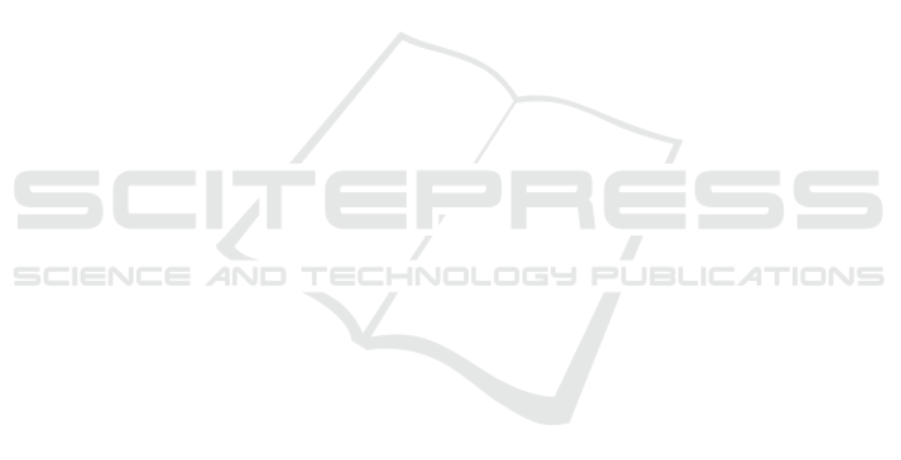
Imaging, Biomarkers and Lifestyle (AIBL) study of
aging: Methodology and baseline characteristics of
1112 individuals recruited for a longitudinal study of
Alzheimer’s Disease. International Psychogeriatrics,
21(4):672–687.
Fischl, B. (2012). FreeSurfer. NeuroImage, 62(2):774–781.
Fischl, B., Salat, D. H., Busa, E., Albert, M., Dieterich,
M., Haselgrove, C., van der Kouwe, A., Killiany, R.,
Kennedy, D., Klaveness, S., Montillo, A., Makris, N.,
Rosen, B., and Dale, A. M. (2002). Whole brain
segmentation: Automated labeling of neuroanatomi-
cal structures in the human brain. Neuron, 33(3):341–
355.
Friedman, J. H. (2001). Greedy function approximation:
A gradient boosting machine. Annals of Statistics,
29(5):1189–1232.
Goodfellow, I., Pouget-Abadie, J., Mirza, M., Xu, B.,
Warde-Farley, D., Ozair, S., Courville, A., and Ben-
gio, Y. (2014). Generative adversarial nets. In
Advances in neural information processing systems,
pages 2672–2680.
Grassi, M., Rouleaux, N., Caldirola, D., Loewenstein,
D., Schruers, K., Perna, G., Dumontier, M., and
Alzheimer’s Disease Neuroimaging Initiative (2019).
A novel ensemble-based Machine Learning algo-
rithm to predict the conversion from Mild Cogni-
tive Impairment to Alzheimer’s Disease using socio-
demographic characteristics, clinical information, and
neuropsychological measures. Frontiers in Neurology,
10:756.
Greenwell, B. (2020). fastshap: Fast Approximate Shapley
Values. Manual of R package v0.0.5 https://CRAN.R-
project.org/package=fastshap, Last accessed: 2020-
09-12.
Hammond, T. C., Xing, X., Wang, C., Ma, D., Nho, K.,
Crane, P. K., Elahi, F., Ziegler, D. A., Liang, G.,
Cheng, Q., Yanckello, L. M., Jacobs, N., and Lin, A.-
L. (2020). β-amyloid and tau drive early Alzheimer’s
Disease decline while glucose hypometabolism drives
late decline. Communications Biology, 3(1).
Hochreiter, S. and Schmidhuber, J. (1997). Long Short-
Term Memory. Neural computation, 9(8):1735–1780.
Jack, C. R., Barnes, J., Bernstein, M. A., Borowski, B. J.,
Brewer, J., Clegg, S., Dale, A. M., Carmichael, O.,
Ching, C., DeCarli, C., Desikan, R. S., Fennema-
Notestine, C., Fjell, A. M., Fletcher, E., Fox, N. C.,
Gunter, J., Gutman, B. A., Holland, D., Hua, X., Insel,
P., Kantarci, K., Killiany, R. J., Krueger, G., Leung,
K. K., Mackin, S., Maillard, P., Molone, I., Mattsson,
N., McEvoy, L., Modat, M., Mueller, S., Nosheny, R.,
Ourselin, S., Schuff, N., Senjem, M. L., Simonson,
A., Thompson, P. M., Rettmann, D., Vemuri, P., Wal-
hovd, K., Zhao, Y., Zuk, S., and Weiner, M. (2015).
