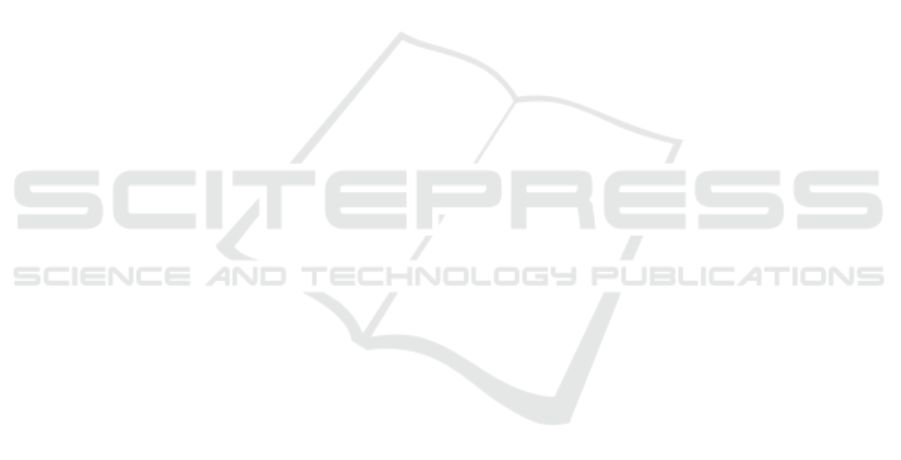
REFERENCES
(2018). Retinal image quality assessment using deep learn-
ing. Computers in Biology and Medicine, 103:64 –
70.
Aquino, E. M., Barreto, S. M., Bensenor, I. M., Car-
valho, M. S., Chor, D., Duncan, B. B., Lotufo, P. A.,
Mill, J. G., Molina, M. D. C., Mota, E. L., et al.
(2012). Brazilian longitudinal study of adult health
(elsa-brasil): objectives and design. American journal
of epidemiology, 175(4):315–324.
Breiman, L. (2001). Random forests. Machine learning,
45(1):5–32.
Costa, P., Campilho, A. J. C., Hooi, B., Smailagic, A., Ki-
tani, K., Liu, S., Faloutsos, C., and Galdran, A. In
ICMLA.
EyePACS (2015). Diabetic retinopathy detection of kaggle.
Available in: https://www. kaggle. com/c/diabetic-
retinopathy-detection/data.
Fasih, M., Langlois, J. P., Tahar, H. B., and Cheriet,
F. (2014). Retinal image quality assessment us-
ing generic features. In Medical Imaging 2014:
Computer-Aided Diagnosis, volume 9035, page
90352Z. International Society for Optics and Photon-
ics.
Florez, E., Nichols, T., Parker, E. E., Lirette, S. T., Howard,
C. M., and Fatemi, A. (2018). Multiparametric-mri
in the assessment of primary brain tumors through ra-
diomic features: a metric for guided radiation treat-
ment planning. Cureus, 10(10).
Fu, H., Wang, B., Shen, J., Cui, S., Xu, Y., Liu, J., and
Shao, L. (2019). Evaluation of retinal image quality
assessment networks in different color-spaces. CoRR,
abs/1907.05345.
Giancardo, L. (2011). Automated fundus images analysis
techniques to screen retinal diseases in diabetic pa-
tients. Theses, Universit
´
e de Bourgogne.
Grassmann, F., Mengelkamp, J., Brandl, C., Harsch, S.,
Zimmermann, M. E., Linkohr, B., Peters, A., Heid,
I. M., Palm, C., and Weber, B. H. (2018). A deep
learning algorithm for prediction of age-related eye
disease study severity scale for age-related macular
degeneration from color fundus photography. Oph-
thalmology, 125(9):1410–1420.
He, K., Zhang, X., Ren, S., and Sun, J. (2015). Deep
residual learning for image recognition. CoRR,
abs/1512.03385.
Jim
´
enez-Garc
´
ıa, J., Romero-Ora
´
a, R., Garc
´
ıa, M., L
´
opez-
G
´
alvez, M. I., and Hornero, R. (2019). Combination
of global features for the automatic quality assessment
of retinal images. Entropy, 21(3):311.
