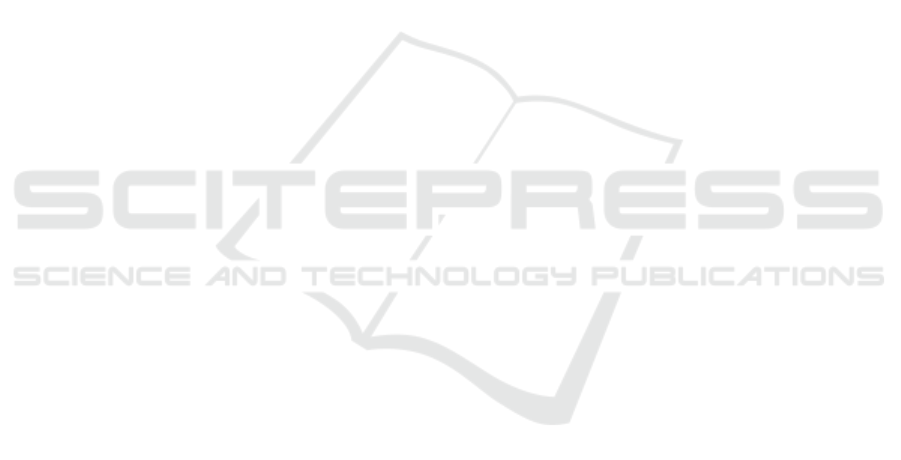
REFERENCES
Arani, E., Marzban, S., Pata, A., and Zonooz, B. (2021).
Rgpnet: A real-time general purpose semantic seg-
mentation. In Proceedings of the IEEE/CVF Win-
ter Conference on Applications of Computer Vision
(WACV). 4
Chawla, H., Jukola, M., Arani, E., and Zonooz, B. (2020a).
Monocular vision based crowdsourced 3d traffic sign
positioning with unknown camera intrinsics and dis-
tortion coefficients. In 2020 IEEE 23rd Interna-
tional Conference on Intelligent Transportation Sys-
tems (ITSC). IEEE. 2, 6, 7, 8, 9, 11
Chawla, H., Jukola, M., Brouns, T., Arani, E., and Zonooz,
B. (2020b). Crowdsourced 3d mapping: A combined
multi-view geometry and self-supervised learning ap-
proach. In 2020 IEEE/RSJ International Conference
on Intelligent Robots and Systems (IROS). IEEE. 2, 6
Che, Z., Li, G., Li, T., Jiang, B., Shi, X., Zhang, X., Lu, Y.,
Wu, G., Liu, Y., and Ye, J. (2019). D
2
-city: A large-
scale dashcam video dataset of diverse traffic scenar-
ios. arXiv preprint arXiv:1904.01975. 2, 6
Dabeer, O., Ding, W., Gowaiker, R., Grzechnik, S. K., Lak-
shman, M. J., Lee, S., Reitmayr, G., Sharma, A.,
Somasundaram, K., Sukhavasi, R. T., et al. (2017).
An end-to-end system for crowdsourced 3d maps for
autonomous vehicles: The mapping component. In
2017 IEEE/RSJ International Conference on Intelli-
gent Robots and Systems (IROS), pages 634–641. 1
de Agapito, L., Hayman, E., and Reid, I. D. (1998). Self-
calibration of a rotating camera with varying intrin-
sic parameters. In British Machine Vision Conference
(BMVC), pages 1–10. Citeseer. 2
Eigen, D. and Fergus, R. (2015). Predicting depth, surface
normals and semantic labels with a common multi-
scale convolutional architecture. In Proceedings of the
IEEE International Conference on Computer Vision
(ICCV), pages 2650–2658. 2, 8
Geiger, A., Lenz, P., and Urtasun, R. (2012). Are we ready
for autonomous driving? the kitti vision benchmark
suite. In 2012 IEEE Conference on Computer Vision
and Pattern Recognition (CVPR), pages 3354–3361.
2, 6
Gherardi, R. and Fusiello, A. (2010). Practical autocali-
bration. In European Conference on Computer Vision
(ECCV), pages 790–801. Springer Berlin Heidelberg.
