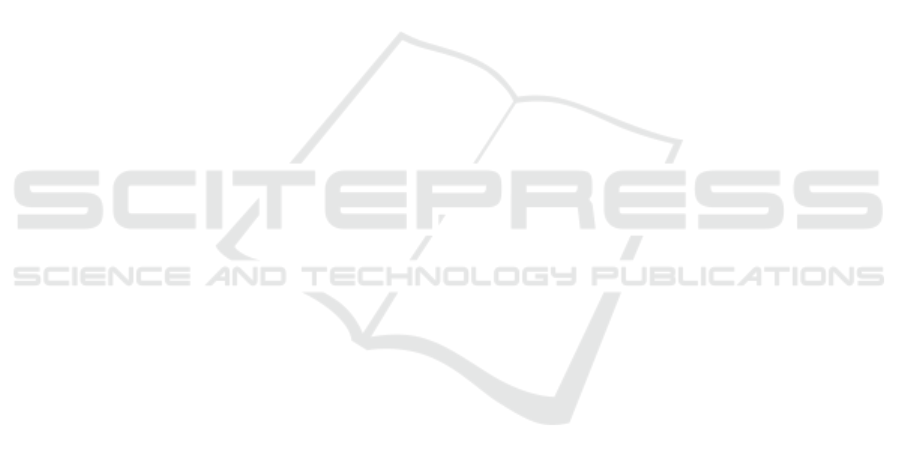
quences. The segmentation was carried out by con-
sidering only the information coming from the hands,
which makes our method applicable to all sign lan-
guages. We first used the hands motion calculated us-
ing body landmarks provided by OpenPose. Then we
corrected the segmentation using the hands shapes.
Once we got all signs isolated individually, we built
a probabilistic model using a portion of MOCAP
dataset which was annotated manually by an expert.
The model was used as a classifier to distinguish lexi-
cal and non lexical signs. To evaluate our algorithm of
classification we used the sensitivity, the specificity,
the precision and the F1 score metrics. The results
showed that our algorithm was capable of detecting
the lexical signs with a F1 score = 0.68 and that the
use of hands shapes for segmentation improved the
detection (F1 score improved by 0.13). To evaluate
the segmentation approach we calculate the average
difference between the beginning and end frames of
annotated and detected signs (3.8 frames). In the fu-
ture, we will try to refine both of the segmentation
and the classification results by including more fea-
tures that could be useful for the task. On a parallel
axis, we will use the set of the annotated features to
create sub-categories of signs based on the similarities
between features. Such categorisation would acceler-
ate the process of automatic recognition, make it more
efficient.
REFERENCES
Baltru
˘
saitis, T., Zadeh, A., Chong Lim, Y., and Morency,
L.-P. (2018). Openface 2.0: Facial behavior analysis
toolkit. IEEE International Conference on Automatic
Face and Gesture Recognition.
Cao, Z., Hidalgo, G., Simon, T., Wei, S.-E., and Sheikh,
Y. (2018). OpenPose: realtime multi-person 2D pose
estimation using Part Affinity Fields. arXiv preprint
arXiv:1812.08008.
Chaaban, H., Gouiff
`
es, M., and Braffort, A. (2019). To-
wards an Automatic Annotation of French Sign Lan-
guage Videos: Detection of Lexical Signs, pages 402–
412.
Cuxac, C. (2000). La langue des signes franc¸aise (lsf). les
voies de l’iconicit
´
e. Biblioth
`
eque de Faits de Langues,
(15-16). Paris: Ophrys.
Fatmi, R., Rashad, S., Integlia, R., and Hutchison, G.
(2017). American sign language recognition using
hidden markov models and wearable motion sensors.
Gonzalez Preciado, M. (2012). Computer Vision Methods
for Unconstrained Gesture Recognition in the Context
of Sign Language Annotation. Theses, Universit
´
e Paul
Sabatier - Toulouse III.
Kipp, M. (2001). Anvil - a generic annotation tool for mul-
timodal dialogue. INTERSPEECH.
Koller, O., Forster, J., and Ney, H. (2015). Continuous
sign language recognition: Towards large vocabulary
statistical recognition systems handling multiple sign-
ers. Computer Vision and Image Understanding 141,
pages 108–125.
Koller, O., Ney, H., and Bowden, R. (2016). Deep hand:
How to train a cnn on 1 million hand images when
your data is continuous and weakly labelled. In IEEE
Conference on Computer Vision and Pattern Recogni-
tion, pages 3793–3802, Las Vegas, NV, USA.
Lefebvre-Albaret, F. and Dalle, P. (2008). Une approche de
segmentation de la langue des signes franc¸aise.
Liang, Z.-j., Liao, S.-b., and Hu, B.-z. (2018). 3D Convolu-
tional Neural Networks for Dynamic Sign Language
Recognition. The Computer Journal, 61(11):1724–
1736.
Lim, K., Tan, A., Lee, C.-P., and Tan, S. (2019). Isolated
sign language recognition using convolutional neural
network hand modelling and hand energy image. Mul-
timedia Tools and Applications, 78.
Naert, L., Reverdy, C., Caroline, L., and Gibet, S. (2018).
Per channel automatic annotation of sign language
motion capture data. Workshop on the Representa-
tion and Processing of Sign Languages: Involving the
Language Community, LREC. Miyazaki Japan.
Pigou, L., Dieleman, S., Kindermans, P.-J., and Schrauwen,
B. (2015). Sign language recognition using convolu-
tional neural networks. volume 8925, pages 572–578.
Rao, G., Syamala, K., Kishore, P., and Sastry, A. (2018).
Deep convolutional neural networks for sign language
recognition. pages 194–197.
Rastgoo, R., Kiani, K., and Escalera, S. (2020). Video-
based isolated hand sign language recognition using a
deep cascaded model. Multimedia Tools and Applica-
tions.
Stokoe, W., Casterline, D., and Croneberg, C. (1976). A
dictionary of american sign language on linguistic
principles (revised ed.). [Silver Spring, Md.]: Linstok
Press.
Wang, H., Leu, M., and Oz, C. (2006). American sign
language recognition using multidimensional hidden
markov models. Journal of Information Science and
Engineering - JISE, 22:1109–1123.
Wittenburg, P., Levinson, S., Kita, S., and Brugman, H.
(2002). Multimodal annotations in gesture and sign
language studies. LREC.
Yang, R., Sarkar, S., Loeding, B., and Karshmer, A. (2006).
Efficient generation of large amounts of training data
for sign language recognition: A semi-automatic tool.
pages 635–642.
Automatic Annotation and Segmentation of Sign Language Videos: Base-level Features and Lexical Signs Classification
491