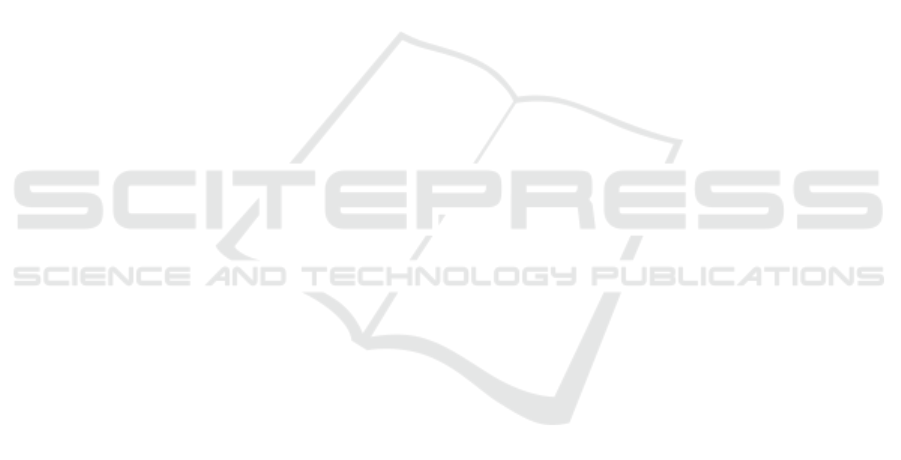
New York, NY, USA. Association for Computing Ma-
chinery.
Du W, Calhoun VD, L. H. e. a. (2012). High classifica-
tion accuracy for schizophrenia with rest and task fmri
data. Front Hum Neurosci, 6.
Fan, J., Gong, W., and Zhu, Z. (2019). Generalized high-
dimensional trace regression via nuclear norm regular-
ization. Journal of Econometrics, 212(1):177—-202.
Grover, A. and Leskovec, J. (2016). Node2vec: Scal-
able feature learning for networks. In Proceedings of
the 22nd ACM SIGKDD International Conference on
Knowledge Discovery and Data Mining, pages 855—-
864, New York, NY, USA. Association for Computing
Machinery.
Hamilton, W., Ying, Z., and Leskovec, J. (2017). Inductive
representation learning on large graphs. In Guyon, I.,
Luxburg, U. V., Bengio, S., Wallach, H., Fergus, R.,
Vishwanathan, S., and Garnett, R., editors, Advances
in Neural Information Processing Systems 30, pages
1024–1034. Curran Associates, Inc.
Khosla, M., Setty, V., and Anand, A. (2020). A comparative
study for unsupervised network representation learn-
ing. IEEE Transactions on Knowledge and Data En-
gineering, pages 1—-1.
Kiehl, KA, P. G. and VD, C. (2008). A review of
challenges in the use of fmri for disease classifica-
tion/characterization and a projection pursuit applica-
tion from a multi-site fmri schizophrenia study. Brain
Imaging Behav, 2(3):147–226.
Kipf, T. and Welling, M. (2016). Variational graph auto-
encoders. ArXiv.
Koltchinskii, V., Lounici, K., and Tsybakov, A. B. (2011).
Nuclear-norm penalization and optimal rates for noisy
low-rank matrix completion. The Annals of Statistics,
39(5):2302—-2329.
Li, Y., Yang, M., and Zhang, Z. (2019). A survey of multi-
view representation learning. IEEE Transactions
on Knowledge and Data Engineering, 31(10):1863–
1883.
Liu, S., Cai, W., Liu, S., Zhang, F., Fulham, M., Feng, D.,
Pujol, S., and Kikinis, R. (2015). Multimodal neu-
roimaging computing: a review of the applications in
neuropsychiatric disorders. Brain Inf, 2:167—-180.
M Symms, HR Jager, K. S. and Yousry, T. (2004). A re-
view of structural magnetic resonance neuroimaging.
J Neurol Neurosurg Psychiatry, 75:235—-1244.
Mihalik, A., Ferreira, F. S., and et al, R. (2019). Brain-
behaviour modes of covariation in healthy and clini-
cally depressed young people. Scientific reports, 9:1–
11.
