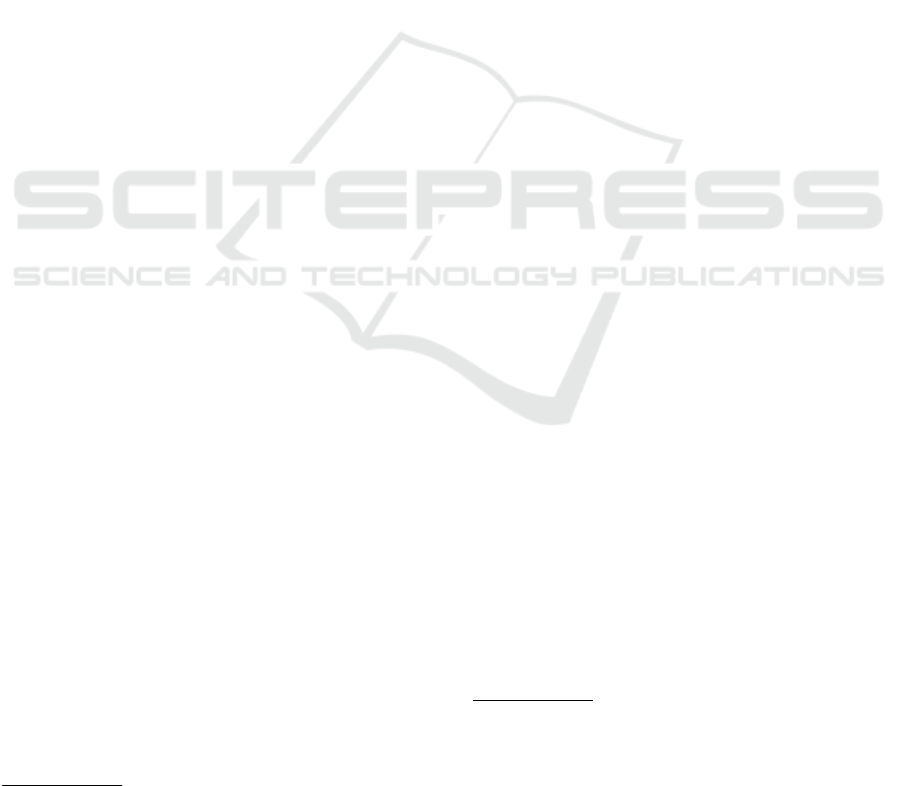
mental health needs during the pandemic time. Xiang
et al. (Xiang et al., 2020) claim that the mental health
needs of patients with confirmed COVID-19, patients
with suspected infection, quarantined family mem-
bers, and medical personnel have been poorly han-
dled throughout this time. Similarly, Yao et al. (Yao
et al., 2020) express their concerns with regards to
the effect of the pandemic on people with mental
health disorders. The obvious solution for Wind et
al. (Wind et al., 2020) to continue mental health care
within a pandemic is to provide mental health care at
a warm distance by video-conferencing psychother-
apy and internet interventions. This idea is further
supported by a study among Chinese citizens (Gao
et al., 2020), in which Gao et al. assess the prevalence
of mental health problems and examine their associa-
tion with social media exposure. Their findings high-
light that there is a link between the two, which sug-
gests that the government need to pay more attention
to mental health problems and their online manifesta-
tion. A portuguese study also found that individuals
previously receiving psychotherapeutic support ben-
efit if they did not interrupt the process as a conse-
quence of the outbreak (Moreira et al., 2020).
The already available scientific evidence previ-
ously introduced strongly suggests that a shift in men-
tal health care provision towards online prevention
and treatment in the near future is needed. As such,
the widespread use of social media combined with
the rapid development of computational infrastruc-
tures to support big data, and the maturation of natural
language processing and machine learning technolo-
gies offer exciting possibilities for the improvement
of both population-level and individual-level mental
health (Conway and O’Connor, 2016).
3 DATA COLLECTION
This work builds on prior research contributions, both
national and international, that enabled the collection
of both social media and clinical statistics data. For
social media data, we collected four and a half months
of Twitter
1
posts whose content contained coron-
avirus related vocabulary, as explained next. The data
collection process followed the pipeline recently pub-
lished by Chen et. al (Chen et al., 2020). According to
Twitter’s Terms and Conditions, Chen et. al have re-
leased Tweet IDs, which are unique identifiers tied to
specific tweets containing coronavirus related terms.
The collection of ids is available on GitHub
2
.
1
www.twitter.com
2
https://github.com/echen102/COVID-19-TweetIDs
Based on this dataset, we queried the Twitter API
and obtained the complete dataset. For each tweet
in the collection we retrieved tweet content (text,
URLs, hashtags) and authors’ metadata. Follow-
ing authors’ suggestions, we used Hydrator
3
, Twit-
ter’s search API
4
and Twarc
5
for retrieving the data.
All tweets included in this collection contain coron-
avirus related vocabulary, both as hashtags or men-
tions/keywords in the text of the tweet. We collected
tweets posted from 21st of January to 31st of May,
2020. More details on the collection process can be
found in the original publication (Chen et al., 2020).
We filtered the collected tweets based on the
tweet’s geo-location or user’s location. When users
post tweets from a GPS-enabled device or they tag
a location in their tweet, this information can be re-
trieved as longitude and latitudine coordinates. Un-
fortunately, only roughly 1–3% of Twitter messages
are geocoded (Paul and Dredze, 2017). Because the
number of geo-located tweets in the corpora we col-
lected is indeed limited, we used the location de-
fined on the user’s profile for extracting tweets of Por-
tuguese users. Using Tweepy
6
we filter for user loca-
tions that match a dictionary of city names in Por-
tugal. We filter out false positives by removing lo-
cations that also match names of cities or states in
other countries, namely Brazil (e.g. a match for Porto
Alegre, a city in Brazil, replaces a match for the Por-
tuguese city Porto). We ended up with a list of 76898
distinct users and 117772 distinct tweets.
For clinical statistics and the identification of key
events of the manifestation of the COVID-19 pan-
demic in Portugal we relied on an open-source re-
spository maintained by Data Science for Social Good
Portugal
7
, an open community of data scientists that
tackle relevant and current societal issues. This repos-
itory contains daily updates of the statistic informa-
tion released by the Portuguese Ministery of Health
regarding COVID-19 cases in Portugal.
4 DATA ANALYSIS
Psychologist Paul Eckman identified six basic emo-
tions that he suggested were universally experienced
in all human cultures. These emotions were happiness
(joy), sadness, disgust, fear, surprise, and anger (Ek-
man, 1992). Happiness is often classified as a posi-
3
https://github.com/DocNow/hydrator
4
https://developer.twitter.com/en/docs/tweets/search/api-
reference/get-search-tweets
5
https://github.com/DocNow/twarc
6
https://www.tweepy.org/
7
https://github.com/dssg-pt/covid19pt-data
Bilingual Emotion Analysis on Social Media throughout the COVID19 Pandemic in Portugal
431