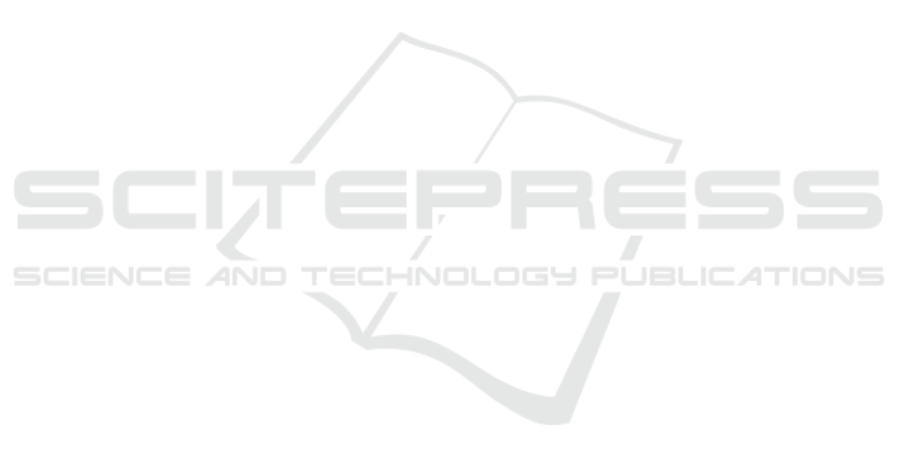
ERATOR (ref. 04/SI/2019) and PREVOCU-
PAI (DSAIPA/AI/0105/2019), and Ph.D. grant
PD/BDE/142816/2018.
REFERENCES
Barandas, M., Folgado, D., Fernandes, L., Santos, S.,
Abreu, M., Bota, P., Liu, H., Schultz, T., and Gam-
boa, H. (2020). TSFEL: Time series feature extraction
library. SoftwareX, 11:100456.
Bauters, K., Cottyn, J., Claeys, D., Slembrouck, M., Vee-
laert, P., and van Landeghem, H. (2018). Auto-
mated work cycle classification and performance mea-
surement for manual work stations. Robotics and
Computer-Integrated Manufacturing, 51:139–157.
Bauters, K., Van Landeghem, H., Slembrouck, M., Van
Cauwelaert, D., and Van Haerenborgh, D. (2014). An
automated work cycle classification and disturbance
detection tool for assembly line work stations. In
ICINCO 2014 - Proceedings of the 11th International
Conference on Informatics in Control, Automation
and Robotics, volume 2, pages 685–691. SciTePress.
Bello, J. P., Grosche, P., M
¨
uller, M., and Weiss, R. J. (2018).
Content-based methods for knowledge discovery in
music. In Bader, R., editor, Springer Handbook
on Systematic Musicology, pages 823–840. Springer,
Berlin, Heidelberg.
Bota, P., Silva, J., Folgado, D., and Gamboa, H. (2019). A
semi-automatic annotation approach for human activ-
ity recognition. Sensors, 19:501.
Cutler, R. and Davis, L. (1999). Real-time periodic motion
detection, analysis, and applications. Proceedings of
the IEEE Computer Society Conference on Computer
Vision and Pattern Recognition, 2:326–332.
Cutler, R. and Davis, L. (2002). View-based detection and
analysis of periodic motion. In Proceedings. Four-
teenth International Conference on Pattern Recogni-
tion, pages 495–500. Institute of Electrical and Elec-
tronics Engineers (IEEE). Cutler, R. and Davis, L. S.
(2000). Robust real-time periodic motion detection,
analysis, and applications. IEEE Transactions on Pat-
tern Analysis and Machine Intelligence, 22(8):781–
796.
Foote, J. (2000). Automatic audio segmentation using a
measure of audio novelty. In IEEE International Con-
ference on Multi-Media and Expo, pages 452–455.
Irastorza, E. S., Xabier, and Copsey, S. (2010). OSH in
figures: Work-related musculoskeletal disorders in the
EU — Facts and figures. European Agency for Safety
and Health at Work.
