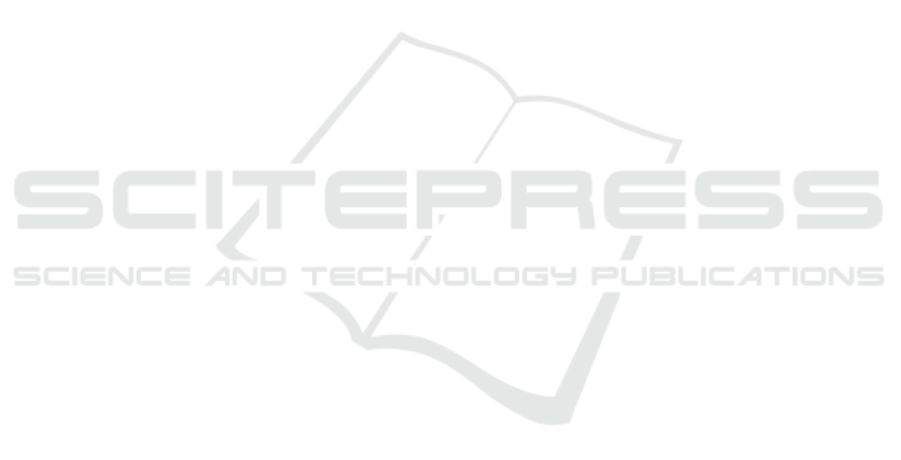
being ADHD synonymous with academic failure.
Therefore, it is necessary to understand the envi-
ronment of that child or adolescent with ADHD be-
fore creating strategies that aim to remedy or alleviate
the educational problems faced by them, since envi-
ronmental and emotional factors directly affect their
school performance, contributing to the difficulties al-
ready existing in the daily lives of people with ADHD
are expanded.
As future work is intended to evaluate new bal-
ancing distributions with the undersampling approach
since the one used in this work was the uniform distri-
bution. Besides, it aims to investigate what the results
would be like if the problem were treated as a multi-
label. In other words, it is intended to evaluate the
quality of the models in predicting the performance
of students in all subjects, jointly.
ACKNOWLEDGEMENTS
This study was financed in part by the Coordination
for the Improvement of Higher Education Personnel
- Brasil (CAPES) - Finance Code 001. The authors
thank the National Council for Scientific and Techno-
logical Development of Brazil (CNPq - Conselho Na-
cional de Desenvolvimento Cient
´
ıfico e Tecnol
´
ogico)
and the Foundation for Research Support of the Mi-
nas Gerais State (FAPEMIG). The work was devel-
oped at the Pontifical Catholic University of Minas
Gerais, PUC Minas in the Applied Computational In-
telligence laboratory – LICAP.
REFERENCES
ABDA, Associac¸
˜
ao Brasileira do D
´
eficit de Atenc¸
˜
ao
(2016). Motivac¸
˜
ao
´
e coisa s
´
eria. https://tdah.org.br/
motivacao-e-coisa-seria/. Online; access October 5,
2020.
Ahmed, A. and Elaraby, I. S. (2014). Data mining: A pre-
diction for student’s performance using classification
method. World Journal of Computer Application and
Technology, 2(2):43–47.
Andrade, A. L. M. and Lohr J
´
unior, A. (2017).
Quest
˜
oes atuais acerca do transtorno de d
´
eficit
de atenc¸
˜
ao/hiperatividade. Psicologia Argumento,
25(48):73–83.
Angeli, C., Howard, S. K., Ma, J., Yang, J., and Kirschner,
P. A. (2017). Data mining in educational technol-
ogy classroom research: Can it make a contribution?
Computers & Education, 113:226 – 242.
Anuradha, J., Tisha, Ramachandran, V., Arulalan, K. V.,
and Tripathy, B. K. (2010). Diagnosis of adhd using
svm algorithm. In Proceedings of the Third Annual
ACM Bangalore Conference, COMPUTE ’10, New
York, NY, USA. Association for Computing Machin-
ery.
