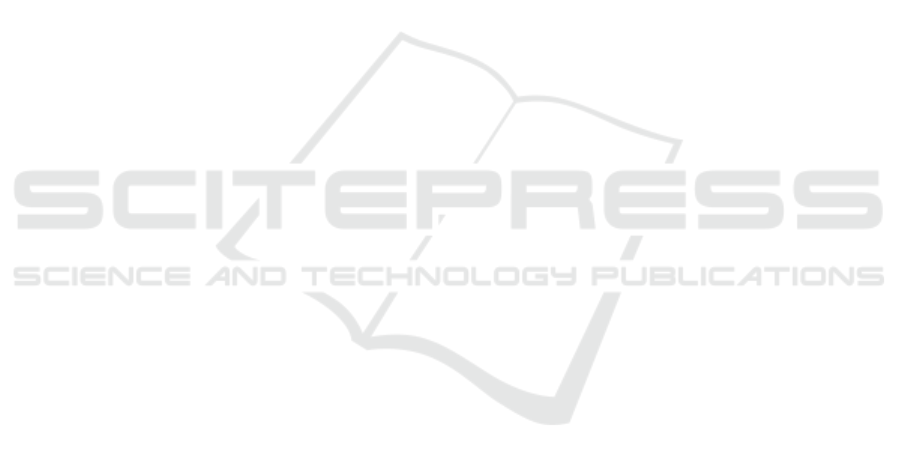
recent years and are often utilized for comparison pur-
poses.
The quantitative results obtained on the RoadLAB
dataset (Beauchemin et al., 2011) are presented in Ta-
ble 2. Our proposed model gives the maximum sim-
ilarity and minimum dissimilarity with respect to the
ground truth data. We conclude that our model pre-
dicts the driver’s eye fixation maps more accurately
than other saliency models.
6 CONCLUSIONS
We proposed convolution neural networks to predict
the potential saliency maps in the driving environ-
ment, and then employed our previous research re-
sults to estimate the probability of the driver gaze di-
rection, given head pose as a top-down factor. Fi-
nally, we statistically combined bottom-up and top-
down factors to obtain accurate drivers’ fixation pre-
dictions.
Our previous study established that driver gaze es-
timation is a crucial factor for driver maneuver predic-
tion. The identification of objects that drivers tend to
fixate on is of equal importance in maneuver predic-
tion models. We believe that the ability to estimate
these aspects of visual behaviour constitutes a signifi-
cant improvement for the prediction of maneuvers, as
drivers generally focus on environmental features a
few seconds before affecting one or more maneuvers.
REFERENCES
Beauchemin, S. S., Bauer, M. A., Kowsari, T., and Cho, J.
(2011). Portable and scalable vision-based vehicular
instrumentation for the analysis of driver intentional-
ity. IEEE Transactions on Instrumentation and Mea-
surement, 61(2):391–401.
Borji, A., Cheng, M.-M., Jiang, H., and Li, J. (2015).
Salient object detection: A benchmark. IEEE trans-
actions on image processing, 24(12):5706–5722.
Borji, A., Sihite, D. N., and Itti, L. (2012). Quantitative
analysis of human-model agreement in visual saliency
modeling: A comparative study. IEEE Transactions
on Image Processing, 22(1):55–69.
Cazzato, D., Leo, M., Distante, C., and Voos, H. (2020).
When i look into your eyes: A survey on computer
vision contributions for human gaze estimation and
tracking. Sensors, 20(13):3739.
Cornia, M., Baraldi, L., Serra, G., and Cucchiara, R. (2016).
A deep multi-level network for saliency prediction.
In 2016 23rd International Conference on Pattern
Recognition (ICPR), pages 3488–3493. IEEE.
Deng, T., Yan, H., and Li, Y.-J. (2017). Learning to boost
bottom-up fixation prediction in driving environments
via random forest. IEEE Transactions on Intelligent
Transportation Systems, 19(9):3059–3067.
Deng, T., Yan, H., Qin, L., Ngo, T., and Manjunath, B.
(2019). How do drivers allocate their potential at-
tention? driving fixation prediction via convolutional
neural networks. IEEE Transactions on Intelligent
Transportation Systems, 21(5):2146–2154.
Deng, T., Yang, K., Li, Y., and Yan, H. (2016). Where does
the driver look? top-down-based saliency detection in
a traffic driving environment. IEEE Transactions on
Intelligent Transportation Systems, 17(7):2051–2062.
Harel, J., Koch, C., and Perona, P. (2007). Graph-based vi-
sual saliency. In Advances in neural information pro-
cessing systems, pages 545–552.
Hou, X., Harel, J., and Koch, C. (2011). Image signa-
ture: Highlighting sparse salient regions. IEEE trans-
actions on pattern analysis and machine intelligence,
34(1):194–201.
Huang, X., Shen, C., Boix, X., and Zhao, Q. (2015). Sali-
con: Reducing the semantic gap in saliency prediction
by adapting deep neural networks. In Proceedings of
the IEEE International Conference on Computer Vi-
sion, pages 262–270.
Itti, L., Koch, C., and Niebur, E. (1998). A model of
saliency-based visual attention for rapid scene anal-
ysis. IEEE Transactions on pattern analysis and ma-
chine intelligence, 20(11):1254–1259.
Jain, A., Koppula, H. S., Raghavan, B., Soh, S., and Saxena,
A. (2015). Car that knows before you do: Anticipating
maneuvers via learning temporal driving models. In
Proceedings of the IEEE International Conference on
Computer Vision, pages 3182–3190.
Judd, T., Durand, F., and Torralba, A. (2012). A benchmark
of computational models of saliency to predict human
fixations.
Khairdoost, N., Shirpour, M., Bauer, M. A., and Beau-
chemin, S. S. (2020). Real-time maneuver prediction
using lstm. IEEE Transactions on Intelligent Vehicles.
Kowsari, T., Beauchemin, S. S., Bauer, M. A., Lauren-
deau, D., and Teasdale, N. (2014). Multi-depth cross-
calibration of remote eye gaze trackers and stereo-
scopic scene systems. In 2014 IEEE Intelligent
Vehicles Symposium Proceedings, pages 1245–1250.
IEEE.
K
¨
ummerer, M., Wallis, T. S., and Bethge, M. (2016).
Deepgaze ii: Reading fixations from deep fea-
tures trained on object recognition. arXiv preprint
arXiv:1610.01563.
Le Meur, O., Le Callet, P., and Barba, D. (2007). Predict-
ing visual fixations on video based on low-level visual
features. Vision research, 47(19):2483–2498.
Li, J., Levine, M. D., An, X., Xu, X., and He, H. (2012). Vi-
sual saliency based on scale-space analysis in the fre-
quency domain. IEEE transactions on pattern analy-
sis and machine intelligence, 35(4):996–1010.
Liu, N., Han, J., Zhang, D., Wen, S., and Liu, T. (2015). Pre-
dicting eye fixations using convolutional neural net-
works. In Proceedings of the IEEE Conference on
Computer Vision and Pattern Recognition, pages 362–
370.
VISAPP 2021 - 16th International Conference on Computer Vision Theory and Applications
74