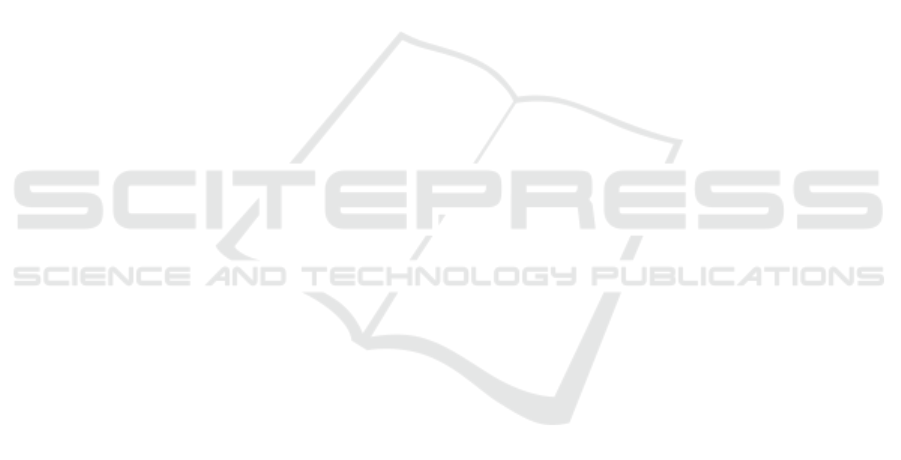
tion procedure significantly by excluding the machine
translation.
ACKNOWLEDGEMENTS
This work is funded by the German Research Foun-
dation (DFG) (grant RA 497/22-1, ”ELISA - Evolu-
tion of Semantic Annotations”). Computations for
this work were done using resources of the Leipzig
University Computing Centre.
REFERENCES
Afzal, Z., Akhondi, S. A., van Haagen, H., van Mulligen,
E. M., and Kors, J. A. (2015). Biomedical concept
recognition in French text using automatic translation
of English terms. In CLEF (Working Notes).
Aronson, A. R. and Lang, F.-M. (2010). An overview of
MetaMap: historical perspective and recent advances.
Journal of the American Medical Informatics Associ-
ation, 17(3):229–236.
Bowman, S. R., Angeli, G., Potts, C., and Manning, C. D.
(2015). A large annotated corpus for learning natural
language inference. arXiv preprint arXiv:1508.05326.
Bucilu
ˇ
a, C., Caruana, R., and Niculescu-Mizil, A. (2006).
Model compression. In Proceedings of the 12th
ACM SIGKDD International Conference on Knowl-
edge Discovery and Data Mining, pages 535–541.
Cabot, C., Soualmia, L. F., Dahamna, B., and Darmoni, S. J.
(2016). SIBM at CLEF eHealth Evaluation Lab 2016:
Extracting concepts in French medical texts with ecmt
and cimind. In CLEF (Working Notes), pages 47–60.
Cer, D., Diab, M., Agirre, E., Lopez-Gazpio, I., and Spe-
cia, L. (2017). Semeval-2017 task 1: Semantic tex-
tual similarity-multilingual and cross-lingual focused
evaluation. arXiv preprint arXiv:1708.00055.
Cer, D., Yang, Y., Kong, S.-y., Hua, N., Limtiaco, N., John,
R. S., Constant, N., Guajardo-Cespedes, M., Yuan,
S., Tar, C., et al. (2018). Universal sentence encoder.
arXiv preprint arXiv:1803.11175.
Christen, V., Groß, A., and Rahm, E. (2016). A reuse-based
annotation approach for medical documents. In Inter-
national Semantic Web Conference, pages 135–150.
Springer.
Christen, V., Groß, A., Varghese, J., Dugas, M., and Rahm,
E. (2015). Annotating medical forms using UMLS. In
International Conference on Data Integration in the
Life Sciences, pages 55–69. Springer.
Conneau, A., Khandelwal, K., Goyal, N., Chaudhary, V.,
Wenzek, G., Guzm
´
an, F., Grave, E., Ott, M., Zettle-
moyer, L., and Stoyanov, V. (2019). Unsupervised
cross-lingual representation learning at scale. arXiv
preprint arXiv:1911.02116.