Mikolov, T., Chen, K., Corrado, G., and Dean, J. (2013).
Efficient estimation of word representations in vector
space.
Ngiam, J., Khosla, A., Kim, M., Nam, J., Lee, H., and Ng,
A. Y. (2011). Multimodal deep learning. In Proceed-
ings of the 28th International Conference on Inter-
national Conference on Machine Learning, ICML’11,
pages 689—-696, Madison, WI, USA. Omnipress.
Pan, S., Hu, R., Long, G., Jiang, J., Yao, L., and Zhang, C.
(2018). Adversarially regularized graph autoencoder.
CoRR.
Perozzi, B., Al-Rfou, R., and Skiena, S. (2014). Deepwalk.
Proceedings of the 20th ACM SIGKDD international
conference on Knowledge discovery and data mining
- KDD ’14.
Saygin, Z., Osher, D. E., Koldewyn, K., Reynolds, G.,
Gabrieli, J., and Saxe, R. (2012). Anatomical connec-
tivity patterns predict face-selectivity in the fusiform
gyrus. Nature neuroscience, 15:321–327.
Sellami, A., Abbes, A. B., Barra, V., and Farah, I. R. (2020).
Fused 3-d spectral-spatial deep neural networks and
spectral clustering for hyperspectral image classifica-
tion. Pattern Recognition Letters, 138:594–600.
Sellami, A. and Farah, M. (2018). Comparative study of
dimensionality reduction methods for remote sensing
images interpretation. In 2018 4th International Con-
ference on Advanced Technologies for Signal and Im-
age Processing (ATSIP), pages 1–6. IEEE.
Sellami, A., Farah, M., Farah, I. R., and Solaiman, B.
(2019). Hyperspectral imagery classification based on
semi-supervised 3-d deep neural network and adap-
tive band selection. Expert Systems with Applications,
129:246–259.
Slawski, M., Li, P., and Hein, M. (2015). Regularization-
free estimation in trace regression with symmet-
ric positive semidefinite matrices. In Cortes, C.,
Lawrence, N. D., Lee, D. D., Sugiyama, M., and Gar-
nett, R., editors, Advances in Neural Information Pro-
cessing Systems 28, pages 2782–2790. Curran Asso-
ciates, Inc.
Sui, J., Adali, T., Yu, Q., Chen, J., and Calhoun, V. D.
(2012). A review of multivariate methods for multi-
modal fusion of brain imaging data. Journal of Neu-
roscience Methods, 204(1):68–81.
Sui, J., Pearlson, G. D., Du, Y., Yu, Q., Jones, T. R., Chen,
J., Jiang, T., Bustillo, J., and Calhoun, V. D. (2015).
In search of multimodal neuroimaging biomarkers of
cognitive deficits in schizophrenia. Biological Psychi-
atry, 78(11):794–804.
Tavor, I., Jones, O. P., Mars, R. B., Smith, S. M., Behrens,
T. E., and Jbabdi, S. (2016). Task-free mri predicts in-
dividual differences in brain activity during task per-
formance. Science, 352:216–220.
Wang, C., Pan, S., Long, G., Zhu, X., and Jiang, J. (2017).
Mgae: Marginalized graph autoencoder for graph
clustering. Proceedings of the 2017 ACM on Confer-
ence on Information and Knowledge Management.
Wu, Z., Pan, S., Chen, F., Long, G., Zhang, C., and Yu,
P. S. (2020). A comprehensive survey on graph neural
networks. IEEE Transactions on Neural Networks and
Learning Systems, pages 1–21.
Yang, X., Ramesh, P., Chitta, R., Madhvanath, S., Bernal,
E. A., and Luo, J. (2017). Deep multimodal represen-
tation learning from temporal data. IEEE Conference
on Computer Vision and Pattern Recognition (CVPR),
pages 5066–5074.
Zhang, Z., Cui, P., and Zhu, W. (2018). Deep learning on
graphs: A survey. CoRR.
Zhao, J., Xie, X., Xu, X., and Sun, S. (2017). Multi-view
learning overview: Recent progress and new chal-
lenges. Information Fusion, 38:43––54.
VISAPP 2021 - 16th International Conference on Computer Vision Theory and Applications
66