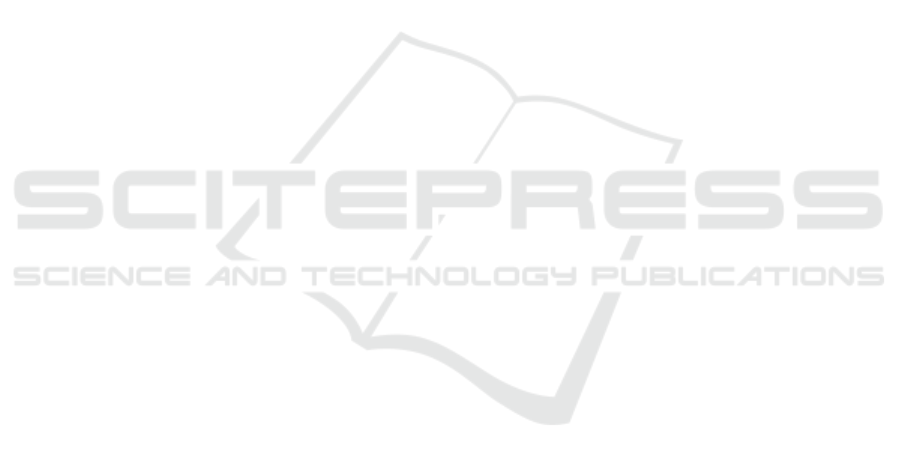
dedicated to the spatio-temporal modelling of long-
term activities in videos. We show that the model
can learn long-term dependencies across timesteps,
resulting in robust representations, and that it is not
possible to accurately classify long activities from a
few timesteps only. We give insights on the amount
of timesteps, their order and the importance of the
frame frequency. Next, a Region Attention module
supports spatio-temporal data to adaptively learn the
importance of the spatial cues in different video re-
gions, which also allow the backbone to learn rich
feature representations. Lastly, an Actor-Focus mech-
anism drives the attention on the truly discriminative
video regions where the actor is performing the ac-
tivity, neglecting background and irrelevant regions.
We demonstrate the effectiveness of the architecture,
benchmarking our model on the Breakfast Actions
Dataset, with a SOTA-matching accuracy of 89.84%.
Because of the modularity of our architecture and of
related work (Hussein et al., 2019a; Hussein et al.,
2019b; Hussein et al., 2020a), our framework could
complement other approaches. Due to the fact that the
strength of our model relies on the way the backbone
is fine-tuned and on the use of attention to account for
the spatial dimension, further modelling of the time
dimension could improve the results. Both PIC (Hus-
sein et al., 2020a) and Timeception (Hussein et al.,
2019a) successfully exploit the time axis and can be
juxtaposed on existing backbones, integrated with our
RA module. Experiments are left for future work. Fi-
nally, future work may include studies on the full I3D
fine-tuning and on a I3D + Region Attention end-to-
end training.
ACKNOWLEDGEMENTS
This work is supported by the Early Research Pro-
gram Hybrid AI, and by the research programme Per-
spectief EDL with project number P16-25 project 3,
which is financed by the Dutch Research Council
(NWO), Applied and Engineering Sciences (TTW).
REFERENCES
Burghouts, G. J. and Schutte, K. (2013). Spatio-temporal
layout of human actions for improved bag-of-words
action detection. In Pattern Recognition Letters.
Carreira, J. and Zisserman, A. (2017). Quo vadis, action
recognition? a new model and the kinetics dataset. In
CVPR.
Girdhar, R., Ramanan, D., Gupta, A., et al. (2017). Action-
vlad: Learning spatio-temporal aggregation for action
classification. In CVPR.
Herzig, R., Levi, E., Xu, H., et al. (2019). Spatio-temporal
action graph networks. In ICCV.
Hussein, N., Gavves, E., and Smeulders, A. W. M. (2019a).
Timeception for complex action recognition. In
CVPR.
Hussein, N., Gavves, E., and Smeulders, A. W. M. (2019b).
Videograph: Recognizing minutes-long human activ-
ities in videos. In arXiv.
Hussein, N., Gavves, E., and Smeulders, A. W. M. (2020a).
Pic: Permutation invariant convolution for recogniz-
ing long-range activities. In arXiv.
Hussein, N., Jain, M., and Bejnordi, B. E. (2020b).
Timegate: Conditional gating of segments in long-
range activities. In arXiv.
Kalfaoglu, M., Kalkan, S., and Alatan, A. (2020). Late tem-
poral modeling in 3d cnn architectures with bert for
action recognition. In arXiv.
Kuehne, H., Arslan, A., and Serre, T. (2014). The language
of actions: Recovering the syntax and semantics of
goal-directed human activities. In CVPR.
Qiu, Z., Yao, T., Ngo, C., et al. (2019). Learning spatio-
temporal representation with local and global diffu-
sion. In CVPR.
Schindler, K. and Gool, L. V. (2008). Action snippets: How
many frames does human action recognition require?
In IEEE Conference on Computer Vision and Pattern
Recognition.
Sigurdsson, G. A., Varol, G., Wang, X., et al. (2016). Hol-
lywood in homes: Crowdsourcing data collection for
activity understanding. In ECCV.
Varol, G., Laptev, I., and Schmid, C. (2017). Long-term
temporal convolutions for action recognition. In IEEE
Transactions on Pattern Analysis and Machine Intel-
ligence.
Wang, K., Peng, X., Yang, J., et al. (2020). Region atten-
tion networks for pose and occlusion robust facial ex-
pression recognition. In IEEE Transactions on Image
Processing.
Wang, X., Girshick, R., Gupta, A., et al. (2018). Non-local
neural networks. In CVPR.
Wang, X. and Gupta, A. (2018). Videos as space-time re-
gion graphs. In ECCV.
Wu, C. Y., Feichtenhofer, C., Fan, H., et al. (2019a). Long-
term feature banks for detailed video understanding.
In CVPR.
Wu, Y., Kirillov, A., Massa, F., et al. (2019b). Detectron2.
https://github.com/facebookresearch/detectron2.
Yang, J., Ren, P., Zhang, D., et al. (2017). Neural aggrega-
tion network for video face recognition. In CVPR.
Yeung, S., Russakovsky, O., Jin, N., et al. (2018). Every
moment counts: Dense detailed labeling of actions in
complex videos. In International Journal of Computer
Vision.
Zhou, B., Andonian, A., Oliva, A., et al. (2018). Temporal
relational reasoning in videos. In ECCV.
Long-term Behaviour Recognition in Videos with Actor-focused Region Attention
369