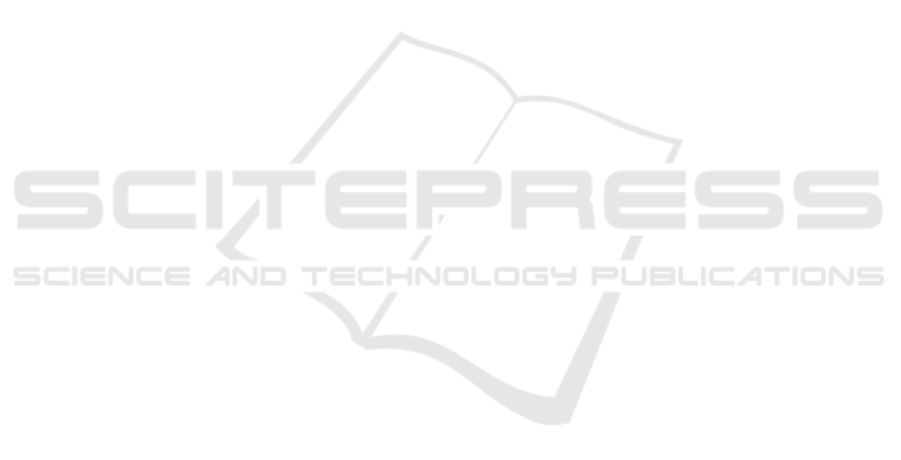
scene understanding. In Proc. of the IEEE Conference
on Computer Vision and Pattern Recognition (CVPR).
Deng, J., Dong, W., Socher, R., Li, L.-J., Li, K., and Fei-
Fei, L. (2009). ImageNet: A Large-Scale Hierarchical
Image Database. In CVPR09.
Dias Da Cruz, S., Wasenm
¨
uller, O., Beise, H., Stifter, T.,
and Stricker, D. (2020). Sviro: Synthetic vehicle in-
terior rear seat occupancy dataset and benchmark. In
IEEE Winter Conference on Applications of Computer
Vision (WACV).
Dundar, A., Liu, M., Wang, T., Zedlewski, J., and Kautz, J.
(2018). Domain stylization: A strong, simple baseline
for synthetic to real image domain adaptation. ArXiv,
abs/1807.09384.
Feld, H., Mirbach, B., Katrolia, J., Selim, M., Wasenm
¨
uller,
O., and Stricker, D. (2020). Dfki cabin simulator: A
test platform for visual in-cabin monitoring functions.
In Commercial Vehicle Technology 2020 - Proceed-
ings of the 6th Commercial Vehicle Technology Sym-
posium - CVT 2020.
Ghifary, M., Kleijn, B., and Zhang, M. (2014). Do-
main adaptive neural networks for object recogni-
tion. In Pham, D.-N. and Park, S.-B., editors, PRICAI
2014: Trends in Artificial Intelligence, pages 898–
904, Cham. Springer International Publishing.
Goodfellow, I., Pouget-Abadie, J., Mirza, M., Xu, B.,
Warde-Farley, D., Ozair, S., Courville, A., and Ben-
gio, Y. (2014). Generative adversarial nets. In Ghahra-
mani, Z., Welling, M., Cortes, C., Lawrence, N. D.,
and Weinberger, K. Q., editors, Advances in Neu-
ral Information Processing Systems 27, pages 2672–
2680. Curran Associates, Inc.
Gu, X., Guo, Y., Deligianni, F., and Yang, G. (2020). Cou-
pled real-synthetic domain adaptation for real-world
deep depth enhancement. IEEE Transactions on Im-
age Processing, 29:6343–6356.
He, K., Gkioxari, G., Doll
´
ar, P., and Girshick, R. (2017).
Mask r-cnn. In 2017 IEEE International Conference
on Computer Vision (ICCV), pages 2980–2988.
He, K., Zhang, X., Ren, S., and Sun, J. (2016). Deep resid-
ual learning for image recognition. In 2016 IEEE Con-
ference on Computer Vision and Pattern Recognition
(CVPR), pages 770–778.
He, W., Zhongzhao, X., Li, Y., Wang, X., and Cai, W.
(2019). Synthesizing depth hand images with gans
and style transfer for hand pose estimation. Sensors,
19:2919.
Hoffman, J., Tzeng, E., Darrell, T., and Saenko, K. (2017).
