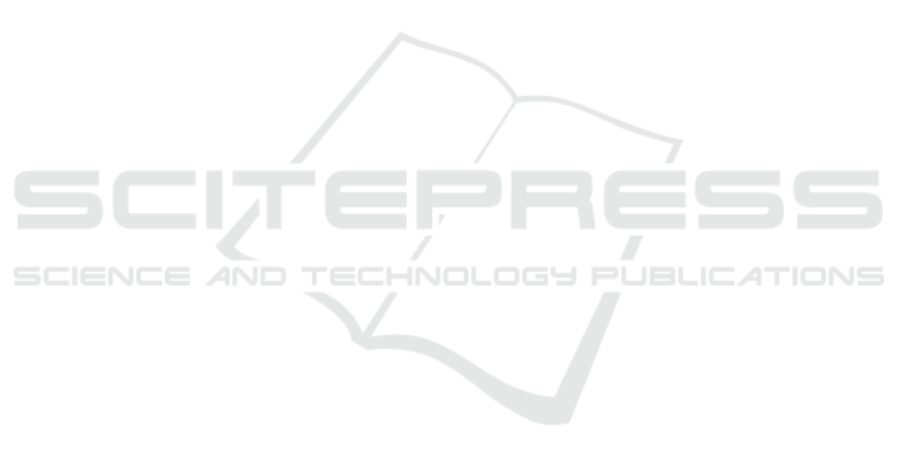
Course Innovation Grant from Moxie Foundation,
and the Intelligence Community Center of Academic
Excellence (IC CAE) at Rutgers University (Awards
#HHM402-19-1-0003 and #HHM402-18-1-0007).
REFERENCES
Achlioptas, P., Diamanti, O., Mitliagkas, I., and Guibas, L.
(2018). Learning representations and generative mod-
els for 3d point clouds. In International conference on
machine learning, pages 40–49. PMLR.
Apple Inc. (2020). iPad Pro. https://www.apple.com/
ipad-pro/specs/.
Bhargava, P., Kim, T., Poulton, C. V., Notaros, J., Yaa-
cobi, A., Timurdogan, E., Baiocco, C., Fahrenkopf,
N., Kruger, S., Ngai, T., Timalsina, Y., Watts, M. R.,
and Stojanovi
´
c, V. (2019). Fully integrated coherent
lidar in 3d-integrated silicon photonics/65nm cmos.
In 2019 Symposium on VLSI Circuits, pages C262–
C263.
Caron, M., Bojanowski, P., Joulin, A., and Douze, M.
(2018). Deep clustering for unsupervised learning of
visual features. In Proceedings of the ECCV, pages
132–149.
Chang, A. X., Funkhouser, T., Guibas, L., Hanrahan, P.,
Huang, Q., Li, Z., Savarese, S., Savva, M., Song, S.,
Su, H., Xiao, J., Yi, L., and Yu, F. (2015). Shapenet:
An information-rich 3d model repository.
Gong, J. (2013). Mobile lidar data collection and analy-
sis for post-sandy disaster recovery. In Computing in
Civil Engineering (2013), pages 677–684.
Gong, J., Zhou, H., Gordon, C., and Jalayer, M. (2012).
Mobile terrestrial laser scanning for highway inven-
tory data collection. In Computing in Civil Engineer-
ing (2012), pages 545–552.
Hackel, T., Savinov, N., Ladicky, L., Wegner, J. D.,
Schindler, K., and Pollefeys, M. (2017). SEMAN-
TIC3D.NET: A new large-scale point cloud classifica-
tion benchmark. In ISPRS Annals of the Photogram-
metry, Remote Sensing and Spatial Information Sci-
ences, volume IV-1-W1, pages 91–98.
Hu, X. and Gong, J. (2018). Advancing smart and re-
silient cities with big spatial disaster data: Challenges,
progress, and opportunities. In Data Analytics for
Smart Cities, pages 53–90. Auerbach Publications.
Huang, J. and You, S. (2016). Point cloud labeling using
3d convolutional neural network. In 2016 23rd Inter-
national Conference on Pattern Recognition (ICPR),
pages 2670–2675. IEEE.
