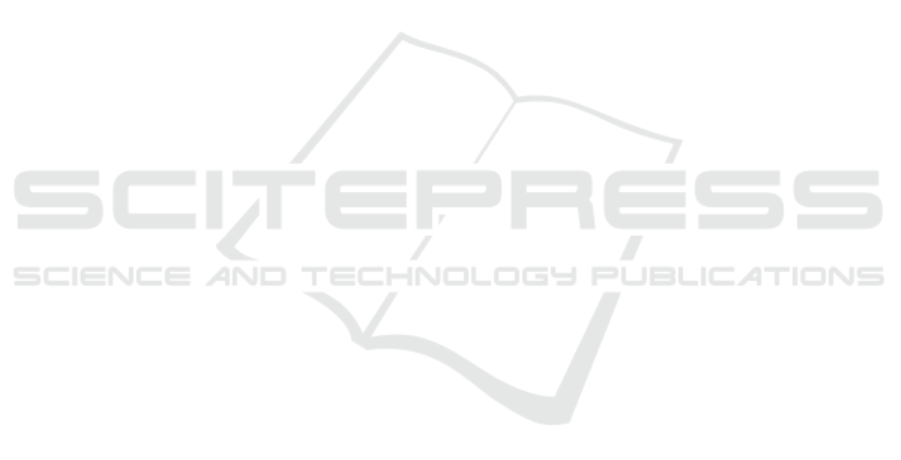
discriminative time warping. 2019 IEEE/CVF Con-
ference on Computer Vision and Pattern Recognition
(CVPR), pages 12418–12427.
Maghoumi, M. and LaViola, J. J. (2018). Deepgru: Deep
gesture recognition utility. In ISVC.
Moon, G., Chang, J., and Lee, K. M. (2018). V2v-
posenet: Voxel-to-voxel prediction network for accu-
rate 3d hand and human pose estimation from a single
depth map. In The IEEE Conference on Computer Vi-
sion and Pattern Recognition (CVPR).
Nguyen, X. S., Brun, L., L
´
ezoray, O., and Bougleux, S.
(2019). A neural network based on spd manifold
learning for skeleton-based hand gesture recognition.
2019 IEEE/CVF Conference on Computer Vision and
Pattern Recognition (CVPR), pages 12028–12037.
Ohn-Bar, E. and Trivedi, M. M. (2013). Joint angles sim-
ilarities and hog2 for action recognition. 2013 IEEE
Conference on Computer Vision and Pattern Recogni-
tion Workshops.
Ohn-Bar, E. and Trivedi, M. M. (2014). Hand gesture recog-
nition in real time for automotive interfaces: A multi-
modal vision-based approach and evaluations. IEEE
Transactions on Intelligent Transportation Systems,
15:2368–2377.
Oreifej, O. and Liu, Z. (2013). Hon4d: Histogram of ori-
ented 4d normals for activity recognition from depth
sequences. 2013 IEEE Conference on Computer Vi-
sion and Pattern Recognition, pages 716–723.
Rahmani, H. and Mian, A. S. (2016). 3d action recogni-
tion from novel viewpoints. 2016 IEEE Conference
on Computer Vision and Pattern Recognition (CVPR),
pages 1506–1515.
Ramirez-Amaro, K., Beetz, M., and Cheng, G. (2017).
Transferring skills to humanoid robots by extracting
semantic representations from observations of human
activities. Artif. Intell., 247:95–118.
Rastgoo, R., Kiani, K., and Escalera, S. (2020). Hand sign
language recognition using multi-view hand skeleton.
Shi, L., Zhang, Y., Cheng, J., and Lu, H. (2018). Non-
local graph convolutional networks for skeleton-based
action recognition.
Si, C., Chen, W., Wang, W., Wang, L., and Tan, T. (2019).
An attention enhanced graph convolutional lstm net-
work for skeleton-based action recognition. In CVPR.
