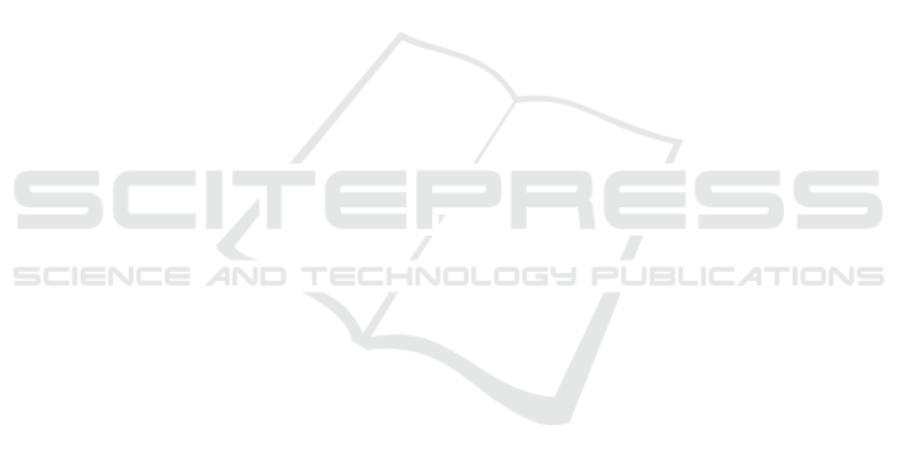
Coley, C. W., Jin, W., Rogers, L., Jamison, T. F., Jaakkola,
T. S., Green, W. H., Barzilay, R., and Jensen, K. F.
(2019). A graph-convolutional neural network model
for the prediction of chemical reactivity. Chemical
science, 10(2):370–377.
Cummins, M. and Newman, P. (2008). Fab-map: Proba-
bilistic localization and mapping in the space of ap-
pearance. Int. J. Robotics Research, 27(6):647–665.
Garcia-Fidalgo, E. and Ortiz, A. (2018). ibow-lcd: An
appearance-based loop-closure detection approach us-
ing incremental bags of binary words. IEEE Robotics
and Automation Letters, 3(4):3051–3057.
Gawel, A., Del Don, C., Siegwart, R., Nieto, J., and Cadena,
C. (2018). X-view: Graph-based semantic multi-view
localization. IEEE Robotics and Automation Letters,
3(3):1687–1694.
Himstedt, M. and Maehle, E. (2017). Semantic monte-
carlo localization in changing environments using rgb-
d cameras. In 2017 European Conference on Mobile
Robots (ECMR), pages 1–8. IEEE.
Hinton, G., Vinyals, O., and Dean, J. (2015). Distilling
the knowledge in a neural network. arXiv preprint
arXiv:1503.02531.
Hsu, D. F. and Taksa, I. (2005). Comparing rank and score
combination methods for data fusion in information
retrieval. Information retrieval, 8(3):449–480.
Hu, H., Wang, H., Liu, Z., Yang, C., Chen, W., and
Xie, L. (2019). Retrieval-based localization based on
domain-invariant feature learning under changing en-
vironments. In IEEE/RSJ Int. Conf. Intelligent Robots
and Systems (IROS), pages 3684–3689.
Imhof, M. and Braschler, M. (2018). A study of untrained
models for multimodal information retrieval. Infor-
mation Retrieval Journal, 21(1):81–106.
Kim, G., Park, B., and Kim, A. (2019). 1-day learning, 1-
year localization: Long-term lidar localization using
scan context image. IEEE Robotics and Automation
Letters, 4(2):1948–1955.
Maddern, W., Pascoe, G., Linegar, C., and Newman, P.
(2017). 1 Year, 1000km: The Oxford RobotCar
Dataset. The International Journal of Robotics Re-
search (IJRR), 36(1):3–15.
Merrill, N. and Huang, G. (2019). CALC2.0: Com-
bining appearance, semantic and geometric informa-
tion for robust and efficient visual loop closure. In
IEEE/RSJ Int. Conf. Intelligent Robots and Systems
(IROS), Macau, China.
Milford, M. J. and Wyeth, G. F. (2012). Seqslam: Vi-
sual route-based navigation for sunny summer days
and stormy winter nights. In 2012 IEEE Int. Conf.
Robotics and Automation, pages 1643–1649. IEEE.
Naseer, T., Spinello, L., Burgard, W., and Stachniss, C.
(2014). Robust visual robot localization across sea-
sons using network flows. In AAAI, pages 2564–2570.
Neira, J., Tard
´
os, J. D., and Castellanos, J. A. (2003). Linear
time vehicle relocation in slam. In ICRA, pages 427–
433. Citeseer.
Raguram, R., Chum, O., Pollefeys, M., Matas, J., and
Frahm, J.-M. (2012). Usac: a universal framework for
random sample consensus. IEEE transactions on pat-
tern analysis and machine intelligence, 35(8):2022–
2038.
Sivic, J. and Zisserman, A. (2003). Video google: A text
retrieval approach to object matching in videos. In
null, page 1470.
Steinlechner, H., Haaser, G., Maierhofer, S., and Tobler,
R. F. (2019). Attribute grammars for incremental
scene graph rendering. In VISIGRAPP (1: GRAPP),
pages 77–88.
S
¨
underhauf, N., Shirazi, S., Dayoub, F., Upcroft, B., and
Milford, M. (2015). On the performance of convnet
features for place recognition. In IEEE/RSJ Int. Conf.
Intelligent Robots and Systems (IROS), pages 4297–
4304.
Wang, M., Yu, L., Zheng, D., Gan, Q., Gai, Y., Ye, Z., Li,
M., Zhou, J., Huang, Q., Ma, C., Huang, Z., Guo, Q.,
Zhang, H., Lin, H., Zhao, J., Li, J., Smola, A. J., and
Zhang, Z. (2019). Deep graph library: Towards ef-
ficient and scalable deep learning on graphs. ICLR
Workshop on Representation Learning on Graphs and
Manifolds.
Ying, R., He, R., Chen, K., Eksombatchai, P., Hamilton,
W. L., and Leskovec, J. (2018). Graph convolutional
neural networks for web-scale recommender systems.
In Proceedings of the 24th ACM SIGKDD Int. Conf.
Knowledge Discovery & Data Mining, pages 974–
983.
Zhang, L. and Zhu, Z. (2019). Unsupervised feature learn-
ing for point cloud understanding by contrasting and
clustering using graph convolutional neural networks.
In IEEE Int. Conf. 3D Vision, pages 395–404.
VISAPP 2021 - 16th International Conference on Computer Vision Theory and Applications
868