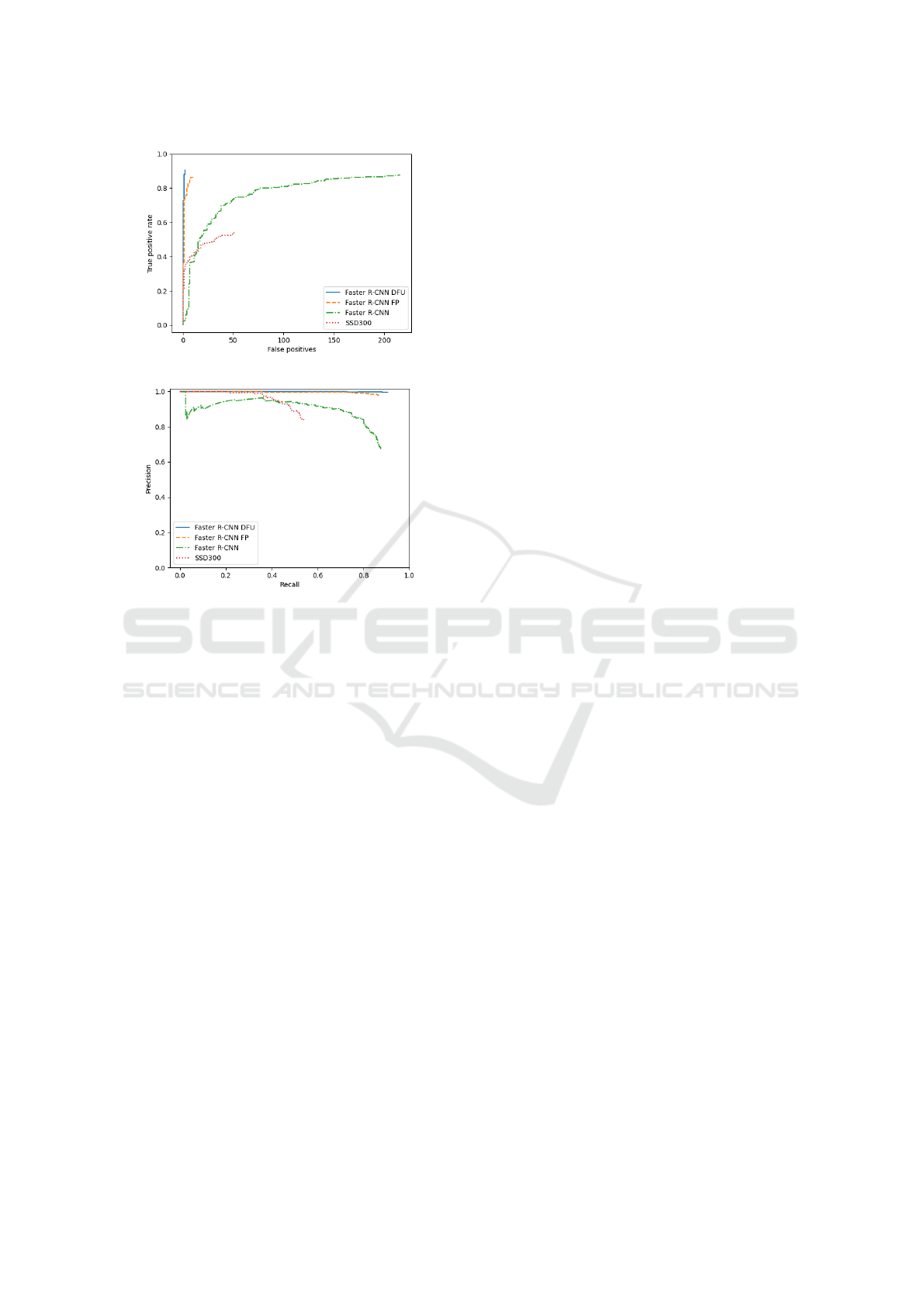
(a)
(b)
Figure 4: Comparisons of ROC curves for different experi-
mental settings for DFU detection.
REFERENCES
Abadi, M., Barham, P., Chen, J., Chen, Z., Davis, A.,
Dean, J., Devin, M., Ghemawat, S., Irving, G., Isard,
M., et al. (2016). Tensorflow: A system for large-
scale machine learning. In 12th {USENIX} sympo-
sium on operating systems design and implementation
({OSDI} 16), pages 265–283.
Armstrong, D. G., Boulton, A. J., and Bus, S. A. (2017). Di-
abetic foot ulcers and their recurrence. New England
Journal of Medicine, 376(24):2367–2375.
