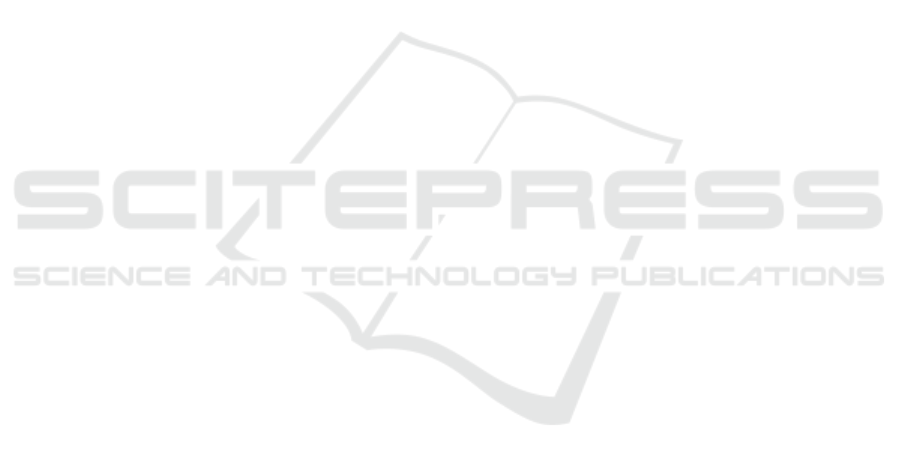
REFERENCES
Andrysiak, T. (2018). Sparse representation and over-
complete dictionary learning for anomaly detection in
electrocardiograms. Neural Computing and Applica-
tions, 32.
Aziz, O., Musngi, M., Park, E., Mori, G., and Robinovitch,
S.(2016). A comparison of accuracy of fall detec-
tion algorithms (threshold-based vs. machine learn-
ing) using waist-mounted tri-axial accelerometer sig-
nals from a comprehensive set of falls and non-fall tri-
als. Medical & Biological Engineering & Computing,
55.
Barrett, J., Gennert, M., and Michalson, W. (2012). An-
alyzing and modeling an imu for use in a low-cost
combined vision and inertial navigation system. 2012
IEEE Conference on Technologies for Practical Robot
Applications, TePRA 2012.
Casilari-P
´
erez, E. and Garc
´
ıa-Lagos, F. (2019). A compre-
hensive study on the use of artificial neural networks
in wearable fall detection systems. Expert Systems
with Applications, 138.
Casilari-P
´
erez, E., Santoyo Ram
´
on, J., and Cano-Garc
´
ıa, J.-
M. (2017). Analysis of public datasets for wearable
fall detection systems. Sensors, 17:1513.
Ceylan, R. (2018). The effect of feature extraction based on
dictionary learning on ecg signal classification. Inter-
national Journal of Intelligent Systems and Applica-
tions in Engineering, 1:40–46.
de Quadros, T., Lazzaretti, A. E., and Schneider, F. K.
(2018). A movement decomposition and machine
learning-based fall detection system using wrist wear-
able device. IEEE Sensors Journal, 18:5082–5089.
Gangeh, M., Farahat, A., Ghodsi, A., and Kamel, M. S.
(2015). Supervised dictionary learning and sparse
representation-a review.
Jiang, Z., Lin, Z., and Davis, L. S. (2011). Learning a dis-
criminative dictionary for sparse coding via label con-
sistent k-svd. pages 1697–1704.
Jiang, Z., Lin, Z., and Davis, L. S. (2013). Label consistent
k-svd: Learning a discriminative dictionary for recog-
nition. IEEE Transactions on Pattern Analysis and
Machine Intelligence, 35(11):2651–2664.
Kj, N., Sreejith, A., Mathew, J., Sarpotdar, M., Suresh,
A., Prakash, A., Safonova, M., and Murthy, J.(2016).