Jing, L., Yang, X., Liu, J., and Tian, Y. (2018). Self-
supervised spatiotemporal feature learning via video
rotation prediction. arXiv preprint arXiv:1811.11387.
Kanezaki, A., Matsushita, Y., and Nishida, Y. (2018). Ro-
tationnet: Joint object categorization and pose estima-
tion using multiviews from unsupervised viewpoints.
In Proceedings of the IEEE CVPR, pages 5010–5019.
Maturana, D. and Scherer, S. (2015). Voxnet: A 3d con-
volutional neural network for real-time object recog-
nition. In 2015 IEEE/RSJ International Conference
on Intelligent Robots and Systems (IROS), pages 922–
928. IEEE.
Munoz, D., Bagnell, J. A. D., Vandapel, N., and Hebert, M.
(2009). Contextual classification with functional max-
margin markov networks. In Proceedings of IEEE
CVPR.
Noroozi, M. and Favaro, P. (2016). Unsupervised learn-
ing of visual representations by solving jigsaw puz-
zles. Lecture Notes in Computer Science, page 69–84.
Novotny, D., Larlus, D., and Vedaldi, A. (2017). Learning
3d object categories by looking around them. 2017
IEEE International Conference on Computer Vision
(ICCV).
Qi, C. R., Su, H., Mo, K., and Guibas, L. J. (2017a). Point-
net: Deep learning on point sets for 3d classification
and segmentation. In Proceedings of the IEEE con-
ference on computer vision and pattern recognition,
pages 652–660.
Qi, C. R., Su, H., Nießner, M., Dai, A., Yan, M., and
Guibas, L. J. (2016). Volumetric and multi-view cnns
for object classification on 3d data. In Proceedings of
the IEEE CVPR, pages 5648–5656.
Qi, C. R., Yi, L., Su, H., and Guibas, L. J. (2017b). Point-
net++: Deep hierarchical feature learning on point sets
in a metric space. In Advances in neural information
processing systems, pages 5099–5108.
Sauder, J. and Sievers, B. (2019). Context prediction for
unsupervised deep learning on point clouds. arXiv
preprint arXiv:1901.08396.
Su, H., Maji, S., Kalogerakis, E., and Learned-Miller, E. G.
(2015). Multi-view convolutional neural networks for
3d shape recognition. In Proc. ICCV.
Wang, Y., Sun, Y., Liu, Z., Sarma, S. E., Bronstein, M. M.,
and Solomon, J. M. (2019). Dynamic graph cnn
for learning on point clouds. ACM Transactions on
Graphics (TOG), 38(5):1–12.
Wu, Z., Song, S., Khosla, A., Yu, F., Zhang, L., Tang, X.,
and Xiao, J. (2015a). 3d shapenets: A deep represen-
tation for volumetric shapes. In Proceedings of the
IEEE CVPR, pages 1912–1920.
Wu, Z., Song, S., Khosla, A., Yu, F., Zhang, L., Tang, X.,
and Xiao, J. (2015b). 3d shapenets: A deep repre-
sentation for volumetric shapes. In Proceedings of
the IEEE conference on computer vision and pattern
recognition, pages 1912–1920.
Xu, K., Yao, Y., Murasaki, K., Ando, S., and Sagata, A.
(2019). Semantic segmentation of sparsely annotated
3d point clouds by pseudo-labelling. In 2019 Inter-
national Conference on 3D Vision (3DV), pages 463–
471.
Xu, X. and Lee, G. H. (2020). Weakly supervised semantic
point cloud segmentation: Towards 10x fewer labels.
In Proceedings of the IEEE/CVF CVPR, pages 13706–
13715.
Yang, Y., Feng, C., Shen, Y., and Tian, D. (2018). Fold-
ingnet: Point cloud auto-encoder via deep grid de-
formation. In Proceedings of the IEEE CVPR, pages
206–215.
A Snapshot-based Approach for Self-supervised Feature Learning and Weakly-supervised Classification on Point Cloud Data
407