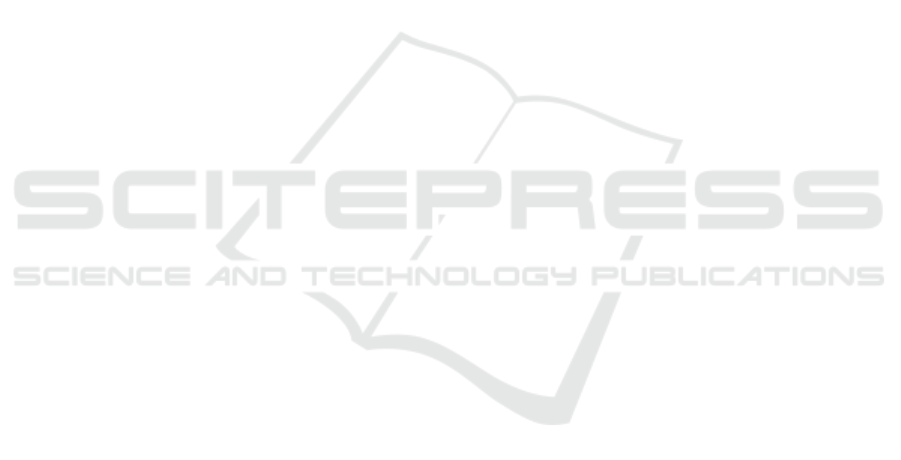
ference on Acoustics, Speech and Signal Processing
(ICASSP), pages 7610–7614.
Dubey, A. R., Shukla, N., and Kumar, D. (2020). Detec-
tion and classification of road signs using HOG-SVM
method. In Elc¸i, A., Sa, P. K., Modi, C. N., Olague,
G., Sahoo, M. N., and Bakshi, S., editors, Smart Com-
puting Paradigms: New Progresses and Challenges,
pages 49–56. Springer Singapore.
Eyben, F., W
¨
ollmer, M., and Schuller, B. (2010). opensmile
– the munich versatile and fast open-source audio fea-
ture extractor. In ACM Multimedia, pages 1459–1462.
Feng, W., Guan, N., Li, Y., Zhang, X., and Luo, Z. (2017).
Audio visual speech recognition with multimodal re-
current neural networks. In 2017 International Joint
Conference on Neural Networks (IJCNN), pages 681–
688. ISSN: 2161-4407.
Greco, F. and Polli, A. (2020). Emotional text mining: Cus-
tomer profiling in brand management. International
Journal of Information Management, 51:101934.
Hara, K., Kataoka, H., and Satoh, Y. (2017). Learning
spatio-temporal features with 3d residual networks for
action recognition. arXiv:1708.07632 [cs].
He, K., Zhang, X., Ren, S., and Sun, J. (2015). Deep resid-
ual learning for image recognition. arXiv:1512.03385
[cs].
Hershey, S., Chaudhuri, S., Ellis, D. P. W., Gemmeke,
J. F., Jansen, A., Moore, R. C., Plakal, M., Platt,
D., Saurous, R. A., Seybold, B., Slaney, M., Weiss,
R. J., and Wilson, K. (2017). CNN architectures
for large-scale audio classification. arXiv:1609.09430
[cs, stat].
Hochreiter, S. and Schmidhuber, J. (1997). Long short-term
memory. Neural computation, 9:1735–80.
Howard, A., Sandler, M., Chu, G., Chen, L.-C., Chen, B.,
Tan, M., Wang, W., Zhu, Y., Pang, R., Vasudevan, V.,
Le, Q. V., and Adam, H. (2019). Searching for mo-
bilenetv3.
Huddar, M. G., Sannakki, S. S., and Rajpurohit, V. S.
(2018). An ensemble approach to utterance level mul-
timodal sentiment analysis. In 2018 International
Conference on Computational Techniques, Electron-
ics and Mechanical Systems (CTEMS), pages 145–
150.
Kahou, S. E., Bouthillier, X., Lamblin, P., Gulcehre,
C., Michalski, V., Konda, K., Jean, S., Frou-
menty, P., Dauphin, Y., Boulanger-Lewandowski, N.,
Chandias Ferrari, R., Mirza, M., Warde-Farley, D.,
Courville, A., Vincent, P., Memisevic, R., Pal, C.,
and Bengio, Y. (2016). EmoNets: Multimodal deep
learning approaches for emotion recognition in video.
