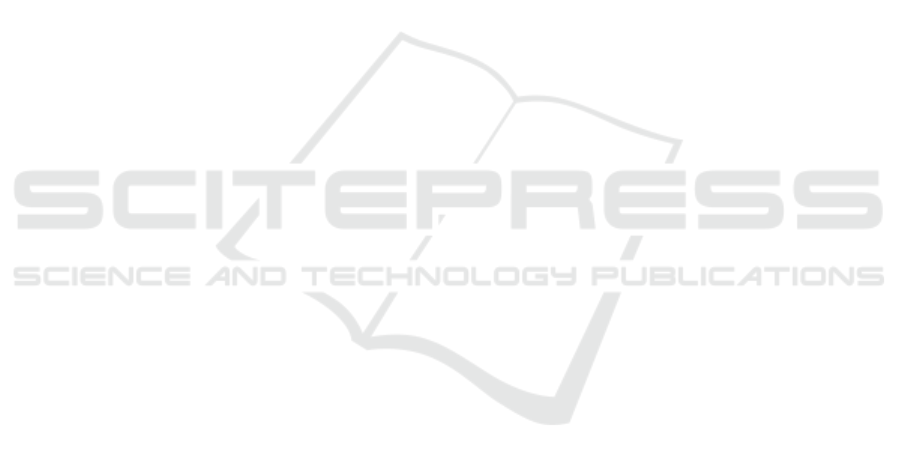
ACKNOWLEDGMENT
This work was supported in part by a UKIERI grant,
ID ‘DST UKIERI-2018-19-011’, and in part by an
Institutional Links grant, ID 429715093, under the
Newton Programme Vietnam partnership.
REFERENCES
Ahlswede, R., Ning Cai, Li, S. . R., and Yeung, R. W.
(2000). Network information flow. IEEE Transac-
tions on Information Theory, 46(4):1204–1216.
Bender, W., Gruhl, D., Morimoto, N., and Lu, A. (1996).
Techniques for data hiding. IBM Systems Journal,
35(3.4):313–336.
Chen, P.-Y. and Lin, H.-J. (2006). A DWT based approach
for image steganography. International Journal of Ap-
plied Science and Engineering, pages 275–290.
Duchon, C. E. (1979). Lanczos Filtering in One and
Two Dimensions. Journal of Applied Meteorology,
18(8):1016–1022.
Franz, E., Jerichow, A., M
¨
oller, S., Pfitzmann, A., and
Stierand, I. (1996). Computer based steganography:
How it works and why therefore any restrictions on
cryptography are nonsense, at best. In Anderson, R.,
editor, Information Hiding, pages 7–21, Berlin, Hei-
delberg. Springer Berlin Heidelberg.
Goodfellow, I., Bengio, Y., and Courville, A. (2016). Deep
learning. MIT press.
Grubinger, M., Clough, P. D., M
¨
uller, H., and Deselaers,
T. (2006). The IAPR TC-12 benchmark: A new
evaluation resource for visual information systems.
https://www.imageclef.org/photodata.
Guerrini, F., Dalai, M., and Leonardi, R. (2020). Minimal
information exchange for secure image hash-based
geometric transformations estimation. IEEE Transac-
tions on Information Forensics and Security, 15:3482–
3496.
Haris, M., Shakhnarovich, G., and Ukita, N. (2018). Deep
back-projection networks for super-resolution. In
2018 IEEE/CVF Conference on Computer Vision and
Pattern Recognition, pages 1664–1673.
Irani, M. and Peleg, S. (1991). Improving resolution by
image registration. CVGIP: Graphical Models and
Image Processing, 53(3):231 – 239.
Keys, R. (1981). Cubic convolution interpolation for digital
image processing. IEEE Transactions on Acoustics,
Speech, and Signal Processing, 29(6):1153–1160.
Kim, J., Kwon Lee, J., and Mu Lee, K. (2016). Accurate
image super-resolution using very deep convolutional
networks. In Proceedings of the IEEE conference on
computer vision and pattern recognition, pages 1646–
1654.
Koetter, R. and Medard, M. (2003). An algebraic approach
to network coding. IEEE/ACM Transactions on Net-
working, 11(5):782–795.
Lai, W., Huang, J., Ahuja, N., and Yang, M. (2017).
Deep laplacian pyramid networks for fast and accurate
super-resolution. In 2017 IEEE Conference on Com-
puter Vision and Pattern Recognition (CVPR), pages
5835–5843.
Li, Y., Liu, D., Li, H., Li, L., Li, Z., and Wu, F. (2019a).
Learning a convolutional neural network for image
compact-resolution. IEEE Transactions on Image
Processing, 28(3):1092–1107.
Li, Z., Yang, J., Liu, Z., Yang, X., Jeon, G., and Wu,
W. (2019b). Feedback network for image super-
resolution. In 2019 IEEE/CVF Conference on Com-
puter Vision and Pattern Recognition (CVPR), pages
3862–3871.
Peng, H., Yang, B., Li, L., and Yang, Y. (2020).
Secure and traceable image transmission scheme
based on semitensor product compressed sensing in
telemedicine system. IEEE Internet of Things Jour-
nal, 7(3):2432–2451.
Smith, P. (1981). Bilinear interpolation of digital images.
Ultramicroscopy, 6(1):201 – 204.
Wang, Z., Chen, J., and Hoi, S. C. H. (2020). Deep learning
for image super-resolution: A survey. IEEE Trans-
actions on Pattern Analysis and Machine Intelligence,
pages 1–1.
Xie, X.-Z., Chang, C.-C., and Lin, C.-C. (2019).
Reversibility-oriented secret image sharing mecha-
nism with steganography and authentication based on
code division multiplexing. IET Image Processing,
13(9):1411–1420.
Zhang, K., Zuo, W., Chen, Y., Meng, D., and Zhang, L.
(2017). Beyond a Gaussian denoiser: Residual learn-
ing of deep CNN for image denoising. IEEE Transac-
tions on Image Processing, 26(7):3142–3155.
VISAPP 2021 - 16th International Conference on Computer Vision Theory and Applications
660