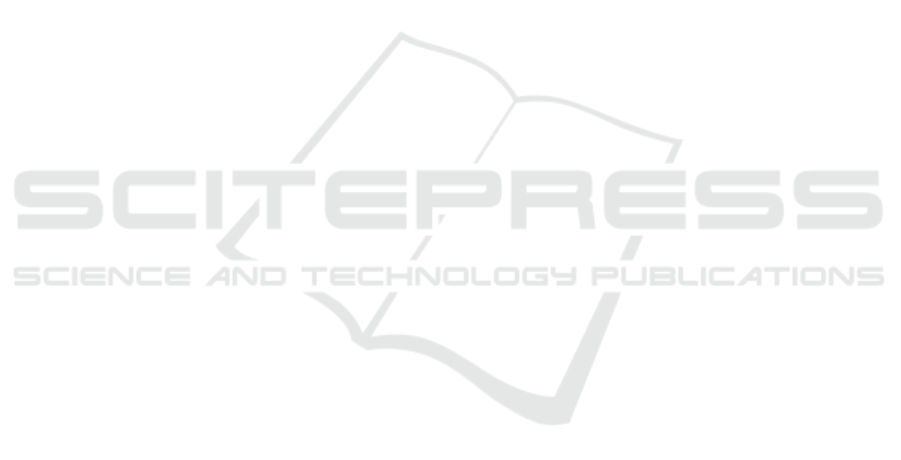
den, pathophysiology, diagnosis, and therapeutic ap-
proaches. Dermatology and therapy, 7(1):5–19.
B
¨
orve, A., Gyllencreutz, J., Terstappen, K., Backman,
E., Aldenbratt, A., Danielsson, M., Gillstedt, M.,
Sandberg, C., and Paoli, J. (2014). Smartphone
teledermoscopy referrals: A novel process for im-
proved triage of skin cancer patients. Acta dermato-
venereologica, 95.
Commissioning, P. C. (2011). Quality standards
for teledermatology using ’store and forward’
images. https://www.bad.org.uk/shared/get-
file.ashx?itemtype=document&id=794. Last accessed
September, 24th, 2020.
Dahl
´
en Gyllencreutz, J., Johansson Backman, E., Terstap-
pen, K., and Paoli, J. (2018). Teledermoscopy images
acquired in primary health care and hospital settings -
a comparative study of image quality. Journal of the
European Academy of Dermatology and Venereology,
32(6):1038–1043.
de Carvalho, T. M., Noels, E., Wakkee, M., Udrea, A., and
Nijsten, T. (2019). Development of smartphone apps
for skin cancer risk assessment: progress and promise.
JMIR Dermatology, 2(1):e13376.
Dugonik, B., Dugonik, A., Marovt, M., and Golob, M.
(2020). Image quality assessment of digital image
capturing devices for melanoma detection. Applied
Sciences (Switzerland), 10(8):2876.
Errichetti, E. and Stinco, G. (2016). Dermoscopy in general
dermatology: a practical overview. Dermatology and
therapy, 6(4):471–507.
EuroMelanoma (2020). https://www.euromelanoma.org/intl.
Last accessed September, 24th, 2020.
Faria, J., Almeida, J., Vasconcelos, M. J. M., and Rosado,
L. (2019). Automated mobile image acquisition of
skin wounds using real-time deep neural networks. In
Annual Conference on Medical Image Understanding
and Analysis, pages 61–73. Springer.
Ferlay, J., Colombet, M., Soerjomataram, I., Mathers, C.,
Parkin, D., Pi
˜
neros, M., Znaor, A., and Bray, F.
(2019). Estimating the global cancer incidence and
mortality in 2018: Globocan sources and methods. In-
ternational journal of cancer, 144(8):1941–1953.
Fernandes, K., Cruz, R., and Cardoso, J. S. (2018). Deep
image segmentation by quality inference. In 2018
International Joint Conference on Neural Networks
(IJCNN), pages 1–8. IEEE.