Smedt, Q. D., Wannous, H., and Vandeborre, J.-P. (2016).
Skeleton-based dynamic hand gesture recognition.
2016 IEEE Conference on Computer Vision and Pat-
tern Recognition Workshops (CVPRW), pages 1206–
1214.
Sridhar, S., Feit, A. M., Theobalt, C., and Oulasvirta, A.
(2015). Investigating the dexterity of multi-finger in-
put for mid-air text entry. In CHI ’15.
Surie, D., Pederson, T., Lagriffoul, F., Janlert, L.-E., and
Sj
¨
olie, D. (2007). Activity recognition using an ego-
centric perspective of everyday objects. In UIC.
Tang, Y., Tian, Y., Lu, J., Li, P., and Zhou, J. (2018).
Deep progressive reinforcement learning for skeleton-
based action recognition. 2018 IEEE/CVF Conference
on Computer Vision and Pattern Recognition, pages
5323–5332.
Tekin, B., Bogo, F., and Pollefeys, M. (2019). H+o: Unified
egocentric recognition of 3d hand-object poses and in-
teractions. 2019 IEEE/CVF Conference on Computer
Vision and Pattern Recognition (CVPR), pages 4506–
4515.
Vemulapalli, R., Arrate, F., and Chellappa, R. (2014). Hu-
man action recognition by representing 3d skeletons
as points in a lie group. 2014 IEEE Conference on
Computer Vision and Pattern Recognition, pages 588–
595.
Wang, H. and Wang, L. (2017). Modeling temporal dynam-
ics and spatial configurations of actions using two-
stream recurrent neural networks. 2017 IEEE Con-
ference on Computer Vision and Pattern Recognition
(CVPR), pages 3633–3642.
Wang, L., Huynh, D. Q., and Koniusz, P. (2019). A compar-
ative review of recent kinect-based action recognition
algorithms. IEEE Transactions on Image Processing,
29:15–28.
Yan, S., Xiong, Y., and Lin, D. (2018). Spatial temporal
graph convolutional networks for skeleton-based ac-
tion recognition. In AAAI.
Ying, X. (2019). An overview of overfitting and its solu-
tions.
Yuan, S., Garcia-Hernando, G., Stenger, B., Moon, G.,
Yong Chang, J., Mu Lee, K., Molchanov, P., Kautz,
J., Honari, S., Ge, L., Yuan, J., Chen, X., Wang, G.,
Yang, F., Akiyama, K., Wu, Y., Wan, Q., Madadi, M.,
Escalera, S., Li, S., Lee, D., Oikonomidis, I., Argy-
ros, A., and Kim, T.-K. (2018). Depth-based 3d hand
pose estimation: From current achievements to future
goals. In The IEEE Conference on Computer Vision
and Pattern Recognition (CVPR).
Zanfir, M., Leordeanu, M., and Sminchisescu, C. (2013).
The moving pose: An efficient 3d kinematics descrip-
tor for low-latency action recognition and detection.
2013 IEEE International Conference on Computer Vi-
sion, pages 2752–2759.
Zhang, C., Yang, X., and Tian, Y. (2013). Histogram of
3d facets: A characteristic descriptor for hand gesture
recognition. 2013 10th IEEE International Confer-
ence and Workshops on Automatic Face and Gesture
Recognition (FG), pages 1–8.
Zhang, X., Qin, S., Xu, Y., and Xu, H. (2019). Quaternion
product units for deep learning on 3d rotation groups.
ArXiv, abs/1912.07791.
Zhang, X., Wang, Y., Gou, M., Sznaier, M., and Camps, O.
(2016). Efficient temporal sequence comparison and
classification using gram matrix embeddings on a rie-
mannian manifold. 2016 IEEE Conference on Com-
puter Vision and Pattern Recognition (CVPR).
VISAPP 2021 - 16th International Conference on Computer Vision Theory and Applications
302