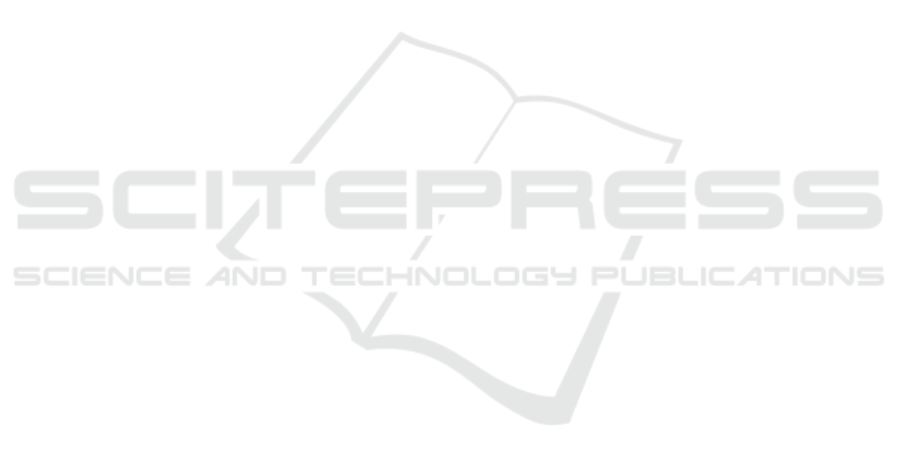
Fromme, C., Stager, D., Pilarski, T., Bancroft, B., and
Hegadorn, T. (2006). Conveyor belt inspection sys-
tem and method. US Patent 6,988,610.
fu Hou, Y. and rui Meng, Q. (2008). Dynamic characteris-
tics of conveyor belts. Journal of China University of
Mining and Technology, 18(4):629 – 633.
Genc¸Tav, A., Aksoy, S., and
¨
ONder, S. (2012). Unsuper-
vised segmentation and classification of cervical cell
images. Pattern recognition, 45(12):4151–4168.
Guan, Y., Zhang, J., Shang, Y., Wu, M., and Liu, X.
(2008). Embedded sensor of forecast conveyer belt
breaks. In Fuzzy Systems and Knowledge Discovery,
2008. FSKD’08. Fifth International Conference on,
volume 5, pages 617–621. IEEE.
Hodge, V. and Austin, J. (2004). A survey of outlier de-
tection methodologies. Artificial intelligence review,
22(2):85–126.
Jeong, J., Park, E., Han, W. S., Kim, K., Choung, S., and
Chung, I. M. (2017). Identifying outliers of non-
gaussian groundwater state data based on ensemble
estimation for long-term trends. Journal of Hydrol-
ogy, 548(Supplement C):135 – 144.
Junior, R. M. C. and Costa, L. D. F. (1996). Towards effec-
tive planar shape representation with multiscale digi-
tal curvature analysis based on signal processing tech-
niques. Pattern Recognition, 29(9):1559–1569.
Kurihara, T., Okuda, K., and Yoshimoto, M. (2006). Longi-
tudinal rip detection method and device for conveyor
belt. Japan Patent 6,282,319.
Leys, C., Ley, C., Klein, O., Bernard, P., and Licata, L.
(2013). Detecting outliers: Do not use standard devi-
ation around the mean, use absolute deviation around
the median. Journal of Experimental Social Psychol-
ogy, 49(4):764–766.
Li, J. and Miao, C. (2016). The conveyor belt longitudi-
nal tear on-line detection based on improved ssr al-
gorithm. Optik-International Journal for Light and
Electron Optics, 127(19):8002–8010.
Li, M., Du, B., Zhu, M., and Zhao, K. (2011). Intelligent de-
tection system for mine belt tearing based on machine
vision. In Control and Decision Conference (CCDC),
2011 Chinese, pages 1250–1253. IEEE.
Lin, L. and Sherman, P. D. (2007). Cleaning data the chau-
venet way. The Proceedings of the SouthEast SAS
Users Group, SESUG Proceedings, Paper SA11.
Nascimento, R., Carvalho, R., Delabrida, S., Bianchi, A. G.,
Oliveira, R. A. R., and Garcia, L. G. U. (2017). An in-
tegrated inspection system for belt conveyor rollers. In
19th International Conference on Enterprise Informa-
tion Systems (ICEIS 2017), volume 2, pages 190–200.
Nicolay, T., Treib, A., and Blum, A. (2004). Rf identifi-
cation in the use of belt rip detection [mining product
belt haulage]. In Sensors, 2004. Proceedings of IEEE,
pages 333–336. IEEE.
Otsu, N. (1979). A threshold selection method from gray-
level histograms. IEEE transactions on systems, man,
and cybernetics, 9(1):62–66.
Pang, Y. and Lodewijks, G. (2005). Large-scale conveyor
belt system maintenance decision-making by using
fuzzy causal modeling. In Intelligent Transportation
Systems, 2005. Proceedings. 2005 IEEE, pages 563–
567. IEEE.
Pang, Y. and Lodewijks, G. (2006). A novel embedded con-
ductive detection system for intelligent conveyor belt
monitoring. In Service Operations and Logistics, and
Informatics, 2006. SOLI’06. IEEE International Con-
ference on, pages 803–808. IEEE.
Peng, X. (2013). A novel image-based method for conveyor
belt rip detection. In Signal Processing, Communica-
tion and Computing (ICSPCC), 2013 IEEE Interna-
tional Conference on, pages 1–4. IEEE.
Perruchet, P. and Peereman, R. (2004). The exploitation
of distributional information in syllable processing.
Journal of Neurolinguistics, 17(2-3):97–119.
Qiao, T., Li, X., Pang, Y., L
¨
u, Y., Wang, F., and Jin, B.
(2017). Research on conditional characteristics vision
real-time detection system for conveyor belt longitu-
dinal tear. IET Science, Measurement & Technology,
11(7):955–960.
Qiao, T., Liu, W., Pang, Y., and Yan, G. (2016). Research
on visible light and infrared vision real-time detection
system for conveyor belt longitudinal tear. IET Sci-
ence, Measurement & Technology, 10(6):577–584.
Radau, P., Lu, Y., Connelly, K., Paul, G., Dick, A., and
Wright, G. (2009). Evaluation framework for algo-
rithms segmenting short axis cardiac mri.
Sasaki, Y. et al. (2007). The truth of the f-measure. Teach
Tutor mater, 1(5):1–5.
Xianguo, L., Lifang, S., Zixu, M., Can, Z., and Hangqi, J.
(2018). Laser-based on-line machine vision detection
for longitudinal rip of conveyor belt. Optik, 168:360–
369.
Yang, Y., Miao, C., Li, X., and Mei, X. (2014). On-
line conveyor belts inspection based on machine vi-
sion. Optik-International Journal for Light and Elec-
tron Optics, 125(19):5803–5807.
Yang, Y., Zhao, Y., Miao, C., and Wang, L. (2016). On-line
longitudinal rip detection of conveyor belts based on
machine vision. In Signal and Image Processing (IC-
SIP), IEEE International Conference on, pages 315–
318. IEEE.
VISAPP 2021 - 16th International Conference on Computer Vision Theory and Applications
310