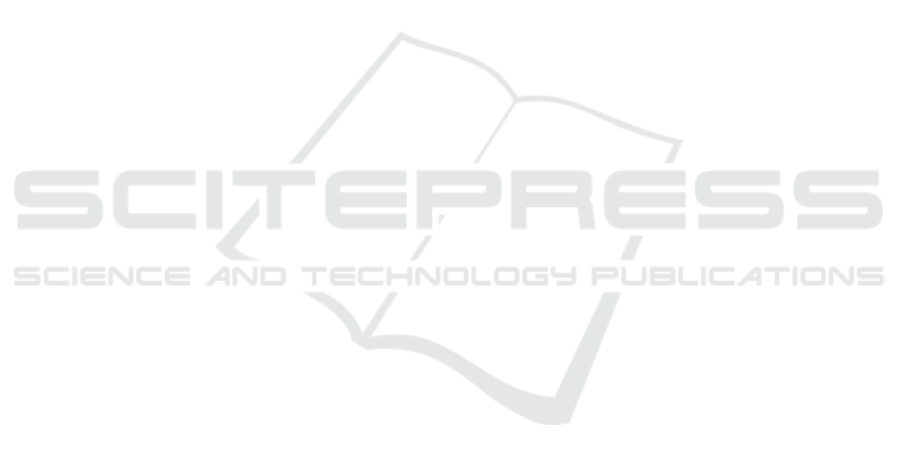
detection gets a better outcome when applying image-
based solutions. However, when computational per-
formance must be taken into account, video-based
neural networks are more feasible, especially models
with 3D convolutions. We have demonstrated the
possibilities the DMD offers to the scientific commu-
nity, extending the discussion for better solutions to
action recognition problems applied to a driver mo-
nitoring context. Finally, we share some thoughts on
some issues this line of research might encounter and
propose some future work with the DMD.
ACKNOWLEDGEMENTS
This work has received funding from Basque Gov-
ernment under project AUTOLIB of the program EL-
KARTEK 2019.
REFERENCES
Abouelnaga, Y., Eraqi, H. M., and Moustafa, M. N. (2018).
Real-time Distracted Driver Posture Classification. In
32nd Conference on Neural Information Processing
Systems (NIPS 2018).
Baheti, B. V., Talbar, S., and Gajre, S. (2020). To-
wards computationally efficient and realtime dis-
tracted driver detection with mobilevgg network.
IEEE Transactions on Intelligent Vehicles.
Borghi, G., Venturelli, M., Vezzani, R., and Cucchiara, R.
(2017). Poseidon: Face-from-depth for driver pose
estimation. In IEEE Conference on Computer Vision
and Pattern Recognition (CVPR).
Chan, A., Saleem, K., Bhutto, Z., Memon, L., Shaikh, M.,
Ahmed, S., and Siyal, A. (2019). Feature fusion based
human action recognition in still images.
Chen, J.-C., Lee, C.-Y., Huang, P.-Y., and Lin, C.-R. (2020).
Driver behavior analysis via two-stream deep convo-
lutional neural network. Applied Sciences.
Deo, N. and Trivedi, M. M. (2018). Looking at the
driver/rider in autonomous vehicles to predict take-
over readiness.
Donahue, J., Hendricks, L. A., Rohrbach, M., Venugopalan,
S., Guadarrama, S., Saenko, K., and Darrell, T.
(2014). Long-term recurrent convolutional networks
for visual recognition and description.
Girish, D., Singh, V., and Ralescu, A. (2020). Under-
standing action recognition in still images. In 2020
IEEE/CVF Conference on Computer Vision and Pat-
tern Recognition Workshops (CVPRW).
Howard, A. G., Zhu, M., Chen, B., Kalenichenko, D.,
Wang, W., Weyand, T., Andreetto, M., and Adam,
H. (2017). Mobilenets: Efficient convolutional neu-
ral networks for mobile vision applications.
Krizhevsky, A., Sutskever, I., and Hinton, G. E. (2012).
Imagenet classification with deep convolutional neu-
ral networks. In Proceedings of the 25th Interna-
tional Conference on Neural Information Processing
Systems.
Martin, M., Roitberg, A., Haurilet, M., Horne, M., Reiss, S.,
Voit, M., and Stiefelhagen, R. (2019). Drive & Act: A
Multi-modal Dataset for Fine-Grained Driver Behav-
ior Recognition in Autonomous Vehicles. In The IEEE
International Conference on Computer Vision (ICCV).
Ortega, J. D., Kose, N., Ca
˜
nas, P., Chao, M.-A., Unnervik,
A., Nieto, M., Otaegui, O., and Salgado, L. (2020).
Dmd: A large-scale multi-modal driver monitoring
dataset for attention and alertness analysis.
Rangesh, A. and Trivedi, M. M. (2018). Handynet: A one-
stop solution to detect, segment, localize & analyze
driver hands.
SAE International (2018). Taxonomy and Definitions for
Terms Related to Driving Automation Systems for
On-Road Motor Vehicles. Technical report, SAE In-
ternational.
Shi, X., Chen, Z., Wang, H., Yeung, D., Wong, W., and
Woo, W. (2015). Convolutional LSTM network: A
machine learning approach for precipitation nowcast-
ing.
StateFarm (2016). State Farm Distracted Driver De-
tection. Online source: https://www.kaggle.com/c/
state-farm-distracted-driver-detection.
Szegedy, C., Vanhoucke, V., Ioffe, S., Shlens, J., and Wojna,
Z. (2015). Rethinking the inception architecture for
computer vision.
Tran, D., Bourdev, L. D., Fergus, R., Torresani, L., and
Paluri, M. (2015). Learning spatiotemporal features
with 3d convolutional networks. 2015 IEEE Interna-
tional Conference on Computer Vision (ICCV).
Tran, D., Manh Do, H., Sheng, W., Bai, H., and Chowdhary,
G. (2018). Real-time detection of distracted driving
based on deep learning. IET Intelligent Transport Sys-
tems.
Xing, Y., Lv, C., Wang, H., Cao, D., Velenis, E., and Wang,
F.-Y. (2019). Driver activity recognition for intelligent
vehicles: A deep learning approach. IEEE Transac-
tions on Vehicular Technology.
Yang, W., Wang, Y., and Mori, G. (2010). Recognizing hu-
man actions from still images with latent poses. In
2010 IEEE Computer Society Conference on Com-
puter Vision and Pattern Recognition.
Yuen, K., Martin, S., and Trivedi, M. M. (2016). Look-
ing at faces in a vehicle: A deep cnn based approach
and evaluation. In 2016 IEEE 19th International Con-
ference on Intelligent Transportation Systems (ITSC).
IEEE.
Zhang, Y., Cheng, L., Wu, J., Cai, J., Do, M. N., and Lu, J.
(2016). Action recognition in still images with mini-
mum annotation efforts. IEEE Transactions on Image
Processing.
Detection of Distraction-related Actions on DMD: An Image and a Video-based Approach Comparison
465