1, 2
Godard, C., Mac Aodha, O., Firman, M., and Brostow, G. J.
(2019). Digging into self-supervised monocular depth
estimation. In Proceedings of the IEEE International
Conference on Computer Vision (ICCV), pages 3828–
3838. 1, 8
Gordon, A., Li, H., Jonschkowski, R., and Angelova, A.
(2019). Depth from videos in the wild: Unsupervised
monocular depth learning from unknown cameras. In
Proceedings of the IEEE International Conference on
Computer Vision (ICCV), pages 8977–8986. 1, 2, 5, 6
Hartley, R. and Zisserman, A. (2003). Multiple view geom-
etry in computer vision. Cambridge university press.
3, 7
Kukelova, Z., Heller, J., Bujnak, M., Fitzgibbon, A., and
Pajdla, T. (2015). Efficient solution to the epipolar
geometry for radially distorted cameras. In 2015 IEEE
International Conference on Computer Vision (ICCV),
pages 2309–2317. 1, 2
Lopez, M., Mari, R., Gargallo, P., Kuang, Y., Gonzalez-
Jimenez, J., and Haro, G. (2019). Deep single image
camera calibration with radial distortion. In Proceed-
ings of the IEEE Conference on Computer Vision and
Pattern Recognition (CVPR), pages 11817–11825. 2
Maddern, W., Pascoe, G., Linegar, C., and Newman, P.
(2017). 1 year, 1000 km: The oxford robotcar
dataset. The International Journal of Robotics Re-
search, 36(1):3–15. 2, 6
Meister, S., Hur, J., and Roth, S. (2018). Unflow: Unsu-
pervised learning of optical flow with a bidirectional
census loss. In Thirty-Second AAAI Conference on
Artificial Intelligence. 1
Mur-Artal, R., Montiel, J. M. M., and Tard
´
os, J. D. (2015).
Orb-slam: A versatile and accurate monocular slam
system. IEEE Transactions on Robotics, 31(5):1147–
1163. 1
Neuhold, G., Ollmann, T., Bul
`
o, S. R., and Kontschieder,
P. (2017). The mapillary vistas dataset for semantic
understanding of street scenes. In 2017 IEEE Interna-
tional Conference on Computer Vision (ICCV), pages
5000–5009. 4
Santana-Cedr
´
es, D., Gomez, L., Alem
´
an-Flores, M., Sal-
gado, A., Esclar
´
ın, J., Mazorra, L., and Alvarez, L.
(2017). Automatic correction of perspective and op-
tical distortions. Computer Vision and Image Under-
standing, 161:1–10. 6, 7, 11
Schonberger, J. L. and Frahm, J.-M. (2016). Structure-
from-motion revisited. In Proceedings of the IEEE
Conference on Computer Vision and Pattern Recogni-
tion (CVPR), pages 4104–4113. 2, 4, 7
Steger, C. (2012). Estimating the fundamental matrix under
pure translation and radial distortion. ISPRS journal
of photogrammetry and remote sensing, 74:202–217.
1, 3
Tummala, G. K., Das, T., Sinha, P., and Ramnath, R.
(2019). Smartdashcam: automatic live calibration for
dashcams. In Proceedings of the 18th International
Conference on Information Processing in Sensor Net-
works (IPSN), pages 157–168. 2
Wang, Z., Bovik, A. C., Sheikh, H. R., and Simoncelli, E. P.
(2004). Image quality assessment: from error visi-
bility to structural similarity. IEEE transactions on
image processing, 13(4):600–612. 7
Wildenauer, H. and Micusik, B. (2013). Closed form solu-
tion for radial distortion estimation from a single van-
ishing point. In British Machine Vision Conference
(BMVC). 1
Wu, C. (2014). Critical configurations for radial distortion
self-calibration. In 2014 IEEE Conference on Com-
puter Vision and Pattern Recognition (CVPR), pages
25–32. 1, 2, 3
Ying, Z. and Li, G. (2016). Robust lane marking detection
using boundary-based inverse perspective mapping.
In 2016 IEEE International Conference on Acoustics,
VISAPP 2021 - 16th International Conference on Computer Vision Theory and Applications
878