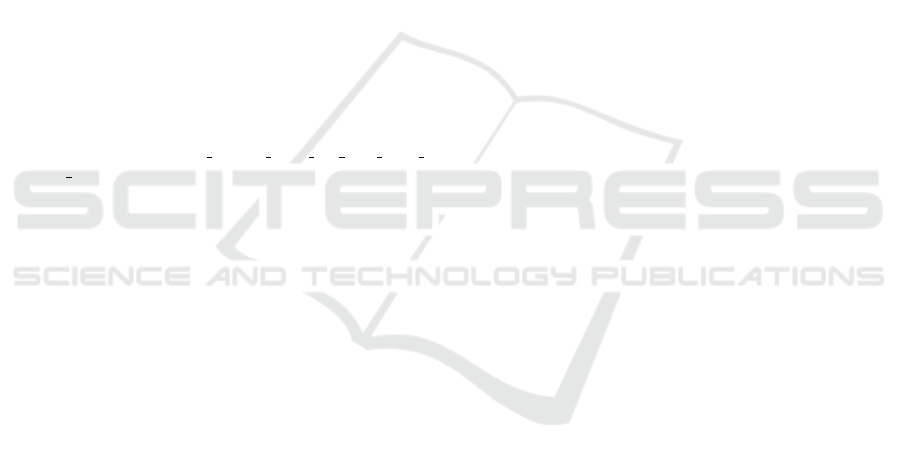
Alejo, D., Caballero, F., and Merino, L. (2017). Rgbd-
based robot localization in sewer networks. In
2017 IEEE/RSJ International Conference on Intelli-
gent Robots and Systems (IROS).
Alejo, D., Mier, G., Marques, C., Caballero, F., Merino, L.,
and Alvito, P. (2020). SIAR: A Ground Robot Solution
for Semi-autonomous Inspection of Visitable Sewers.
Springer International Publishing, Cham.
American Society of Civil Engineers (2017).
2017 infrastructure report card - wastewater.
https://www.infrastructurereportcard.org/wp-content/
uploads/2017/01/Wastewater-Final.pdf. Accessed:
14-11-2020.
Barnett, V. and Lewis, T. (1984). Outliers in Statistical
Data. Wiley Series in Probability and Statistics. Wi-
ley.
Beery, S., Liu, Y., Morris, D., Piavis, J., Kapoor, A., Meis-
ter, M., Joshi, N., and Perona, P. (2020). Synthetic
examples improve generalization for rare classes. In
2020 IEEE Winter Conference on Applications of
Computer Vision (WACV).
Bronstein, M. M., Bruna, J., LeCun, Y., Szlam, A., and Van-
dergheynst, P. (2017). Geometric deep learning: Go-
ing beyond euclidean data. IEEE Signal Processing
Magazine, 34(4).
CamBoard (2018). Development kit brief camboard pico
flexx. https://pmdtec.com/picofamily/wp-content/
uploads/2018/03/PMD DevKit Brief CB pico flexx
CE V0218-1.pdf. Accessed: 25-11-2020.
Cao, W., Yan, Z., He, Z., and He, Z. (2020). A comprehen-
sive survey on geometric deep learning. IEEE Access,
8.
Car, M., Markovic, L., Ivanovic, A., Orsag, M., and Bog-
dan, S. (2020). Autonomous wind-turbine blade in-
spection using lidar-equipped unmanned aerial vehi-
cle. IEEE Access, 8.
Duran, O., Althoefer, K., and Seneviratne, L. D. (2003).
Pipe inspection using a laser-based transducer and au-
tomated analysis techniques. IEEE/ASME Transac-
tions on Mechatronics, 8(3).
Duran, O., Althoefer, K., and Seneviratne, L. D. (2004).
Automated pipe inspection using ann and laser data
fusion. In IEEE International Conference on Robotics
and Automation, 2004. Proceedings. ICRA ’04. 2004,
volume 5.
Duran, O., Althoefer, K., and Seneviratne, L. D. (2007).