Magnetic Resonance Imaging in ADNI. Alzheimer’s
& dementia, 11(7):740–756.
Kuhn, M. (2019). caret: Classification and Regres-
sion Training. Manual of R package v6.0-82
https://CRAN.R-project.org/package=caret, Last ac-
cessed: 2020-09-12.
LeCun, Y., Bengio, Y., and Hinton, G. (2015). Deep learn-
ing. Nature, 521(7553):436–444.
Li, M., Reisman, J., Morris-Eppolito, B., Qian, S. X.,
Kazis, L. E., Wolozin, B., Goldstein, L. E., and Xia,
W. (2020). Beneficial association of angiotensin-
converting enzyme inhibitors and statins on the occur-
rence of possible Alzheimer’s Disease after traumatic
brain injury. Alzheimer’s Research & Therapy, 12(1).
Liaw, A. and Wiener, M. (2002). Classification and Regres-
sion by randomForest. R News, 2(3):18–22.
Lloret, A., Esteve, D., Lloret, M.-A., Cervera-Ferri, A.,
Lopez, B., Nepomuceno, M., and Monllor, P. (2019).
When does Alzheimer’s Disease really start? The role
of biomarkers. International Journal of Molecular
Sciences, 20(22):5536.
Lundberg, S. M., Erion, G. G., and Lee, S. (2018).
Consistent individualized feature attribution for
tree ensembles. Computing Research Repository.
http://arxiv.org/abs/1802.03888, Last accessed: 2020-
09-13.
Lundberg, S. M. and Lee, S.-I. (2017). A unified ap-
proach to interpreting model predictions. In Guyon,
I., Luxburg, U. V., Bengio, S., Wallach, H., Fer-
gus, R., Vishwanathan, S., and Garnett, R., editors,
Advances in Neural Information Processing Systems,
volume 30, pages 4765–4774. Curran Associates, Inc.
Marcus, D. S., Fotenos, A. F., Csernansky, J. G., Morris,
J. C., and Buckner, R. L. (2010). Open Access Series
of Imaging Studies: Longitudinal MRI data in nonde-
mented and demented older adults. Journal of Cogni-
tive Neuroscience, 22(12):2677–2684.
McKay, M. D., Beckman, R. J., and Conover, W. J. (1979).
A Comparison of three methods for selecting values of
input variables in the analysis of output from a com-
puter code. Technometrics, 21(2):239–245.
Mo
ˇ
ckus, J. (1975). On bayesian methods for seeking the
extremum. In Proceedings of the Optimization Tech-
niques IFIP Technical Conference, pages 400–404.
Springer.
Mofrad, R. B., Schoonenboom, N. S., Tijms, B. M., Schel-
tens, P., Visser, P. J., Flier, W. M., and Teunissen, C. E.
(2018). Decision tree supports the interpretation of
CSF biomarkers in Alzheimer’s Disease. Alzheimer’s
& Dementia: Diagnosis, Assessment & Disease Mon-
itoring, 11(1):1–9.
Molnar, C. (2019). Interpretable Machine Learning:
A guide for making black box models explain-
able. https://christophm.github.io/interpretable-ml-
book/, Last accessed: 2020-08-30.
Patterson, C. (2018). World Alzheimer report
2018 - The state of the art of dementia re-
search: New frontiers. Alzheimer’s Dis-
ease International, London, Great Britain.
https://www.alz.co.uk/research/WorldAlzheimerRepo
rt2018.pdf, Last accessed: 2020-08-26.
Pelka, O., Friedrich, C. M., Nensa, F., M
¨
onninghoff, C.,
Bloch, L., J
¨
ockel, K.-H., Schramm, S., Sanchez Hoff-
mann, S., Winkler, A., Weimar, C., Jokisch, M., and
for the Alzheimer’s Disease Neuroimaging Initiative
(2020). Sociodemographic data and APOE- 4 aug-
mentation for MRI-based detection of amnestic Mild
Cognitive Impairment using deep learning systems.
PLOS ONE, 15(9):1–24.
HEALTHINF 2021 - 14th International Conference on Health Informatics
98