Feurer, M., Klein, A., Eggensperger, K., Springenberg, J.,
Blum, M., and Hutter, F. (2015). Efficient and robust
automated machine learning. In Advances in neural
information processing systems, pages 2962–2970.
Finnane, A., Curiel-Lewandrowski, C., Wimberley, G., Caf-
fery, L., Katragadda, C., Halpern, A., Marghoob,
A. A., Malvehy, J., Kittler, H., Hofmann-Wellenhof,
R., Abraham, I., and Soyer, H. P. (2017a). Proposed
technical guidelines for the acquisition of clinical im-
ages of skin-related conditions. JAMA Dermatology,
153(5):453–457.
Finnane, A., Dallest, K., Janda, M., and Soyer, H. P.
(2017b). Teledermatology for the diagnosis and man-
agement of skin cancer: a systematic review. JAMA
dermatology, 153(3):319–327.
Flores, E. and Scharcanski, J. (2016). Segmentation
of melanocytic skin lesions using feature learning
and dictionaries. Expert Systems with Applications,
56:300–309.
Gonc¸alves, J., Conceic¸ao, T., and Soares, F. (2019). Inter-
observer reliability in computer-aided diagnosis of di-
abetic retinopathy. In HEALTHINF, pages 481–491.
Ltd, E. I. (2019). Dermofit image library - edinburgh in-
novations. https://licensing.eri.ed.ac.uk/i/
software/dermofit-image-library.html. Last
accessed 11 June 2019.
Lubax, I. (2019). Dermpic. (mobile software).
Munteanu, C. (2016). Spotmole. (mobile software).
Oliveira, R. B., Marranghello, N., Pereira, A. S., and
Tavares, J. M. R. (2016). A computational approach
for detecting pigmented skin lesions in macroscopic
images. Expert Systems with Applications, 61:53–63.
Pertuz, S., Puig, D., and Garcia, M. A. (2013). Analysis of
focus measure operators for shape-from-focus. Pat-
tern Recognition, 46(5):1415–1432.
Rat, C., Hild, S., S
´
erandour, J. R., Gaultier, A., Quereux,
G., Dreno, B., and Nguyen, J.-M. (2018). Use of
smartphones for early detection of melanoma: sys-
tematic review. Journal of medical Internet research,
20(4):e135.
Rosado, L., Da Costa, J. M. C., Elias, D., and Cardoso, J. S.
(2017). Mobile-based analysis of malaria-infected
thin blood smears: automated species and life cycle
stage determination. Sensors, 17(10):2167.
Rosado, L. and Vasconcelos, M. (2015). Automatic seg-
mentation methodology for dermatological images ac-
quired via mobile devices. In Proceedings of the Inter-
national Joint Conference on Biomedical Engineering
Systems and Technologies-Volume 5, pages 246–251.
SCITEPRESS-Science and Technology Publications,
Lda.
Santos, A., Ortiz de Sol
´
orzano, C., Vaquero, J. J., Pena,
J. M., Malpica, N., and del Pozo, F. (1997). Evalu-
ation of autofocus functions in molecular cytogenetic
analysis. Journal of microscopy, 188(3):264–272.
Udrea, A. and Lupu, C. (2014). Real-time acquisition of
quality verified nonstandardized color images for skin
lesions risk assessment - A preliminary study. In
2014 18th International Conference on System The-
ory, Control and Computing, ICSTCC 2014, pages
199–204. Institute of Electrical and Electronics Engi-
neers Inc.
Vasconcelos, M. J. M. and Rosado, L. (2014). No-
reference blur assessment of dermatological images
acquired via mobile devices. In International Confer-
ence on Image and Signal Processing, pages 350–357.
Springer.
Vasconcelos, M. J. M., Rosado, L., and Ferreira, M. (2014).
Principal axes-based asymmetry assessment method-
ology for skin lesion image analysis. In Interna-
tional symposium on visual computing, pages 21–31.
Springer.
HEALTHINF 2021 - 14th International Conference on Health Informatics
132