Simultaneous Deep Transfer Across Domains and
Tasks, pages 173–187.
Hoffman, J., Tzeng, E., Park, T., Zhu, J., Isola, P., Saenko,
K., Efros, A., and Darrell, T. (2018). Cycada: Cycle-
consistent adversarial domain adaptation. In Proceed-
ings of the 35th International Conference on Machine
Learning. PMLR 80:1989-1998.
Hu, J., Lu, J., and Tan, Y. (2015). Deep transfer met-
ric learning. In 2015 IEEE Conference on Computer
Vision and Pattern Recognition (CVPR), pages 325–
333.
Isola, P., Zhu, J., Zhou, T., and Efros, A. (2017). Image-
to-image translation with conditional adversarial net-
works. CVPR.
Karacan, L., Akata, Z., Erdem, A., and Erdem, E. (2016).
Learning to generate images of outdoor scenes from
attributes and semantic layouts.
Kingma, D. and Ba, J. (2015). Adam: A method for
stochastic optimization. CoRR, abs/1412.6980.
Li, Y., Liu, M., Li, X., Yang, M., and Kautz, J. (2018). A
closed-form solution to photorealistic image styliza-
tion. In ECCV.
Liu, M.-Y., Breuel, T., and Kautz, J. (2017). Unsuper-
vised image-to-image translation networks. ArXiv,
abs/1703.00848.
Motiian, S., Piccirilli, M., Adjeroh, D. A., and Doretto,
G. (2017). Unified deep supervised domain adapta-
tion and generalization. In 2017 IEEE International
Conference on Computer Vision (ICCV), pages 5716–
5726.
Mueller, F., Bernard, F., Sotnychenko, O., Mehta, D., Srid-
har, S., Casas, D., and Theobalt, C. (2018). Ganerated
hands for real-time 3d hand tracking from monocular
rgb. In 2018 IEEE/CVF Conference on Computer Vi-
sion and Pattern Recognition, pages 49–59.
Rambach, J., Deng, C., Pagani, A., and Stricker, D. (2018).
Learning 6dof object poses from synthetic single
channel images. In Proceedings of the 17th IEEE
ISMAR —. IEEE International Symposium on Mixed
and Augmented Reality (ISMAR-2018), 17th, October
16-20, M
¨
unchen, Germany. IEEE, IEEE.
Ren, S., He, K., Girshick, R., and Sun, J. (2015). Faster
r-cnn: Towards real-time object detection with region
proposal networks. In Proceedings of the 28th Inter-
national Conference on Neural Information Process-
ing Systems - Volume 1, NIPS’15, page 91–99, Cam-
bridge, MA, USA. MIT Press.
Richter, S. R., Vineet, V., Roth, S., and Koltun, V. (2016).
Playing for data: Ground truth from computer games.
In Leibe, B., Matas, J., Sebe, N., and Welling,
M., editors, European Conference on Computer Vi-
sion (ECCV), volume 9906 of LNCS, pages 102–118.
Springer International Publishing.
Shrivastava, A., Pfister, T., Tuzel, O., Susskind, J., Wang,
W., and Webb, R. (2017). Learning from simulated
and unsupervised images through adversarial training.
2017 IEEE Conference on Computer Vision and Pat-
tern Recognition (CVPR), pages 2242–2251.
Simonyan, K. and Zisserman, A. (2015). Very deep con-
volutional networks for large-scale image recognition.
In International Conference on Learning Representa-
tions.
Song, S., Lichtenberg, S. P., and Xiao, J. (2015). Sun rgb-
d: A rgb-d scene understanding benchmark suite. In
2015 IEEE Conference on Computer Vision and Pat-
tern Recognition (CVPR), pages 567–576.
Toldo, M., Michieli, U., Agresti, G., and Zanuttigh, P.
(2020). Unsupervised domain adaptation for mobile
semantic segmentation based on cycle consistency and
feature alignment. Image Vision Comput., 95(C).
Tzeng, E., Hoffman, J., Zhang, N., Saenko, K., and Darrell,
T. (2014). Deep domain confusion: Maximizing for
domain invariance. ArXiv, abs/1412.3474.
Zhu, J., Park, T., Isola, P., and Efros, A. (2017). Unpaired
image-to-image translation using cycle-consistent ad-
versarial networks. pages 2242–2251.
An Adversarial Training based Framework for Depth Domain Adaptation
361