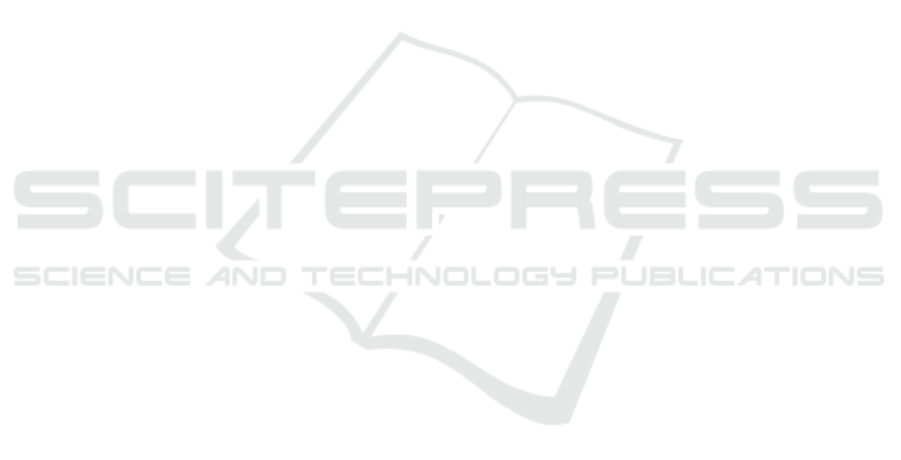
REFERENCES
Ajivar (2019). Life coach powered by artificial intelligence,
https://ajivar.com.
AlKandari, N. Y. (2020). Students anxiety experiences in
higher education institutions. In Anxiety Disorders.
IntechOpen.
Bagroy, S., Kumaraguru, P., and De Choudhury, M. (2017).
A social media based index of mental well-being in
college campuses. In Proceedings of the 2017 CHI
Conference on Human factors in Computing Systems,
pages 1634–1646.
Brooker, A., Brooker, S., and Lawrence, J. (2017). First
year students’ perceptions of their difficulties. Student
Success, 8(1):49–63.
Cer, D., Diab, M., Agirre, E., Lopez-Gazpio, I., and Spe-
cia, L. (2017). Semeval-2017 task 1: Semantic tex-
tual similarity-multilingual and cross-lingual focused
evaluation. arXiv preprint arXiv:1708.00055.
Chiesi, F. and Primi, C. (2009). Recency effects in primary-
age children and college students. International Elec-
tronic Journal of Mathematics Education, 4(3):259–
279.
Devlin, J., Chang, M.-W., Lee, K., and Toutanova, K.
(2018). Bert: Pre-training of deep bidirectional trans-
formers for language understanding. arXiv preprint
arXiv:1810.04805.
Doygun, O. and Gulec, S. (2012). The problems faced
by university students and proposals for solution.
Procedia-Social and Behavioral Sciences, 47:1115–
1123.
Gilbert, C. and Hutto, E. (2014). Vader: A parsimo-
nious rule-based model for sentiment analysis of so-
cial media text. In Eighth International Confer-
ence on Weblogs and Social Media (ICWSM-14).
Available at (20/04/16) http://comp. social. gatech.
edu/papers/icwsm14. vader. hutto. pdf, volume 81,
page 82.
Head, A. and Eisenberg, M. (2011). How college students
use the web to conduct everyday life research. First
Monday, 16(4).
Hochreiter, S. and Schmidhuber, J. (1997). Long short-term
memory. Neural computation, 9(8):1735–1780.
Jelodar, H., Wang, Y., Orji, R., and Huang, H. (2020). Deep
sentiment classification and topic discovery on novel
coronavirus or covid-19 online discussions: Nlp us-
ing lstm recurrent neural network approach. arXiv
preprint arXiv:2004.11695.
Kabir, M. and Madria, S. (2020). Coronavis: A
real-time covid-19 tweets analyzer. arXiv preprint
arXiv:2004.13932.
Kushin, M. J. and Yamamoto, M. (2010). Did social me-
dia really matter? college students’ use of online me-
dia and political decision making in the 2008 election.
Mass Communication and Society, 13(5):608–630.
Lai, G., Xie, Q., Liu, H., Yang, Y., and Hovy, E. (2017).
Race: Large-scale reading comprehension dataset
from examinations. arXiv preprint arXiv:1704.04683.
Li, B. and Han, L. (2013). Distance weighted cosine simi-
larity measure for text classification. In International
Conference on Intelligent Data Engineering and Au-
tomated Learning, pages 611–618. Springer.
Liu, Y., Ott, M., Goyal, N., Du, J., Joshi, M., Chen, D.,
Levy, O., Lewis, M., Zettlemoyer, L., and Stoyanov,
V. (2019). Roberta: A robustly optimized bert pre-
training approach. arXiv preprint arXiv:1907.11692.
Loper, E. and Bird, S. (2002). Nltk: the natural language
toolkit. arXiv preprint cs/0205028.
Loria, S. (2018). textblob documentation. Release 0.15, 2.
Oyebode, O., Ndulue, C., Adib, A., Mulchandani, D., Su-
ruliraj, B., Orji, F. A., Chambers, C., Meier, S., and
Orji, R. (2020). Health, psychosocial, and social is-
sues emanating from covid-19 pandemic based on so-
cial media comments using natural language process-
ing. arXiv preprint arXiv:2007.12144.
Paul, J. A., Baker, H. M., and Cochran, J. D. (2012).
Effect of online social networking on student aca-
demic performance. Computers in Human Behavior,
28(6):2117–2127.
Reimers, N. and Gurevych, I. (2019). Sentence-bert: Sen-
tence embeddings using siamese bert-networks. arXiv
preprint arXiv:1908.10084.
Schroff, F., Kalenichenko, D., and Philbin, J. (2015).
Facenet: A unified embedding for face recognition
and clustering. In Proceedings of the IEEE conference
on computer vision and pattern recognition, pages
815–823.
Sin, M., Pyeon, H., Kim, H., and Moon, J. (2019). Inter-
personal relationship, body image, academic achieve-
ment according to sns use time of college students.
The Journal of the Convergence on Culture Technol-
ogy, 5(1):257–264.
Thelwall, M., Buckley, K., and Paltoglou, G. (2012). Sen-
timent strength detection for the social web. Journal
of the American Society for Information Science and
Technology, 63(1):163–173.
Tsakalidis, A., Liakata, M., Damoulas, T., and Cristea, A. I.
(2018). Can we assess mental health through social
media and smart devices? addressing bias in method-
ology and evaluation. In Joint European Conference
on Machine Learning and Knowledge Discovery in
Databases, pages 407–423. Springer.
Vaswani, A., Shazeer, N., Parmar, N., Uszkoreit, J., Jones,
L., Gomez, A. N., Kaiser, Ł., and Polosukhin, I.
(2017). Attention is all you need. In Advances in
neural information processing systems, pages 5998–
6008.
Wang, A., Singh, A., Michael, J., Hill, F., Levy, O., and
Bowman, S. R. (2018). Glue: A multi-task bench-
mark and analysis platform for natural language un-
derstanding. arXiv preprint arXiv:1804.07461.
Williams, A., Nangia, N., and Bowman, S. R. (2017).
A broad-coverage challenge corpus for sentence
understanding through inference. arXiv preprint
arXiv:1704.05426.
Yang, C.-c. and Lee, Y. (2020). Interactants and activities
on facebook, instagram, and twitter: Associations be-
tween social media use and social adjustment to col-
lege. Applied Developmental Science, 24(1):62–78.
HEALTHINF 2021 - 14th International Conference on Health Informatics
466