Journal on Multimodal User Interfaces, 10(2):99–
111.
Kingma, D. P. and Ba, J. (2017). Adam: A method for
stochastic optimization. arXiv:1412.6980 [cs].
Krizhevsky, A., Sutskever, I., and Hinton, G. E. (2012). Im-
ageNet classification with deep convolutional neural
networks. In Pereira, F., Burges, C. J. C., Bottou,
L., and Weinberger, K. Q., editors, Advances in Neu-
ral Information Processing Systems 25, pages 1097–
1105. Curran Associates, Inc.
Li, H., Sun, J., Xu, Z., and Chen, L. (2017). Multimodal
2d+3d facial expression recognition with deep fusion
convolutional neural network. IEEE Transactions on
Multimedia, 19(12):2816–2831. Conference Name:
IEEE Transactions on Multimedia.
Maas, A. L., Daly, R. E., Pham, P. T., Huang, D., Ng, A. Y.,
and Potts, C. (2011). Learning word vectors for sen-
timent analysis. In Proceedings of the 49th Annual
Meeting of the Association for Computational Lin-
guistics: Human Language Technologies, pages 142–
150, Portland, Oregon, USA. Association for Compu-
tational Linguistics.
Mao, J., Xu, W., Yang, Y., Wang, J., Huang, Z., and Yuille,
A. (2015). Deep captioning with multimodal recurrent
neural networks (m-RNN). arXiv:1412.6632 [cs].
Mikolov, T., Chen, K., Corrado, G., and Dean, J. (2013).
Efficient estimation of word representations in vector
space.
M
¨
antyl
¨
a, M. V., Graziotin, D., and Kuutila, M. (2018). The
evolution of sentiment analysis—a review of research
topics, venues, and top cited papers. Computer Sci-
ence Review, 27:16 – 32.
Morency, L.-P., Mihalcea, R., and Doshi, P. (2011). To-
wards Multimodal Sentiment Analysis: Harvesting
Opinions from The Web. In International Confer-
ence on Multimodal Interfaces (ICMI 2011), Alicante,
Spain.
Nandal, N., Tanwar, R., and Pruthi, J. (2020). Machine
learning based aspect level sentiment analysis for
amazon products. Spatial Information Research.
Pan, S. J. and Yang, Q. (2010). A survey on transfer learn-
ing. IEEE Transactions on Knowledge and Data En-
gineering, 22(10):1345–1359.
P
´
erez-Rosas, V., Mihalcea, R., and Morency, L.-P. (2013).
Utterance-level multimodal sentiment analysis. In
Proceedings of the 51st Annual Meeting of the Associ-
ation for Computational Linguistics (Volume 1: Long
Papers), pages 973–982, Sofia, Bulgaria. Association
for Computational Linguistics.
Poria, S., Cambria, E., Hazarika, D., Majumder, N., Zadeh,
A., and Morency, L.-P. (2017). Context-dependent
sentiment analysis in user-generated videos. In Pro-
ceedings of the 55th Annual Meeting of the Associa-
tion for Computational Linguistics (Volume 1: Long
Papers), pages 873–883. Association for Computa-
tional Linguistics.
Pradeep, K., Kamalavasan, K., Natheesan, R., and Pasqual,
A. (2018). Edgenet: Squeezenet like convolution neu-
ral network on embedded fpga. In 2018 25th IEEE
International Conference on Electronics, Circuits and
Systems (ICECS), pages 81–84.
Rawat, W. and Wang, Z. (2017). Deep convolutional neural
networks for image classification: A comprehensive
review. Neural Computation, 29:1–98.
Reshma, B. and Kiran, K. A. (2017). Active noise cancel-
lation for in-ear headphones implemented on fpga. In
2017 International Conference on Intelligent Comput-
ing and Control Systems (ICICCS), pages 602–606.
Schuller, B., Steidl, S., Batliner, A., Burkhardt, F., Dev-
illers, L., Muller, C., and Narayanan, S. S. (2010). The
Multimodal Neural Network for Sentiment Analysis in Embedded Systems
397