Jatob
´
a, L. C., Großmann, U., Kunze, C., Ottenbacher, J.,
and Stork, W. (2008). Context-aware mobile health
monitoring: Evaluation of different pattern recogni-
tion methods for classification of physical activity. In
Proceedings of the 30th Annual International Con-
ference of the IEEE Engineering in Medicine and
Biology Society, EMBS’08 - ”Personalized Health-
care through Technology”, volume 2008, pages 5250–
5253. IEEE Computer Society.
Lara,
´
O. D. and Labrador, M. A. (2013). A survey on human
activity recognition using wearable sensors. IEEE
Communications Surveys and Tutorials, 15(3):1192–
1209.
Law, S. M. (2019). STUMPY: A Powerful and Scalable
Python Library for Time Series Data Mining. The
Journal of Open Source Software, 4(39):1504.
Lu, C. M. and Ferrier, N. J. (2003). Automated Analysis of
Repetitive Joint Motion. IEEE Transactions on Infor-
mation Technology in Biomedicine, 7(4):263–273.
Lu, C. M. and Ferrier, N. J. (2004). Repetitive Motion Anal-
ysis: Segmentation and Event Classification. IEEE
Transactions on Pattern Analysis and Machine Intel-
ligence, 26(2):258–263.
Machado, I. P., Lu
´
ısa Gomes, A., Gamboa, H., Paix
˜
ao,
V., and Costa, R. M. (2015). Human activity data
discovery from triaxial accelerometer sensor: Non-
supervised learning sensitivity to feature extraction
parametrization. Information Processing & Manage-
ment, 51(2):204 – 214.
Nunes, N., Ara
´
ujo, T., and Gamboa, H. (2011). Two-modes
cyclic biosignal clustering based on time series anal-
ysis. In Proceedings of the International Conference
on Bio-inspired Systems and Signal Processing, pages
257–264.
Paulus, J., M
¨
uller, M., and Klapuri, A. (2010). Audio-based
music structure analysis. In Proceedings of the Inter-
national Conference on Music Information Retrieval
(ISMIR), pages 625–636, Utrecht, The Netherlands.
Rodrigues, J., Belo, D., and Gamboa, H. (2017). Noise de-
tection on ecg based on agglomerative clustering of
morphological features. Computers in Biology and
Medicine, 87:322 – 334.
Rodrigues, J., Gamboa, H., Mollaei, N., Os
´
orio, D.,
Assunc¸
˜
ao, A., Fuj
˜
ao, C., and Carnide, F. (2020).
A genetic algorithm to design job rotation sched-
ules with low risk exposure. In Camarinha-Matos,
L. M., Farhadi, N., Lopes, F., and Pereira, H., editors,
Technological Innovation for Life Improvement, pages
395–402, Cham. Springer International Publishing.
Romero, D., Stahre, J., Wuest, T., Noran, O., Bernus, P.,
Fasth, Fast-Berglund, A., and Gorecky, D. (2016). To-
wards an Operator 4.0 Typology: A Human-Centric
Perspective on the Fourth Industrial Revolution Tech-
nologies. In International conference on computers
and industrial engineering (CIE46) proceedings.
Santos, S., Folgado, D., Rodrigues, J., Mollaei, N., Fuj
˜
ao,
C., and Gamboa, H. (2020). Explaining the ergonomic
assessment of human movement in industrial contexts.
In Proceedings of the 13th International Joint Confer-
ence on Biomedical Engineering Systems and Tech-
nologies - Volume 4: BIOSIGNALS,, pages 79–88. IN-
STICC, SciTePress.
Varandas., R., Folgado., D., and Gamboa., H. (2019).
Evaluation of spatial-temporal anomalies in the anal-
ysis of human movement. In Proceedings of the
12th International Joint Conference on Biomedical
Engineering Systems and Technologies - Volume 4
BIOSIGNALS: BIOSIGNALS,, pages 163–170. IN-
STICC, SciTePress.
Self-Similarity Matrix of Morphological Features for Motion Data Analysis in Manufacturing Scenarios
89