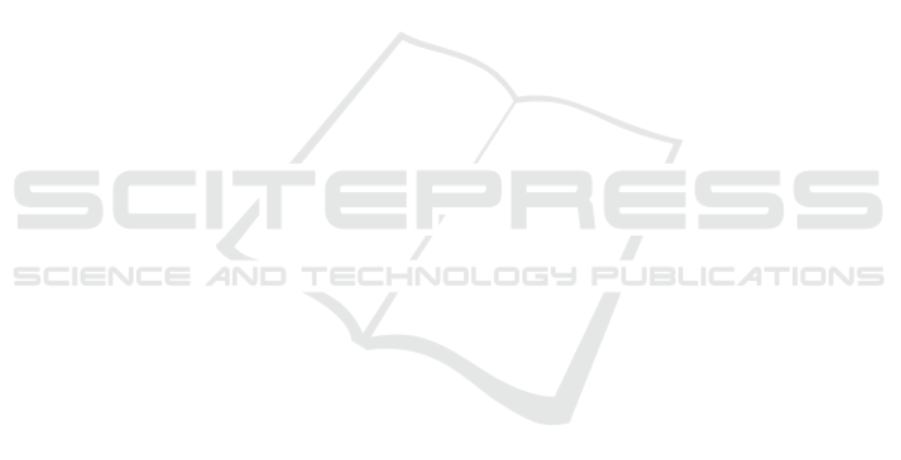
Collins, M. H., Martin, L. J., Alexander, E. S., Boyd, J. T.,
Sheridan, R., He, H., Pentiuk, S., Putnam, P. E., Abo-
nia, J. P., Mukkada, V. A., Franciosi, J. P., and Rothen-
berg, M. E. (2017). Newly developed and validated
eosinophilic esophagitis histology scoring system and
evidence that it outperforms peak eosinophil count for
disease diagnosis and monitoring. Diseases of the
Esophagus : Official Journal of the International So-
ciety for Diseases of the Esophagus, 30(3):1–8.
Dellon, E. S. (2014). Epidemiology of eosinophilic
esophagitis. Gastroenterology Clinics, 43(2):201–
218.
Dellon, E. S., Kim, H. P., Sperry, S. L., Rybnicek, D. A.,
Woosley, J. T., and Shaheen, N. J. (2014). A pheno-
typic analysis shows that eosinophilic esophagitis is
a progressive fibrostenotic disease. Gastrointestinal
Endoscopy, 79(4):577–585.
Eelbode, T., Bertels, J., Berman, M., Vandermeulen, D.,
Maes, F., Bisschops, R., and Blaschko, M. B. (2020).
Optimization for medical image segmentation: The-
ory and practice when evaluating with dice score or
jaccard index. IEEE Transactions on Medical Imag-
ing.
Furuta, G. T., Liacouras, C. A., Collins, M. H., Gupta,
S. K., Justinich, C., Putnam, P. E., Bonis, P., Hassall,
E., Straumann, A., Rothenberg, M. E., et al. (2007).
Eosinophilic esophagitis in children and adults: A sys-
tematic review and consensus recommendations for
diagnosis and treatment: Sponsored by the Ameri-
can Gastroenterological Association (AGA) institute
and north American society of pediatric gastroenterol-
ogy, hepatology, and nutrition. Gastroenterology,
133(4):1342–1363.
Godwin, B., Wilkins, B., and Muir, A. B. (2020). Eoe dis-
ease monitoring: Where we are and where we are
going. Annals of Allergy, Asthma & Immunology,
124(3):240–247.
Habibzadeh, M., Jannesari, M., Rezaei, Z., Baharvand, H.,
and Totonchi, M. (2018). Automatic white blood cell
classification using pre-trained deep learning models:
Resnet and inception. In Tenth International Confer-
ence on Machine Vision (ICMV 2017), volume 10696,
page 1069612. International Society for Optics and
Photonics.
Heinz, B., Gil, J., Kirkpatrick, D., and Werman, M. (1995).
Linear time Euclidean distance transform algorithms.
IEEE Transactions on Pattern Analysis and Machine
Intelligence, 17(5):529–533.
Kingma, D. and Ba, J. (2014). Adam: A method for
stochastic optimization. International Conference on
Learning Representations.