Automated pipe defect detection and categorization
using camera/laser-based profiler and artificial neural
network. IEEE Transactions on Automation Science
and Engineering, 4(1).
Garrido, G. G., Sattar, T., Corsar, M., James, R., and
Seghier, D. (2018). Towards safe inspection of long
weld lines on ship hulls using an autonomous robot. In
21st International Conference on Climbing and Walk-
ing Robots.
Hassan, S. I., Dang, L. M., Mehmood, I., Im, S., Choi, C.,
Kang, J., Park, Y.-S., and Moon, H. (2019). Under-
ground sewer pipe condition assessment based on con-
volutional neural networks. Automation in Construc-
tion, 106.
Haurum, J. B. and Moeslund, T. B. (2020). A survey on
image-based automation of cctv and sset sewer in-
spections. Automation in Construction, 111.
Henriksen, K. S., Lynge, M. S., Jeppesen, M. D. B., Al-
lahham, M. M. J., Nikolov, I. A., Haurum, J. B., and
Moeslund, T. B. (2020). Generating synthetic point
clouds of sewer networks: An initial investigation.
In Augmented Reality, Virtual Reality, and Computer
Graphics, Cham. Springer International Publishing.
Iyer, S., Sinha, S. K., Pedrick, M. K., and Tittmann, B. R.
(2012). Evaluation of ultrasonic inspection and imag-
ing systems for concrete pipes. Automation in Con-
struction, 22. Planning Future Cities-Selected papers
from the 2010 eCAADe Conference.
Khan, M. S. and Patil, R. (2018a). Acoustic characterization
of pvc sewer pipes for crack detection using frequency
domain analysis. In 2018 IEEE International Smart
Cities Conference (ISC2).
Khan, M. S. and Patil, R. (2018b). Statistical analysis of
acoustic response of pvc pipes for crack detection. In
SoutheastCon 2018.
Kolesnik, M. and Baratoff, G. (2000). Online distance
recovery for a sewer inspection robot. In Proceed-
ings 15th International Conference on Pattern Recog-
nition. ICPR-2000, volume 1.
Kumar, S. S., Wang, M., Abraham, D. M., Jahanshahi,
M. R., Iseley, T., and Cheng, J. C. P. (2020). Deep
learning–based automated detection of sewer
defects in cctv videos. Journal of Computing in Civil
Engineering, 34(1).
Lang, A. H., Vora, S., Caesar, H., Zhou, L., Yang, J.,
and Beijbom, O. (2019). Pointpillars: Fast encoders
for object detection from point clouds. In 2019
IEEE/CVF Conference on Computer Vision and Pat-
tern Recognition (CVPR).
Lepot, M., Stani
´
c, N., and Clemens, F. H. (2017). A tech-
nology for sewer pipe inspection (part 2): Experimen-
tal assessment of a new laser profiler for sewer defect
detection and quantification. Automation in Construc-
tion, 73.
Li, D., Cong, A., and Guo, S. (2019). Sewer damage detec-
tion from imbalanced cctv inspection data using deep
convolutional neural networks with hierarchical clas-
sification. Automation in Construction, 101.
Loshchilov, I. and Hutter, F. (2017). SGDR: stochastic gra-
dient descent with warm restarts. In 5th International
Conference on Learning Representations, ICLR 2017,
Toulon, France, April 24-26, 2017, Conference Track
Proceedings. OpenReview.net.
Menendez, E., Victores, J. G., Montero, R., Mart
´
ınez, S.,
and Balaguer, C. (2018). Tunnel structural inspection
and assessment using an autonomous robotic system.
Automation in Construction, 87.
Myrans, J., Everson, R., and Kapelan, Z. (2018). Auto-
mated detection of faults in sewers using cctv image
sequences. Automation in Construction, 95.
Nasrollahi, M., Bolourian, N., and Hammad, A. (2019).
Concrete surface defect detection using deep neural
network based on lidar scanning. In Proceedings of
Sewer Defect Classification using Synthetic Point Clouds
899