Noise modeling and analysis of an imu-based attitude
sensor: Improvement of performance by filtering and
sensor fusion. page 99126W.
Madgwick, S. O. H. (2010). An efficient orientation filter
for inertial and inertial/ magnetic sensor arrays.
Mo, H., Luo, C., and Jan, G. E. (2017). Eeg classification
based on sparse representation. In 2017 International
Joint Conf. on Neural Networks(IJCNN), pages 59–
62.
Othmen, F., Baklouti, M., Lazzaretti, A. E., Jmal, M., and
Abid, M. (2020). A novel on-wrist fall detection sys-
tem using supervised dictionary learning technique. In
The Impact of Digital Technologies on Public Health
in Developed and Developing Countries, pages 184–
196, Cham. Springer International Publishing.
Ozdemir, A. (2016). An analysis on sensor locations of
the human body for wearable fall detection devices:
Principles and practice. Sensors, 16:1161.
Pannurat, N., Thiemjarus, S., and Nantajeewarawat, E.
(2014). Automatic fall monitoring: A review. Sen-
sors (Basel, Switzerland), 14:12900–12936.
Ramachandran, A. and Karuppiah, A. (2020). A survey
on recent advances in wearable fall detection systems.
BioMed Research International, 2020.
Ramirez, I., Sprechmann, P., and Sapiro, G. (2010). Clas-
sification and clustering via dictionary learning with
structured incoherence and shared features. pages
3501–3508.
Sheykhivand, S., Rezaii, T. Y., Mousavi, Z., Delpak,
A., and Farzamnia, A. (2020). Automatic identifi-
cation of epileptic seizures from eeg signals using
sparse representation-based classification. IEEE Ac-
cess, 8:138834–138845.
Suo, Y., Dao, M., Srinivas, U., Monga, V., and Tran, T. D.
(2014). Structured dictionary learning for classifica-
tion.
Vallabh, P. and Malekian, R. (2017). Fall detection moni-
toring systems: a comprehensive review. Journal of
Ambient Intelligence and Humanized Computing.
Vallabh, P., Malekian, R., Ye, N., and Capeska Bogatinoska,
D. (2016). Fall detection using machine learning al-
gorithms.
Vu, T. and Monga, V. (2016). Fast low-rank shared dictio-
nary learning for image classification. IEEE Transac-
tions on Image Processing.
Vu, T. H. and Monga, V. (2016). Learning a low-rank shared
dictionary for object classification, pages 4428–4432.
World Health Organization (2008). WHO global report on
falls prevention in older age. NIH Publication.
World Health Organization (2019). Icd-11 for
mortality and morbidity statistics (version
04/2019). https://icd.who.int/browse11/l-m/en#/,
http://id.who.int/icd/entity/134290789, Last accessed
on 09.11.2019.
World Health Organization and US National Institute of Ag-
ing (2011). Global health and ageing. NIH Publica-
tion.
Wright, J., Yang, A. Y., Ganesh, A., Sastry, S. S., and Ma,
Y.(2009). Robust face recognition via sparse repre-
sentation. IEEE Transactions on Pattern Analysis and
Machine Intelligence, 31(2):210–227.
Xu, T., Zhou, Y., and Zhu, J. (2018). New advances and
challenges of fall detection systems: A survey. Ap-
plied Sciences, 8:418.
Xu, Y., Li, Z., Yang, J., and Zhang, D. (2017). A survey
of dictionary learning algorithms for face recognition.
IEEE Access, pages 1–1.
Yang, M., Zhang, L., Feng, X., and Zhang, D. (2011).
Fisher discrimination dictionary learning for sparse
representation. pages 543–550.
Zhang, H., Alrifaai, M., Zhou, K., and Hu, H. (2019). A
novel fuzzy logic algorithm for accurate fall detection
of smart wristband. Transactions of the Institute of
Measurement and Control, page 014233121988157.
HEALTHINF 2021 - 14th International Conference on Health Informatics
416