health using
ubiquitous sensors and machine learning. Annual re-
view of clinical psychology, 13:23–47.
Pu, J., Xu, P., Qu, H., Cui, W., Liu, S., and Ni, L. (2011).
Visual analysis of people’s mobility pattern from mo-
bile phone data. In Proceedings of the 2011 Visual In-
formation Communication-International Symposium,
page 13. ACM.
Ravesloot, C., Ward, B., Hargrove, T., Wong, J., Livingston,
N., Torma, L., and Ipsen, C. (2016). Why stay home?
temporal association of pain, fatigue and depression
with being at home. Disability and health journal,
9(2):218–225.
Restuccia, F., Ghosh, N., Bhattacharjee, S., Das, S. K., and
Melodia, T. (2017). Quality of information in mobile
crowdsensing: Survey and research challenges. ACM
Transactions on Sensor Networks (TOSN), 13(4):1–
43.
Rousseeuw, P. J. (1987). Silhouettes: a graphical aid to
the interpretation and validation of cluster analysis.
Journal of computational and applied mathematics,
20:53–65.
Sacha, D., Zhang, L., Sedlmair, M., Lee, J. A., Peltonen, J.,
Weiskopf, D., North, S. C., and Keim, D. A. (2016).
Visual interaction with dimensionality reduction: A
structured literature analysis. IEEE transactions on
visualization and computer graphics, 23(1):241–250.
Saeb, S., Zhang, M., Karr, C. J., Schueller, S. M., Corden,
M. E., Kording, K. P., and Mohr, D. C. (2015). Mo-
bile phone sensor correlates of depressive symptom
severity in daily-life behavior: an exploratory study.
Journal of medical Internet research, 17(7):e175.
Senaratne, H., Mueller, M., Behrisch, M., Lalanne, F.,
Bustos-Jim
´
enez, J., Schneidewind, J., Keim, D., and
Schreck, T. (2017). Urban mobility analysis with mo-
bile network data: a visual analytics approach. IEEE
Transactions on Intelligent Transportation Systems,
19(5):1537–1546.
Shen, Z. and Ma, K.-L. (2008). Mobivis: A visualization
system for exploring mobile data. In 2008 IEEE Pa-
cific Visualization Symposium, pages 175–182. IEEE.
Tenenbaum, J. B., De Silva, V., and Langford, J. C. (2000).
A global geometric framework for nonlinear dimen-
sionality reduction. science, 290(5500):2319–2323.
Vaizman, Y., Ellis, K., Lanckriet, G., and Weibel, N. (2018).
Extrasensory app: Data collection in-the-wild with
rich user interface to self-report behavior. In Proceed-
ings of the 2018 CHI Conference on Human Factors
in Computing Systems, pages 1–12.
Vetter, C. (2018). Circadian disruption: What do we actu-
ally mean? European Journal of Neuroscience.
Wang, R., Chen, F., Chen, Z., Li, T., Harari, G., Tignor, S.,
Zhou, X., Ben-Zeev, D., and Campbell, A. T. (2014).
Studentlife: assessing mental health, academic perfor-
mance and behavioral trends of college students using
smartphones. In Proceedings of the 2014 ACM inter-
national joint conference on pervasive and ubiquitous
computing, pages 3–14.
Wang, W., Mirjafari, S., Harari, G., Ben-Zeev, D., Brian,
R., Choudhury, T., Hauser, M., Kane, J., Masaba, K.,
Nepal, S., et al. (2020). Social sensing: Assessing so-
cial functioning of patients living with schizophrenia
using mobile phone sensing. In Proceedings of the
2020 CHI Conference on Human Factors in Comput-
ing Systems, pages 1–15.
Wenskovitch, J. and North, C. (2019). Pollux: Interac-
tive cluster-first projections of high-dimensional data.
In 2019 IEEE Visualization in Data Science (VDS),
pages 38–47. IEEE.
Weston, G., Zilanawala, A., Webb, E., Carvalho, L. A.,
and McMunn, A. (2019). Long work hours, week-
end working and depressive symptoms in men and
women: findings from a uk population-based study.
J Epidemiol Community Health, 73(5):465–474.
PLEADES: Population Level Observation of Smartphone Sensed Symptoms for In-the-wild Data using Clustering
75