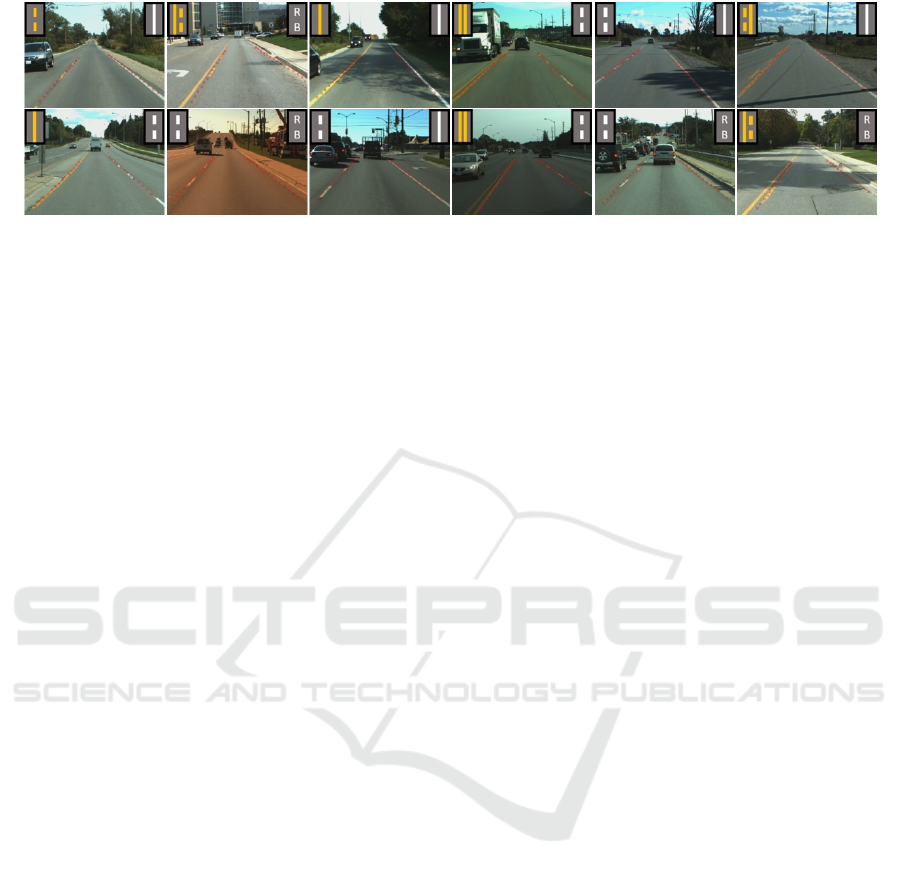
Figure 9: Output samples of our experiments on the RoadLAB dataset.
REFERENCES
Ali, A. A. and Hussein, H. A. (2019). Real-time lane mark-
ings recognition based on seed-fill algorithm. In Pro-
ceedings of the International Conference on Informa-
tion and Communication Technology, pages 190–195.
Beauchemin, S., Bauer, M., Kowsari, T., and Cho, J. (2012).
Portable and scalable vision-based vehicular instru-
mentation for the analysis of driver intentionality.
IEEE Transactions on Instrumentation and Measure-
ment, 61(2):391–401.
Cheng, H.-Y., Jeng, B.-S., Tseng, P.-T., and Fan, K.-C.
(2006). Lane detection with moving vehicles in the
traffic scenes. IEEE Transactions on intelligent trans-
portation systems, 7(4):571–582.
Chiu, K.-Y. and Lin, S.-F. (2005). Lane detection using
color-based segmentation. In IEEE Proceedings. In-
telligent Vehicles Symposium, 2005., pages 706–711.
IEEE.
Choi, H.-C. and Oh, S.-Y. (2010). Illumination invariant
lane color recognition by using road color reference
& neural networks. In The 2010 International Joint
Conference on Neural Networks (IJCNN), pages 1–5.
