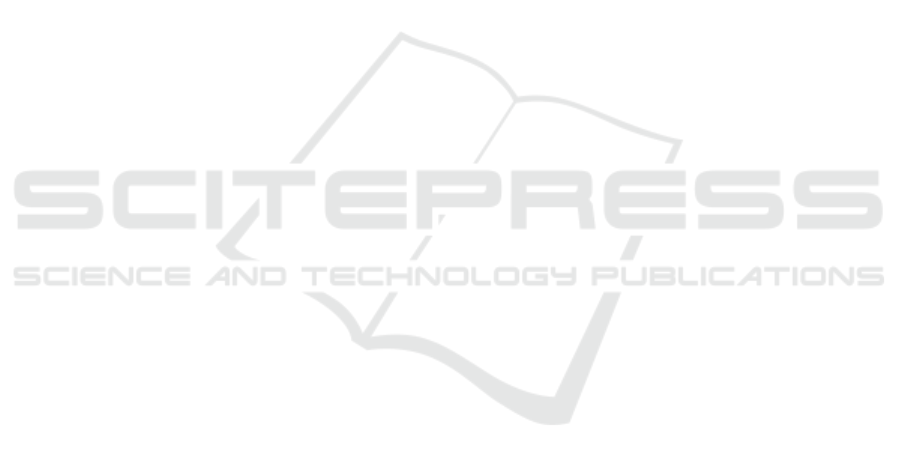
(Aronson, 1985), (Beretta and Capasso, 1986). The
family of SIR models with various generalizations of
the status elements S (susceptible), I (infected or in-
fectious) and R (removed or recovered) with respect
to other status elements as E (exposed) and subpopu-
lations use ordinary differential equations, cf. (Ahmed
and Agiza, 1998), (Andersen and May, 1979), (Capas-
so, 1993), (Redheffer and Walter, 1984).
The variables are fractions of the population mo-
delled as positive real numbers as in the mass acti-
on law supposing homogeneous distributions. Peri-
odic solutions of first order ordinary differential equa-
tions are unstable with respect to system parameters.
A large number of SIR models use bilinear differenti-
al equations, cf.(R
¨
udiger et al., 2020).
We propose a model which could be presented as
a cellular automata. In order to use methods of par-
tial differential equations and mean value operators
in differential geometry we use differential manifolds
as a general modelling background and its discreti-
zation which generalizes the Euclidean structure of
cellular automata, cf. (Mikeler et al., 2005), (G
¨
unther
and Pr
¨
ufer, 1999), (Schuster, 1988), (Schuster, 1997),
(Wang et al., 2015), (Welch et al., 2011).
An essential feature will be the visualization of de-
velopment of the pandemic with special structures, cf.
(Murray, 2002), (Murray, 2003), (Barrat and Vespig-
nani, 2008), (Andersen and May, 1979). This will be
realized with Mathematica from Wolfram Research
which also allows to use a syntax without coordinates.
A visualization of the pandemic status of 40 thousand
individual can be reached with some hours of calcula-
tion time. The results to be considered in the followi-
ng have proven to be the optimal solution within the
used simulations with regard to the target variable that
is still to be discussed.
If one would change the discretization of the torus
structure of the used differential manifold by a graph
structure (cf. (Solimano and Bretta, 1982), (R
¨
udiger
et al., 2020), (Welch et al., 2011), (Boguna et al.,
2003)) much less individuals could be visualized.
Cyclical structures in the sense of a boundary cy-
cle or a cyclical band were discussed in chemical
and biological examples, where there are fundamen-
tal theoretical differences, but also commonalities bet-
ween discrete and continuous systems, cf. (Capas-
so and Maddalena, 1983), (Field and Burger, 1985).
When modeling exponential growth, there are not on-
ly differences between continuous and discrete sy-
stems, but also between spatially bounded and un-
bounded systems (compact and non-compact mani-
folds in differential geometry), cf. (Murray, 2002),
(Murray, 2003), (Barrat and Vespignani, 2008), (Be-
retta and Capasso, 1986), (Boguna et al., 2003), (So-
limano and Bretta, 1982). In this context, the r-value
plays a role in the current Covid-19 discussion that
has already been analyzed earlier in various contexts,
cf. (Dieckman et al., 1990). The r-value should de-
scribe the prevailing value of an exponential spread.
The exponential mapping provides the crucial relati-
onship between Lie algebras and Lie groups in diffe-
rential geometry. This goes far beyond the exponen-
tial function on real numbers, which already shows a
completely different qualitative behavior on complex
numbers. Using the exponential function to describe
the spread of Covid-19, which could practically ne-
ver be sensibly modeled with an exponential spread,
leads to theoretical and practical problems. The expo-
nential map on a discretized torus leads to a growth in
which the saturation value has to be estimated, which
is in principle impossible from short initial data. The
actual paper is a first step in the stated context.
2 MATERIAL AND METHODS
Individuals are the elements of an n by m array (in the
examples we use the special values n = m = 200). In-
dividuals can be in the status S (susceptible: healthy
without immune behavior), I
1
or I
2
(infected or infec-
tious after incubation period) and R
1
or R
2
(recovered
with immune behavior) for each day after initializa-
tion. Further on for every individual in the I
1
, I
2
, R
1
or R
2
status the number of days being in that status is
considered, cf. Figure 1. It is also possible to model
different immune behavior specifically related to the
infectious disease in different S compartments, here
this is be done in the R compartments.
The right and left hand as well as the upper and
lower elements of the array resulting from the dis-
cretization of the torus are connected. If one would
change the upper and lower side of the right and left
sides of the array and respectively for the upper and
lower side we would get the discretization of double
M
¨
obius strip which can’t be realized in three dimen-
sional geometry. With an other boundary identificati-
on one also could reach the topology of a sphere.
As a local neighborhood of the array element (i, j)
we consider all elements (i + k, j + k) mod n with
−m ≤ k ≤ m (m = 5 in the examples). An S indivi-
dul in the local neighborhood of a I individual is in-
fected randomly with equal probability with the pro-
perty that during the infectious period r individuals (r
as the theoretical reproduction number) are infected.
This theoretical reproduction number supposes that
all other individuals in the local neighborhood are in
the S status.
The individuals of the I
2
compartment are less in-
HEALTHINF 2021 - 14th International Conference on Health Informatics
476