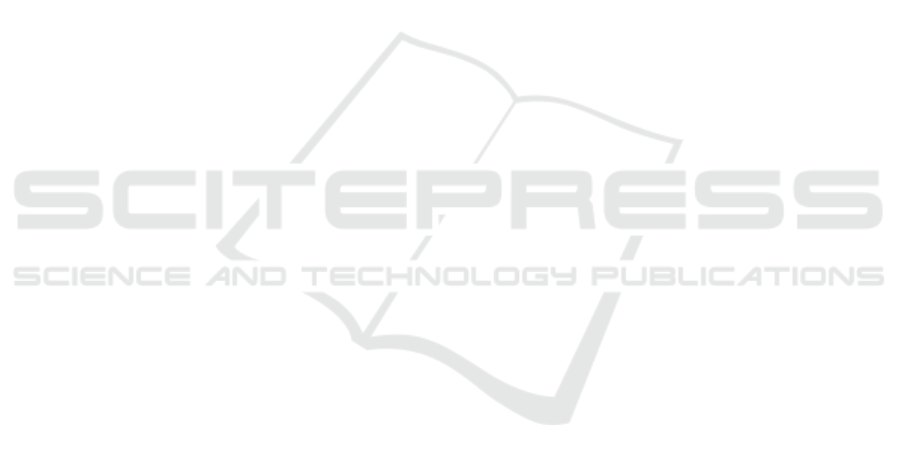
ACKNOWLEDGEMENTS
This work has received funding from the Clean Sky
2 Joint Undertaking under the European Union’s
Horizon 2020 research and innovation program
under grant agreement No. 865162, SmaCS
(https://www.smacs.eu/).
REFERENCES
ASAM (2020). OpenLABEL. https://www.asam.net/
project-detail/scenario-storage-and-labelling/.
Bengio, Y. and CA, M. (2015). Rmsprop and equilibrated
adaptive learning rates for nonconvex optimization.
corr abs/1502.04390.
Bourke, P. and Felinto, D. (2010). Blender and immer-
sive gaming in a hemispherical dome. In International
Conference on Computer Games, Multimedia and Al-
lied Technology, volume 1, pages 280–284.
Deng, J., Dong, W., Socher, R., Li, L.-J., Li, K., and Fei-
Fei, L. (2009). Imagenet: A large-scale hierarchical
image database. In 2009 IEEE conference on com-
puter vision and pattern recognition, pages 248–255.
Ieee.
Hernandez-Leal, P., Kartal, B., and Taylor, M. (2019). A
survey and critique of multiagent deep reinforcement
learning. Autonomous Agents and Multi-Agent Sys-
tems, 33:750–797.
Hou, Q., Cheng, M., Hu, X., Borji, A., Tu, Z., and Torr,
P. H. S. (2019). Deeply supervised salient object
detection with short connections. IEEE Transac-
tions on Pattern Analysis and Machine Intelligence,
41(4):815–828.
Hurl, B., Czarnecki, K., and Waslander, S. L. (2019).
Precise synthetic image and LiDAR (PreSIL) dataset
for autonomous vehicle perception. arXiv preprint
arXiv:1905.00160.
Khan, S., Phan, B., Salay, R., and Czarnecki, K. (2019).
ProcSy: Procedural synthetic dataset generation to-
wards influence factor studies of semantic segmenta-
tion networks. In Proceedings of the IEEE/CVF Con-
ference on Computer Vision and Pattern Recognition
(CVPR) Workshops, pages 88–96.
Lai, K.-T., Lin, C.-C., Kang, C.-Y., Liao, M.-E., and Chen,
M.-S. (2018). VIVID: Virtual environment for visual
deep learning. In Proceedings of the ACM Interna-
tional Conference on Multimedia (MM), pages 1356–
1359.
Loper, M., Mahmood, N., Romero, J., Pons-Moll, G., and
Black, M. J. (2015). SMPL: A skinned multi-person
linear model. ACM Transactions on Graphics (Pro-
ceedings of SIGGRAPH Asia), 34(6):248:1–248:16.
Maninis, K. K., Caelles, S., Chen, Y., Pont-Tuset, J., Leal-
Taix
´
e, L., Cremers, D., and Van Gool, L. (2019).
Video object segmentation without temporal informa-
tion. IEEE Transactions on Pattern Analysis and Ma-
chine Intelligence, 41(6):1515–1530.
Mujika, A., Fanlo, A. D., Tamayo, I., Senderos, O., Baran-
diaran, J., Aranjuelo, N., Nieto, M., and Otaegui, O.
(2019). Web-based video-assisted point cloud anno-
tation for ADAS validation. In Proceedings of the In-
ternational Conference on 3D Web Technology, pages
1–9.
Nikolenko, S. I. (2019). Synthetic data for deep learning.
arXiv preprint arXiv:1909.11512.
Rajpura, P. S., Bojinov, H., and Hegde, R. S. (2017). Ob-
ject detection using deep CNNs trained on synthetic
images. arXiv preprint arXiv:1706.06782.
Saleh, F. S., Aliakbarian, M. S., Salzmann, M., Peters-
son, L., and Alvarez, J. M. (2018). Effective use of
synthetic data for urban scene semantic segmentation.
arXiv preprint arXiv:1807.06132.
Scheck, T., Seidel, R., and Hirtz, G. (2020). Learning from
theodore: A synthetic omnidirectional top-view in-
door dataset for deep transfer learning. In Proceed-
ings of the IEEE Winter Conference on Applications
of Computer Vision (WACV), pages 932–941.
Seib, V., Lange, B., and Wirtz, S. (2020). Mixing real
and synthetic data to enhance neural network train-
ing – a review of current approaches. arXiv preprint
arXiv:2007.08781.
Shah, S., Dey, D., Lovett, C., and Kapoor, A. (2017). Air-
Sim: High-fidelity visual and physical simulation for
autonomous vehicles. Field and Service Robotics,
pages 621–635.
Shorten, C. and Khoshgoftaar, T. (2019). A survey on image
data augmentation for deep learning. Journal of Big
Data, 6:1–48.
Singh, R., Vatsa, M., Patel, V. M., and Ratha, N., editors
(2020). Domain Adaptation for Visual Understanding.
Springer, Cham.
Tan, M. and Le, Q. V. (2019). Efficientnet: Rethink-
ing model scaling for convolutional neural networks.
arXiv preprint arXiv:1905.11946.
Vicomtech (2020). VCD - video content description. https:
//vcd.vicomtech.org/.
Xi, Y., Zhang, Y., Ding, S., and Wan, S. (2020). Visual ques-
tion answering model based on visual relationship de-
tection. Signal Processing: Image Communication,
80:115648.
Zhang, P., Lan, C., Xing, J., Zeng, W., Xue, J., and Zheng,
N. (2019). View adaptive neural networks for high
performance skeleton-based human action recogni-
tion. IEEE Transactions on Pattern Analysis and Ma-
chine Intelligence, 41(8):1963–1978.
Building Synthetic Simulated Environments for Configuring and Training Multi-camera Systems for Surveillance Applications
91