IEEE.
Chougule, S., Koznek, N., Ismail, A., Adam, G., Narayan,
V., and Schulze, M. (2018). Reliable multilane detec-
tion and classification by utilizing cnn as a regression
network. In Proceedings of the European Conference
on Computer Vision (ECCV).
de Paula, M. B. and Jung, C. R. (2013). Real-time detection
and classification of road lane markings. In 2013 XXVI
Conference on Graphics, Patterns and Images, pages
83–90. IEEE.
Ghafoorian, M., Nugteren, C., Baka, N., Booij, O., and Hof-
mann, M. (2018). El-gan: Embedding loss driven gen-
erative adversarial networks for lane detection. In Pro-
ceedings of the European Conference on Computer Vi-
sion (ECCV).
Goodfellow, I., Pouget-Abadie, J., Mirza, M., Xu, B.,
Warde-Farley, D., Ozair, S., Courville, A., and Ben-
gio, Y. (2014). Generative adversarial nets. In
Advances in neural information processing systems,
pages 2672–2680.
He, B., Ai, R., Yan, Y., and Lang, X. (2016). Accurate
and robust lane detection based on dual-view convo-
lutional neutral network. In 2016 IEEE Intelligent Ve-
hicles Symposium (IV), pages 1041–1046. IEEE.
Hoang, T. M., Hong, H. G., Vokhidov, H., and Park, K. R.
(2016). Road lane detection by discriminating dashed
and solid road lanes using a visible light camera sen-
sor. Sensors, 16(8):1313.
Kim, H., Park, J., Min, K., and Huh, K. (2020). Anomaly
monitoring framework in lane detection with a gener-
ative adversarial network. IEEE Transactions on In-
telligent Transportation Systems.
Kim, Z. (2008). Robust lane detection and tracking in chal-
lenging scenarios. IEEE Transactions on Intelligent
Transportation Systems, 9(1):16–26.
Lee, C. and Moon, J.-H. (2018). Robust lane detection and
tracking for real-time applications. IEEE Transactions
on Intelligent Transportation Systems, 19(12):4043–
4048.
Lee, S., Kim, J., Shin Yoon, J., Shin, S., Bailo, O., Kim, N.,
Lee, T.-H., Seok Hong, H., Han, S.-H., and So Kweon,
I. (2017). Vpgnet: Vanishing point guided network for
lane and road marking detection and recognition. In
Proceedings of the IEEE international conference on
computer vision, pages 1947–1955.
Linarth, A. and Angelopoulou, E. (2011). On feature
templates for particle filter based lane detection. In
2011 14th International IEEE Conference on Intel-
ligent Transportation Systems (ITSC), pages 1721–
1726. IEEE.
Liu, G., W
¨
org
¨
otter, F., and Markeli
´
c, I. (2010). Combining
statistical hough transform and particle filter for robust
lane detection and tracking. In 2010 IEEE Intelligent
Vehicles Symposium, pages 993–997. IEEE.
Liu, T., Chen, Z., Yang, Y., Wu, Z., and Li, H. (2020).
Lane detection in low-light conditions using an effi-
cient data enhancement: Light conditions style trans-
fer. arXiv preprint arXiv:2002.01177.
Lo, S.-Y., Hang, H.-M., Chan, S.-W., and Lin, J.-J. (2019a).
Efficient dense modules of asymmetric convolution
for real-time semantic segmentation. In Proceedings
of the ACM Multimedia Asia, pages 1–6.
Lo, S.-Y., Hang, H.-M., Chan, S.-W., and Lin, J.-J. (2019b).
Multi-class lane semantic segmentation using efficient
convolutional networks. In 2019 IEEE 21st Inter-
national Workshop on Multimedia Signal Processing
(MMSP), pages 1–6. IEEE.
VISAPP 2021 - 16th International Conference on Computer Vision Theory and Applications
456