Conneau, A., Kiela, D., Schwenk, H., Barrault, L., and Bor-
des, A. (2017). Supervised learning of universal sen-
tence representations from natural language inference
data. arXiv preprint arXiv:1705.02364.
Devlin, J., Chang, M.-W., Lee, K., and Toutanova, K.
(2018). BERT: Pre-training of deep bidirectional
transformers for language understanding. arXiv
preprint arXiv:1810.04805.
FAD (2017). Stady data standards: What you need to know.
https://www.fda.gov/media/98907/download.
Garc
´
ıa-Campayo, J., Zamorano, E., Ruiz, M. A., Pardo, A.,
P
´
erez-P
´
aramo, M., L
´
opez-G
´
omez, V., Freire, O., and
Rejas, J. (2010). Cultural adaptation into Spanish of
the generalized anxiety disorder-7 (GAD-7) scale as a
screening tool. Health and Quality of Life Outcomes,
8(1):8.
Hinton, G., Vinyals, O., and Dean, J. (2015). Distilling
the knowledge in a neural network. arXiv preprint
arXiv:1503.02531.
Kors, J. A., Clematide, S., Akhondi, S. A., Van Mulligen,
E. M., and Rebholz-Schuhmann, D. (2015). A mul-
tilingual gold-standard corpus for biomedical concept
recognition: the Mantra GSC. Journal of the Ameri-
can Medical Informatics Association, 22(5):948–956.
Lample, G. and Conneau, A. (2019). Cross-lingual
language model pretraining. arXiv preprint
arXiv:1901.07291.
Lan, Z., Chen, M., Goodman, S., Gimpel, K., Sharma, P.,
and Soricut, R. (2019). ALBERT: A lite BERT for
self-supervised learning of language representations.
arXiv preprint arXiv:1909.11942.
Lin, Y.-C., Christen, V., Groß, A., Cardoso, S. D., Pruski,
C., Da Silveira, M., and Rahm, E. (2017). Evaluat-
ing and improving annotation tools for medical forms.
In Proc. Data Integration in the Life Science (DILS
2017), pages 1–16. Springer.
Lin, Y.-C., Christen, V., Groß, A., Kirsten, T., Cardoso,
S. D., Pruski, C., Da Silveira, M., and Rahm, E.
(2020). Evaluating cross-lingual semantic annotation
for medical forms. In Proceedings of the 13th Inter-
national Joint Conference on Biomedical Engineering
Systems and Technologies - Volume 5 HEALTHINF,
pages 145–155.
Liu, Y., Ott, M., Goyal, N., Du, J., Joshi, M., Chen,
D., Levy, O., Lewis, M., Zettlemoyer, L., and Stoy-
anov, V. (2019). RoBERTa: A Robustly Opti-
mized BERT Pretraining Approach. arXiv preprint
arXiv:1907.11692.
Loeffler, M., Engel, C., Ahnert, P., Alfermann, D., Are-
lin, K., Baber, R., Beutner, F., Binder, H., Br
¨
ahler,
E., Burkhardt, R., et al. (2015). The LIFE-Adult-
Study: objectives and design of a population-based
cohort study with 10,000 deeply phenotyped adults in
Germany. BMC Public Health, 15(1):691.
L
¨
owe, B., Decker, O., M
¨
uller, S., Br
¨
ahler, E., Schellberg,
D., Herzog, W., and Herzberg, P. Y. (2008). Valida-
tion and standardization of the Generalized Anxiety
Disorder Screener (GAD-7) in the general population.
Medical Care, 46(3):266–274.
N
´
ev
´
eol, A., Cohen, K. B., Grouin, C., Hamon, T., Lavergne,
T., Kelly, L., Goeuriot, L., Rey, G., Robert, A., Tan-
nier, X., and Zweigenbaum, P. (2016). Clinical infor-
HEALTHINF 2021 - 14th International Conference on Health Informatics
198