Bankman, I. (2008). Handbook of medical image process-
ing and analysis. Elsevier.
Cassidy, B., Reeves, N. D., Joseph, P., Gillespie, D.,
O’Shea, C., Rajbhandari, S., Maiya, A. G., Frank, E.,
Boulton, A., Armstrong, D., et al. (2020). Dfuc2020:
Analysis towards diabetic foot ulcer detection. arXiv
preprint arXiv:2004.11853.
Dai, J., Li, Y., He, K., and Sun, J. (2016). R-fcn: Object de-
tection via region-based fully convolutional networks.
In Advances in neural information processing sys-
tems, pages 379–387.
Everingham, M., Eslami, S. A., Van Gool, L., Williams,
C. K., Winn, J., and Zisserman, A. (2015). The pascal
visual object classes challenge: A retrospective. Inter-
national journal of computer vision, 111(1):98–136.
Everingham, M., Van Gool, L., Williams, C. K., Winn, J.,
and Zisserman, A. (2007). The pascal visual object
classes challenge 2007 (voc2007) results.
Fan, Q., Brown, L., and Smith, J. (2016). A closer look
at faster r-cnn for vehicle detection. In 2016 IEEE
intelligent vehicles symposium (IV), pages 124–129.
IEEE.
Girshick, R. (2015). Fast R-CNN. In Proceedings of the
IEEE international conference on computer vision,
pages 1440–1448.
Goyal, M., Reeves, N. D., Davison, A. K., Rajbhandari, S.,
Spragg, J., and Yap, M. H. (2018a). Dfunet: Convo-
lutional neural networks for diabetic foot ulcer clas-
sification. IEEE Transactions on Emerging Topics in
Computational Intelligence.
Goyal, M., Reeves, N. D., Rajbhandari, S., and Yap, M. H.
(2018b). Robust methods for real-time diabetic foot
ulcer detection and localization on mobile devices.
IEEE journal of biomedical and health informatics,
23(4):1730–1741.
Goyal, M., Yap, M. H., Reeves, N. D., Rajbhandari, S., and
Spragg, J. (2017). Fully convolutional networks for
diabetic foot ulcer segmentation. In 2017 IEEE inter-
national conference on systems, man, and cybernetics
(SMC), pages 618–623. IEEE.
He, K., Zhang, X., Ren, S., and Sun, J. (2016). Deep resid-
ual learning for image recognition. In Proceedings of
the IEEE conference on computer vision and pattern
recognition, pages 770–778.
Huang, J., Rathod, V., Sun, C., Zhu, M., Korattikara, A.,
Fathi, A., Fischer, I., Wojna, Z., Song, Y., Guadar-
rama, S., et al. (2017). Speed/accuracy trade-offs for
modern convolutional object detectors. In Proceed-
ings of the IEEE conference on computer vision and
pattern recognition, pages 7310–7311.
Ioffe, S. and Szegedy, C. (2015). Batch normalization: Ac-
celerating deep network training by reducing internal
covariate shift. arXiv preprint arXiv:1502.03167.
Lemley, J., Bazrafkan, S., and Corcoran, P. (2017). Smart
augmentation learning an optimal data augmentation
strategy. Ieee Access, 5:5858–5869.
Leung, P. (2007). Diabetic foot ulcers—a comprehensive
review. The Surgeon, 5(4):219–231.
Litjens, G., Kooi, T., Bejnordi, B. E., Setio, A. A. A.,
Ciompi, F., Ghafoorian, M., Van Der Laak, J. A.,
Van Ginneken, B., and S
´
anchez, C. I. (2017). A survey
on deep learning in medical image analysis. Medical
image analysis, 42:60–88.
Liu, C., van Netten, J. J., Van Baal, J. G., Bus, S. A., and
van Der Heijden, F. (2015). Automatic detection of di-
abetic foot complications with infrared thermography
by asymmetric analysis. Journal of biomedical optics,
20(2):026003.
Liu, W., Anguelov, D., Erhan, D., Szegedy, C., Reed, S.,
Fu, C.-Y., and Berg, A. C. (2016). Ssd: Single shot
multibox detector. In European conference on com-
puter vision, pages 21–37. Springer.
Pan, S. J. and Yang, Q. (2009). A survey on transfer learn-
ing. IEEE Transactions on knowledge and data engi-
neering, 22(10):1345–1359.
Faster R-CNN Approach for Diabetic Foot Ulcer Detection
683