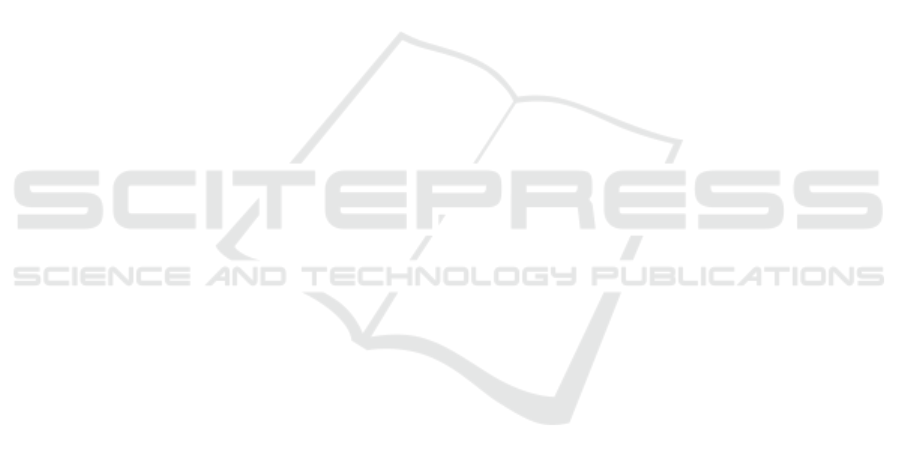
ternational Conference on Computer Vision (ICCV),
pages 1026–1034.
Jim
´
enez, J., Skalic, M., Martinez-Rosell, G., and De Fabri-
tiis, G. (2018). K deep: protein–ligand absolute bind-
ing affinity prediction via 3d-convolutional neural net-
works. Journal of chemical information and model-
ing, 58(2):287–296.
Kaggle, M. (2012). Kaggle: Merck molecular activity chal-
lenge. https://www.kaggle.com/c/MerckActivity, Ac-
cessed Feb 8, 2019.
Kingma, D. P. and Ba, J. (2014). Adam: A
method for stochastic optimization. cite
arxiv:1412.6980Comment: Published as a con-
ference paper at the 3rd International Conference for
Learning Representations, San Diego, 2015.
Landrum, G. (2020). Rdkit: Open-source cheminformatics.
Accessed April, 2020.
Leach, A. R., Gillet, V. J., Lewis, R. A., and Taylor, R.
(2010). Three-dimensional pharmacophore methods
in drug discovery. Journal of medicinal chemistry,
53(2):539–558.
LeCun, Y., Bengio, Y., and Hinton, G. (2015). Deep learn-
ing. nature, 521(7553):436–444.
Li, Y., Liu, Z., Li, J., Han, L., Liu, J., Zhao, Z., and Wang,
R. (2014). Comparative assessment of scoring func-
tions on an updated benchmark: 1. compilation of the
test set. Journal of chemical information and model-
ing, 54(6):1700–1716.
Liu, Z., Cui, Y., Xiong, Z., Nasiri, A., Zhang, A., and
Hu, J. (2019). Deepseqpan, a novel deep convolu-
tional neural network model for pan-specific class i
hla-peptide binding affinity prediction. Scientific re-
ports, 9(1):794.
Liu, Z., Su, M., Han, L., Liu, J., Yang, Q., Li, Y., and
Wang, R. (2017a). Forging the basis for developing
protein–ligand interaction scoring functions. Accounts
of chemical research, 50(2):302–309.
Liu, Z., Su, M., Han, L., Liu, J., Yang, Q., Li, Y., and
Wang, R. (2017b). Forging the basis for developing
protein–ligand interaction scoring functions. Accounts
of chemical research, 50(2):302–309.
Lundberg, S. M. and Lee, S.-I. (2017). A unified approach
to interpreting model predictions. In Advances in Neu-
ral Information Processing Systems 30, pages 4765–
4774. Curran Associates, Inc.
Nguyen, D. D. and Wei, G.-W. (2019a). Agl-score: Al-
gebraic graph learning score for protein–ligand bind-
ing scoring, ranking, docking, and screening. Journal
of chemical information and modeling, 59(7):3291–
3304.