K
¨
ohler, T., Budai, A., Kraus, M. F., Odstr
ˇ
cilik, J., Michel-
son, G., and Hornegger, J. (2013). Automatic no-
reference quality assessment for retinal fundus images
using vessel segmentation. In Proc of the 26th IEEE
international symposium on computer-based medical
systems, pages 95–100. IEEE.
Lamiaa Abdel-Hamid, Ahmed El-Rafei, S. E.-R. G. M. and
Hornegger, J. (2016). Retinal image quality assess-
ment based on image clarity and content. Journal of
Biomedical Optics, 21(9):1 – 17 – 17.
Lin, J., Yu, L., Weng, Q., and Zheng, X. (2019). Reti-
nal image quality assessment for diabetic retinopathy
screening: A survey. Multimedia Tools and Applica-
tions.
Mariotti, A. and Pascolini, D. (2012). Global estimates of
visual impairment. Br J Ophthalmol, 96(5):614–8.
Narvekar, Niranjan D, K. L. J. (2009). A no-reference per-
ceptual image sharpness metric based on a cumulative
probability of blur detection. In International Work-
shop on Quality of Multimedia Experience, pages 87–
91. IEEE.
Nayak, J., Acharya, R., Bhat, P. S., S., N., and Lim,
T. (2009). Automated diagnosis of glaucoma using
digital fundus images. Journal of medical systems,
33(5):337.
Paulus, J., Meier, J., Bock, R., Hornegger, J., and Michel-
son, G. (2010). Automated quality assessment of reti-
nal fundus photos. International Journal of Computer
Assisted Radiology and Surgery, 5(6):557–564.
Pires, R., Jelinek, H. F., Wainer, J., and Rocha, A. (2012).
Retinal image quality analysis for automatic diabetic
retinopathy detection. In SIBGRAPI Conference on
Graphics, Patterns and Images, pages 229–236.
Raman, R., Srinivasan, S., Virmani, S., Sivaprasad, S., Rao,
C., and Rajalakshmi, R. (2018). Fundus photograph-
based deep learning algorithms in detecting diabetic
retinopathy. Eye.
Russakovsky, O., Deng, J., Su, H., Krause, J., Satheesh, S.,
Ma, S., Huang, Z., Karpathy, A., Khosla, A., Bern-
stein, M., Alexander, Berg, C., and Fei-Fei, L. (2015).
ImageNet Large Scale Visual Recognition Challenge.
IJCV, 115(3):211–252.
Saha, S., Fernando, B., Cuadros, J., Xiao, D., and Kana-
gasingam, Y. (2018). Automated quality assess-
ment of colour fundus images for diabetic retinopathy
screening in telemedicine. Journal of Digital Imaging,
31:869–878.
Salz, D. A. and Witkin, A. J. (2015). Imaging in diabetic
retinopathy. Middle East African journal of ophthal-
mology, 22(2):145.
Sevik, U., Kose, C., Berber, T., and Erdol, H. (2014). Iden-
tification of suitable fundus images using automated
quality assessment methods. Journal of Biomedical
Optics, 19(4):1 – 11.
Simonyan, K. and Zisserman, A. (2014). Very deep con-
volutional networks for large-scale image recognition.
arXiv preprint arXiv:1409.1556.
Szegedy, C., Ioffe, S., and Vanhoucke, V. (2016). Inception-
v4, inception-resnet and the impact of residual con-
nections on learning. CoRR, abs/1602.07261.
Szegedy, C., Vanhoucke, V., Ioffe, S., Shlens, J., and Wojna,
Z. (2015). Rethinking the inception architecture for
computer vision. CoRR, abs/1512.00567.
Wang, S., Jin, K., Lu, H., Cheng, C., Ye, J., and Qian, D.
(2015). Human visual system-based fundus image
quality assessment of portable fundus camera pho-
tographs. IEEE transactions on medical imaging,
35(4):1046–1055.
VISAPP 2021 - 16th International Conference on Computer Vision Theory and Applications
668