APA, A. P. A. et al. (2014). DSM-5: Manual diagn
´
ostico e
estat
´
ıstico de transtornos mentais. Artmed Editora.
Aradhya, A. M. S., Sundaram, S., and Pratama, M. (2020).
Metaheuristic spatial transformation (mst) for accu-
rate detection of attention deficit hyperactivity disor-
der (adhd) using rs-fmri. In 2020 42nd Annual In-
ternational Conference of the IEEE Engineering in
Medicine Biology Society (EMBC), pages 2829–2832.
Ara
´
ujo, A. P. d. Q. C. (2002). Avaliac¸
˜
ao e manejo de crianc¸a
com dificuldade escolar e dist
´
urbio de atenc¸
˜
ao. Jornal
de Pediatria, 78:S104–S110.
Borges, H. B. and Nievola, J. C. (2012). Comparing
the dimensionality reduction methods in gene expres-
sion databases. Expert Systems with Applications,
39(12):10780–10795.
Cardoso, L., Mollica, A. M. V., Sales, A. M., and Ara
´
ujo,
L. C. (2019). O l
´
udico e a aprendizagem de crianc¸as
com transtorno de d
´
eficit de atenc¸
˜
ao/hiperatividade.
Revista Cient
´
ıfica FAGOC-Multidisciplinar, 3(2).
Carvalho, M. P. d. (2007). Por que tantos meninos v
˜
ao mal
na escola? crit
´
erios de avaliac¸
˜
ao escolar segundo o
sexo. Cadernos de Pesquisa.
Cohen, W. W. (1995). Fast effective rule induction. In
Twelfth International Conference on Machine Learn-
ing, pages 115–123. Morgan Kaufmann.
Cortez, M. T. and Pinheiro,
ˆ
A. M. V. (2018). Tdah e escola:
incompatibilidade? Paid
´
eia, 13(19).
da Rocha Antony, S. M. (2009). Os ajustamentos cria-
tivos da crianc¸a em sofrimento: uma compreens
˜
ao
da gestalt-terapia sobre as principais psicopatologias
da inf
ˆ
ancia. Estudos e pesquisas em Psicologia,
9(2):356–375.
de Azevedo Santos, J. L. (2017). Tdah-transtorno de d
´
eficit
de atenc¸
˜
ao e hiperatividade: Intervenc¸
˜
ao psicope-
dag
´
ogica. Ideias e Inovac¸
˜
ao-Lato Sensu, 4(1):115.
de Lacerda, E. G. and de Carvalho, A. (1999). Introduc¸
˜
ao
aos algoritmos gen
´
eticos. Sistemas inteligentes:
aplicac¸oes a recursos hıdricos e ci
ˆ
encias ambientais,
1:99–148.
de Lima, C. B. and Coelho, C. L. M. (2018). Transtorno de
d
´
eficit de atenc¸
˜
ao/hiperatividade-um olhar sob a per-
spectiva da educac¸
˜
ao especial inclusiva. Cadernos de
Pesquisa em Educac¸
˜
ao, 20(47):172–192.
dos Santos, H. G., do Nascimento, C. F., Izbicki, R.,
de Oliveira Duarte, Y. A., Chiavegatto Filho, A. D. P.,
et al. (2019). Machine learning para an
´
alises predi-
tivas em sa
´
ude: exemplo de aplicac¸
˜
ao para predizer
´
obito em idosos de s
˜
ao paulo, brasil. Cad. Sa
´
ude
P
´
ublica, 35(7):e00050818.
DuPaul, G. J., Dahlstrom-Hakki, I., Gormley, M. J., Fu, Q.,
Pinho, T. D., and Banerjee, M. (2017). College stu-
dents with adhd and ld: Effects of support services
on academic performance. Learning Disabilities Re-
search & Practice, 32(4):246–256.
Fortin, F.-A., De Rainville, F.-M., Gardner, M.-A. G.,
Parizeau, M., and Gagn
´
e, C. (2012). Deap: Evolu-
tionary algorithms made easy. J. Mach. Learn. Res.,
13(1):2171–2175.
HEALTHINF 2021 - 14th International Conference on Health Informatics
164