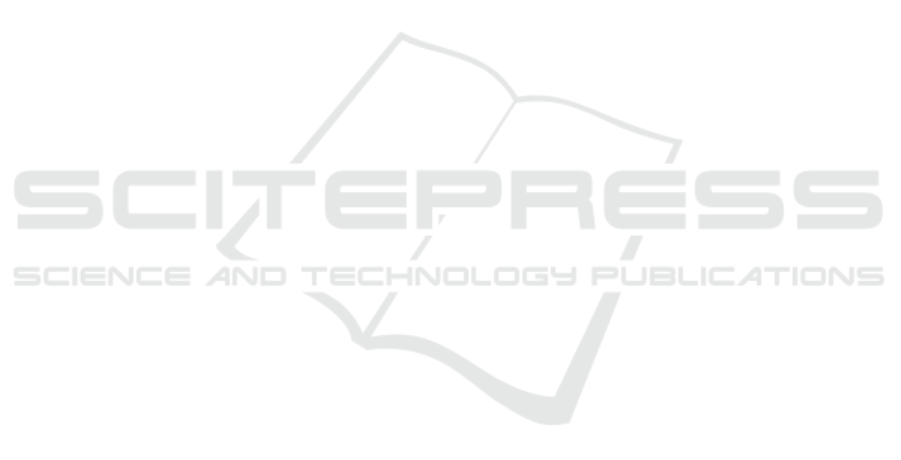
tle and mci. Computers in Biology and Medicine,
117:103592.
Jia, W., Muhammad, K., Wang, S.-H., and Zhang, Y.-
D. (2019). Five-category classification of patho-
logical brain images based on deep stacked sparse
autoencoder. Multimedia Tools and Applications,
78(4):4045–4064.
Kingma, D. P. and Welling, M. (2013). Auto-encoding vari-
ational bayes. arXiv preprint arXiv:1312.6114.
Litjens, G., Kooi, T., Bejnordi, B. E., Setio, A. A. A.,
Ciompi, F., Ghafoorian, M., Van Der Laak, J. A.,
Van Ginneken, B., and S
´
anchez, C. I. (2017). A survey
on deep learning in medical image analysis. Medical
image analysis, 42:60–88.
Liu, M., Zhang, J., Lian, C., and Shen, D. (2019). Weakly
supervised deep learning for brain disease prognosis
using mri and incomplete clinical scores. IEEE Trans-
actions on Cybernetics.
Ma, J. and Plonka, G. (2010). A review of curvelets and re-
cent applications. IEEE Signal Processing Magazine,
27(2):118–133.
Makhzani, A. and Frey, B. (2013). K-sparse autoencoders.
arXiv preprint arXiv:1312.5663.
Masci, J., Meier, U., Cires¸an, D., and Schmidhuber, J.
(2011). Stacked convolutional auto-encoders for hi-
erarchical feature extraction. In International con-
ference on artificial neural networks, pages 52–59.
Springer.
Nayak, D. R., Dash, R., Majhi, B., and Prasad, V. (2017).
Automated pathological brain detection system: A
fast discrete curvelet transform and probabilistic neu-
ral network based approach. Expert Systems with Ap-
plications, 88:152–164.
Nozadi, S. H., Kadoury, S., Initiative, A. D. N., et al.
(2018). Classification of alzheimer’s and mci patients
from semantically parcelled pet images: a compari-
son between av45 and fdg-pet. International journal
of biomedical imaging, 2018.
Payan, A. and Montana, G. (2015). Predicting alzheimer’s
disease: a neuroimaging study with 3d convolutional
neural networks. arXiv preprint arXiv:1502.02506.
Pinaya, W. H., Gadelha, A., Doyle, O. M., Noto, C., Zug-
man, A., Cordeiro, Q., Jackowski, A. P., Bressan,
R. A., and Sato, J. R. (2016). Using deep belief net-
work modelling to characterize differences in brain
morphometry in schizophrenia. Scientific reports,
6:38897.
Ramzan, F., Khan, M. U. G., Rehmat, A., Iqbal, S., Saba,
T., Rehman, A., and Mehmood, Z. (2020). A deep
learning approach for automated diagnosis and multi-
class classification of alzheimer’s disease stages using
resting-state fmri and residual neural networks. Jour-
nal of Medical Systems, 44(2):37.
Rifai, S., Mesnil, G., Vincent, P., Muller, X., Bengio, Y.,
Dauphin, Y., and Glorot, X. (2011). Higher order
contractive auto-encoder. In Joint European confer-
ence on machine learning and knowledge discovery
in databases, pages 645–660. Springer.
Ryu, C., Jang, D. C., Jung, D., Kim, Y. G., Shim, H. G.,
Ryu, H.-H., Lee, Y.-S., Linden, D. J., Worley, P. F.,
and Kim, S. J. (2017). Stim1 regulates somatic
ca2+ signals and intrinsic firing properties of cere-
bellar purkinje neurons. Journal of Neuroscience,
37(37):8876–8894.
Scheltens, P. and Korf, E. S. (2000). Contribution of
neuroimaging in the diagnosis of alzheimer’s disease
and other dementias. Current opinion in neurology,
13(4):391–396.
Shen, D., Wu, G., and Suk, H.-I. (2017). Deep learning in
medical image analysis. Annual review of biomedical
engineering, 19:221–248.
Silverman, D. (2009). PET in the Evaluation of Alzheimer’s
Disease and Related Disorders. Springer.
Suk, H.-I., Lee, S.-W., Shen, D., Initiative, A. D. N., et al.
(2017). Deep ensemble learning of sparse regression
models for brain disease diagnosis. Medical image
analysis, 37:101–113.
Swain, B. K., Sahani, M., and Sharma, R. (2020). Auto-
matic recognition of the early stage of alzheimer’s dis-
ease based on discrete wavelet transform and reduced
deep convolutional neural network. In Innovation in
Electrical Power Engineering, Communication, and
Computing Technology, pages 531–542. Springer.
Vincent, P., Larochelle, H., Bengio, Y., and Manzagol, P.-
A. (2008). Extracting and composing robust features
with denoising autoencoders. In Proceedings of the
25th international conference on Machine learning,
pages 1096–1103.
Wee, C.-Y., Liu, C., Lee, A., Poh, J. S., Ji, H., Qiu, A.,
Initiative, A. D. N., et al. (2019). Cortical graph
neural network for ad and mci diagnosis and transfer
learning across populations. NeuroImage: Clinical,
23:101929.
Zhang, L. and Suganthan, P. N. (2016). A comprehensive
evaluation of random vector functional link networks.
Information sciences, 367:1094–1105.
VISAPP 2021 - 16th International Conference on Computer Vision Theory and Applications
292