Nguyen, D. D. and Wei, G.-W. (2019b). Dg-gl: Differen-
tial geometry-based geometric learning of molecular
datasets. International journal for numerical methods
in biomedical engineering, 35(3):e3179.
¨
Ozt
¨
urk, H.,
¨
Ozg
¨
ur, A., and Ozkirimli, E. (2018). Deepdta:
deep drug–target binding affinity prediction. Bioinfor-
matics, 34(17):i821–i829.
Paszke, A., Gross, S., Massa, F., Lerer, A., Bradbury, J.,
Chanan, G., Killeen, T., Lin, Z., Gimelshein, N.,
Antiga, L., Desmaison, A., Kopf, A., Yang, E., De-
Vito, Z., Raison, M., Tejani, A., Chilamkurthy, S.,
Steiner, B., Fang, L., Bai, J., and Chintala, S. (2019).
Pytorch: An imperative style, high-performance deep
learning library. In Advances in Neural Information
Processing Systems 32, pages 8024–8035. Curran As-
sociates, Inc.
P
´
erez-Sianes, J., P
´
erez-S
´
anchez, H., and D
´
ıaz, F. (2019).
Virtual screening meets deep learning. Current
computer-aided drug design, 15(1):6–28.
Ragoza, M., Hochuli, J., Idrobo, E., Sunseri, J., and Koes,
D. R. (2017). Protein–ligand scoring with convolu-
tional neural networks. Journal of chemical informa-
tion and modeling, 57(4):942–957.
Rifaioglu, A. S., Atas, H., Martin, M. J., Cetin-Atalay, R.,
Atalay, V., and Dogan, T. (2018). Recent applications
of deep learning and machine intelligence on in silico
drug discovery: methods, tools and databases. Brief.
Bioinform, 10.
Sieg, J., Flachsenberg, F., and Rarey, M. (2019). In need
of bias control: Evaluating chemical data for machine
learning in structure-based virtual screening. Jour-
nal of chemical information and modeling, 59(3):947–
961.
Stepniewska-Dziubinska, M. M., Zielenkiewicz, P., and
Siedlecki, P. (2017). Pafnucy–a deep neural network
for structure-based drug discovery. stat, 1050:19.
Su, M., Yang, Q., Du, Y., Feng, G., Liu, Z., Li, Y., and
Wang, R. (2018). Comparative assessment of scoring
functions: the casf-2016 update. Journal of chemical
information and modeling, 59(2):895–913.
Szegedy, C., Liu, W., Jia, Y., Sermanet, P., Reed, S.,
Anguelov, D., Erhan, D., Vanhoucke, V., and Rabi-
novich, A. (2015). Going deeper with convolutions.
In Proceedings of the IEEE conference on computer
vision and pattern recognition, pages 1–9.
Tompson, J., Goroshin, R., Jain, A., LeCun, Y., and Bregler,
C. (2015). Efficient object localization using convo-
lutional networks. In Proceedings of the IEEE Con-
ference on Computer Vision and Pattern Recognition,
pages 648–656.
Ulyanov, D., Vedaldi, A., and Lempitsky, V. (2017). Im-
proved texture networks: Maximizing quality and di-
versity in feed-forward stylization and texture synthe-
sis. In The IEEE Conference on Computer Vision and
Pattern Recognition (CVPR). IEEE.
W
´
ojcikowski, M., Kukiełka, M., Stepniewska-Dziubinska,
M. M., and Siedlecki, P. (2019). Development of
a protein–ligand extended connectivity (plec) finger-
print and its application for binding affinity predic-
tions. Bioinformatics, 35(8):1334–1341.
Zhang, H., Liao, L., Saravanan, K. M., Yin, P., and Wei,
Y. (2019). Deepbindrg: a deep learning based method
for estimating effective protein–ligand affinity. PeerJ,
7:e7362.
Zheng, L., Fan, J., and Mu, Y. (2019). Onionnet: a multiple-
layer intermolecular-contact-based convolutional neu-
ral network for protein–ligand binding affinity predic-
tion. ACS omega, 4(14):15956–15965.
LigityScore: Convolutional Neural Network for Binding-affinity Predictions
49