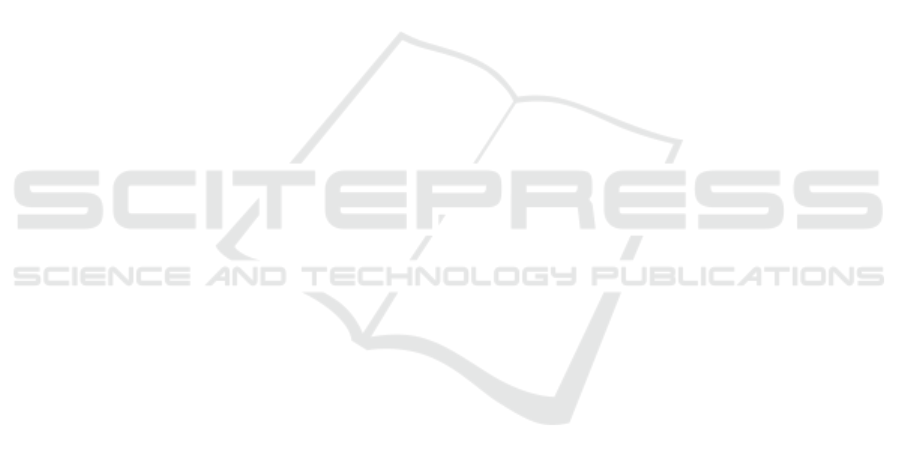
Chen, Q., Zhang, D., Pan, W., Ouyang, Q., Li, H., Ur-
mila, K., and Zhao, J. (2015). Recent developments of
green analytical techniques in analysis of tea’s quality
and nutrition. Trends in Food Science & Technology,
43(1):63 – 82.
de Lima, A. B. S., Batista, A. S., de Jesus, J. C., de Je-
sus Silva, J., de Ara
´
ujo, A. C. M., and Santos, L. S.
(2020). Fast quantitative detection of black pepper and
cumin adulterations by near-infrared spectroscopy and
multivariate modeling. Food Control, 107:106802.
Galli, M., Pagni, F., De Sio, G., Smith, A., Chinello, C.,
Stella, M., L’Imperio, V., Manzoni, M., Garancini, M.,
Massimini, D., et al. (2017). Proteomic profiles of thy-
roid tumors by mass spectrometry-imaging on tissue
microarrays. Biochimica et Biophysica Acta (BBA)-
Proteins and Proteomics, 1865(7):817–827.
Galli, M., Zoppis, I., De Sio, G., Chinello, C., Pagni, F.,
Magni, F., and Mauri, G. (2016). A support vector ma-
chine classification of thyroid bioptic specimens using
maldi-msi data. Advances in bioinformatics, 2016.
Grossi, M., Di Lecce, G., Arru, M., Gallina Toschi, T., and
Ricc
`
o, B. (2015). An opto-electronic system for in-
situ determination of peroxide value and total phenol
content in olive oil. J. of Food Engineering, 146:1 – 7.
Huang, H., Yu, H., Xu, H., and Ying, Y. (2008). Near in-
frared spectroscopy for on/in-line monitoring of qual-
ity in foods and beverages: A review. J. of Food Engi-
neering, 87(3):303 – 313.
Kingma, D. P. and Ba, J. (2015). Adam: A method for
stochastic optimization. In Bengio, Y. and LeCun,
Y., editors, 3rd International Conference on Learn-
ing Representations, ICLR 2015, San Diego, CA, USA,
May 7-9, 2015, Conference Track Proceedings.
Li, Z., Tang, X., Shen, Z., Yang, K., Zhao, L., and Li,
Y. (2019). Comprehensive comparison of multiple
quantitative near-infrared spectroscopy models for as-
pergillus flavus contamination detection in peanut. J.
of the Science of Food and Agriculture, 99(13):5671–
5679.
Liu, Y., Li, Y., Peng, Y., Yang, Y., and Wang, Q. (2020). De-
tection of fraud in high-quality rice by near-infrared
spectroscopy. Journal of Food Science.
Nebauer, C. (1998). Evaluation of convolutional neural net-
works for visual recognition. IEEE Transactions on
Neural Networks, 9(4):685–696.
Ng, W., Minasny, B., Montazerolghaem, M., Padarian, J.,
Ferguson, R., Bailey, S., and McBratney, A. B. (2019).