Kumar, N., Verma, R., Anand, D., Zhou, Y., Onder, O. F.,
Tsougenis, E., Chen, H., Heng, P. A., Li, J., Hu, Z.,
et al. (2019). A multi-organ nucleus segmentation
challenge. IEEE Transactions on Medical Imaging.
Liacouras, C. A., Furuta, G. T., Hirano, I., Atkins, D.,
Attwood, S. E., Bonis, P. A., Burks, A. W., Chehade,
M., Collins, M. H., Dellon, E. S., et al. (2011).
Eosinophilic esophagitis: Updated consensus recom-
mendations for children and adults. Journal of Allergy
and Clinical Immunology, 128(1):3–20.
Liang, G., Hong, H., Xie, W., and Zheng, L. (2018). Com-
bining convolutional neural network with recursive
neural network for blood cell image classification.
IEEE Access, 6:36188–36197.
Lipka, S., Kumar, A., and Richter, J. E. (2016). Impact of
diagnostic delay and other risk factors on eosinophilic
esophagitis phenotype and esophageal diameter. Jour-
nal of Clinical Gastroenterology, 50(2):134–140.
Milletari, F., Navab, N., and Ahmadi, S.-A. (2016). V-
net: Fully convolutional neural networks for volumet-
ric medical image segmentation. In 2016 Fourth Inter-
national Conference on 3D Vision (3DV), pages 565–
571. IEEE.
Nielsen, J. A., Lager, D. J., Lewin, M., Rendon, G., and
Roberts, C. A. (2014). The optimal number of biopsy
fragments to establish a morphologic diagnosis of
eosinophilic esophagitis. The American Journal of
Gastroenterology, 109(4):515.
Oktay, O., Schlemper, J., Folgoc, L. L., Lee, M., Heinrich,
M., Misawa, K., Mori, K., McDonagh, S., Hammerla,
N. Y., Kainz, B., et al. (2018). Attention u-net: Learn-
ing where to look for the pancreas. International Con-
ference on Medical Imaging with Deep Learning.
¨
Ozyurt, F. (2019). A fused CNN model for WBC detection
with MRMR feature selection and extreme learning
machine. Soft Computing, pages 1–10.
Reed, C. C., Koutlas, N. T., Robey, B. S., Hansen, J.,
and Dellon, E. S. (2018). Prolonged time to diag-
nosis of eosinophilic esophagitis despite increasing
knowledge of the disease. Clinical Gastroenterology
and Hepatology: the Official Clinical Practice Jour-
nal of the American Gastroenterological Association,
16(10):1667.
Ronneberger, O., Fischer, P., and Brox, T. (2015). U-
Net: Convolutional networks for biomedical image
segmentation. In International Conference on Medi-
cal image computing and computer-assisted interven-
tion, pages 234–241. Springer.
Rosenberg, H. F., Dyer, K. D., and Foster, P. S. (2012).
Eosinophils: changing perspectives in health and dis-
ease. Nature Reviews Immunology, 13(1):9–22.
Runge, T. M., Eluri, S., Cotton, C. C., Burk, C. M.,
Woosley, J. T., Shaheen, N. J., and Dellon, E. S.
(2017). Causes and outcomes of esophageal perfo-
ration in eosinophilic esophagitis. Journal of Clinical
Gastroenterology, 51(9):805.
Russakovsky, O., Deng, J., Su, H., Krause, J., Satheesh, S.,
Ma, S., Huang, Z., Karpathy, A., Khosla, A., Bern-
stein, M., et al. (2015). Imagenet large scale visual
recognition challenge. International journal of com-
puter vision, 115(3):211–252.
Selvaraju, R. R., Cogswell, M., Das, A., Vedantam, R.,
Parikh, D., and Batra, D. (2017). Grad-CAM: Visual
explanations from deep networks via gradient-based
localization. In Proceedings of the IEEE International
Conference on Computer Vision, pages 618–626.
Simonyan, K. and Zisserman, A. (2015). Very deep con-
volutional networks for large-scale image recognition.
In International Conference on Learning Representa-
tions.
BIOIMAGING 2021 - 8th International Conference on Bioimaging
54