Convolutional neural network for simultaneous pre-
diction of several soil properties using visible/near-
infrared, mid-infrared, and their combined spectra.
Geoderma, 352:251 – 267.
Ni, C., Wang, D., and Tao, Y. (2019). Variable weighted
convolutional neural network for the nitrogen content
quantization of masson pine seedling leaves with near-
infrared spectroscopy. Spectrochimica Acta Part A:
Molecular and Biomolecular Spectroscopy, 209:32 –
39.
Osborne, B. G. (2006). Near-infrared spectroscopy in food
analysis. Enc. of analytical chemistry: applications,
theory and instrumentation.
Qingyun, L., Yeming, C., Mikami, T., Kawano, M., and
Zaigui, L. (2007). Adaptability of four-samples sen-
sory tests and prediction of visual and near-infrared
reflectance spectroscopy for chinese indica rice. J. of
Food Engineering, 79(4):1445 – 1451.
Shin, H., Roth, H. R., Gao, M., Lu, L., Xu, Z., Nogues,
I., Yao, J., Mollura, D., and Summers, R. M. (2016).
Deep convolutional neural networks for computer-
aided detection: Cnn architectures, dataset charac-
teristics and transfer learning. IEEE Trans. on Med.
Imaging, 35(5):1285–1298.
Sun, X., Zhu, K., and Liu, J. (2019). Nondestructive detec-
tion of reducing sugar of potato flours by near infrared
spectroscopy and kernel partial least square algorithm.
Journal of Food Measurement and Characterization,
13(1):231–237.
Teye, E., Amuah, C. L., McGrath, T., and Elliott, C.
(2019). Innovative and rapid analysis for rice authen-
ticity using hand-held nir spectrometry and chemo-
metrics. Spectrochimica Acta Part A: Molecular and
Biomolecular Spectroscopy, 217:147 – 154.
´
Eva Szab
´
o, Gergely, S., Spaits, T., Simon, T., and Salg
´
o,
A. (2019). Near-infrared spectroscopy-based methods
for quantitative determination of active pharmaceuti-
cal ingredient in transdermal gel formulations. Spec-
troscopy Letters, 52(10):599–611.
V
´
asconez, M.,
´
Edgar P
´
erez-Esteve, Arnau-Bonachera, A.,
Barat, J., and Talens, P. (2018). Rapid fraud detection
of cocoa powder with carob flour using near infrared
spectroscopy. Food Control, 92:183 – 189.
Wang, H., Lv, D., Dong, N., Wang, S., and Liu, J. (2019).
Application of near-infrared spectroscopy for screen-
ing the potato flour content in chinese steamed bread.
Food science and biotechnology, 28(4):955–963.
Windham, W. R., Lyon, B. G., Champagne, E. T., Bar-
ton, F. E., Webb, B. D., McClung, A. M., Molden-
hauer, K. A., Linscombe, S., and McKenzie, K. S.
(1997). Prediction of cooked rice texture quality us-
ing near-infrared reflectance analysis of whole-grain
milled samples. Cereal Chemistry, 74(5):626–632.
Wu, D., Feng, S., and He, Y. (2008). Short-wave near-
infrared spectroscopy of milk powder for brand iden-
tification and component analysis. Journal of Dairy
Science, 91(3):939 – 949.
Zareef, M., Chen, Q., Hassan, M. M., Arslan, M., Hashim,
M. M., Ahmad, W., Kutsanedzie, F. Y., and Agyekum,
A. A. (2020). An overview on the applications of
typical non-linear algorithms coupled with nir spec-
troscopy in food analysis. Food Engineering Reviews,
pages 1–18.
Zhang, L., Ding, X., and Hou, R. (2020). Classification
modeling method for near-infrared spectroscopy of to-
bacco based on multimodal convolution neural net-
works. Journal of Analytical Methods in Chemistry,
2020.
Zoppis, I., Gianazza, E., Borsani, M., Chinello, C., Mainini,
V., Galbusera, C., Ferrarese, C., Galimberti, G., Sorbi,
S., Borroni, B., et al. (2011). Mutual information opti-
mization for mass spectra data alignment. IEEE/ACM
transactions on computational biology and bioinfor-
matics, 9(3):934–939.
BIOSIGNALS 2021 - 14th International Conference on Bio-inspired Systems and Signal Processing
176