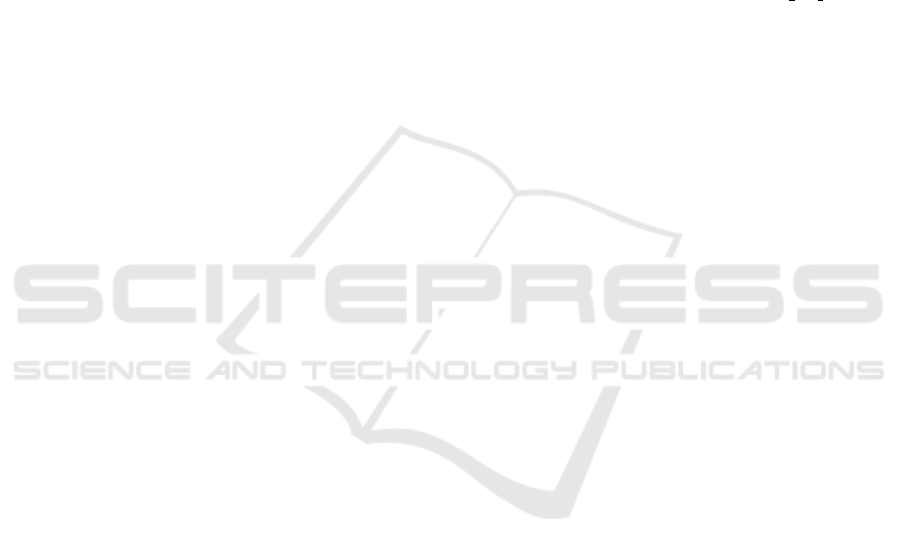
Conference on Image Processing - ICIP, pages 2045–
2048. IEEE.
Lin, M., Chen, Q., and Yan, S. (2013). Network in network.
Liu, Y. and Long, F. (2019). Acute lymphoblastic leukemia
cells image analysis with deep bagging ensemble
learning. In Lecture Notes in Bioengineering, pages
113–121. Springer Singapore.
Marzahl, C., Aubreville, M., Voigt, J., and Maier, A. (2019).
Classification of leukemic b-lymphoblast cells from
blood smear microscopic images with an attention-
based deep learning method and advanced augmenta-
tion techniques. In Lecture Notes in Bioengineering,
pages 13–22. Springer Singapore.
Mishra, S., Majhi, B., and Sa, P. K. (2019). Texture feature
based classification on microscopic blood smear for
acute lymphoblastic leukemia detection. Biomedical
Signal Processing and Control, 47:303–311.
Mishra, S., Sharma, L., Majhi, B., and Sa, P. K. (2016).
Microscopic image classification using DCT for the
detection of acute lymphoblastic leukemia (ALL).
In Advances in Intelligent Systems and Computing,
pages 171–180. Springer Singapore.
MoradiAmin, M., Memari, A., Samadzadehaghdam, N.,
Kermani, S., and Talebi, A. (2016). Computer aided
detection and classification of acute lymphoblastic
leukemia cell subtypes based on microscopic im-
age analysis. Microscopy Research and Technique,
79(10):908–916.
Moshavash, Z., Danyali, H., and Helfroush, M. S. (2018).
An automatic and robust decision support system
for accurate acute leukemia diagnosis from blood
microscopic images. Journal of Digital Imaging,
31(5):702–717.
Mourya, S., Kant, S., Kumar, P., Gupta, A., and Gupta, R.
(2018). LeukoNet: DCT-based CNN architecture for
the classification of normal versus Leukemic blasts in
B-ALL Cancer.
Mourya, S., Kant, S., Kumar, P., Gupta, A., and Gupta, R.
(2019). ALL challenge dataset of ISBI.
Pan, Y., Liu, M., Xia, Y., and Shen, D. (2019).
Neighborhood-correction algorithm for classification
of normal and malignant cells. In Lecture Notes in
Bioengineering, pages 73–82. Springer Singapore.
Prellberg, J. and Kramer, O. (2019). Acute lymphoblastic
leukemia classification from microscopic images us-
ing convolutional neural networks. In Lecture Notes
in Bioengineering, pages 53–61. Springer Singapore.
Putzu, L., Caocci, G., and Ruberto, C. D. (2014). Leuco-
cyte classification for leukaemia detection using im-
age processing techniques. Artificial Intelligence in
Medicine, 62(3):179–191.
Rawat, J., Singh, A., Bhadauria, H. S., Virmani, J., and De-
vgun, J. S. (2017). Classification of acute lymphoblas-
tic leukaemia using hybrid hierarchical classifiers.
Multimedia Tools and Applications, 76(18):19057–
19085.
Rehman, A., Abbas, N., Saba, T., ur Rahman, S. I.,
Mehmood, Z., and Kolivand, H. (2018). Classification
of acute lymphoblastic leukemia using deep learning.
Microscopy Research and Technique, 81(11):1310–
1317.
Russakovsky, O., Deng, J., Su, H., Krause, J., Satheesh,
S., Ma, S., Huang, Z., Karpathy, A., Khosla, A.,
Bernstein, M., Berg, A. C., and Fei-Fei, L. (2015).
ImageNet Large Scale Visual Recognition Challenge.
International Journal of Computer Vision (IJCV),
115(3):211–252.
SBILab (2019a). Classification of Normal
vs Malignant Cells in B-ALL White
Blood Cancer Microscopic Images: ISBI.
https://competitions.codalab.org/competitions/20395.
SBILab (2019b). Classification of Normal vs Malignant
Cells in B-ALL White Blood Cancer Micro-
scopic Images: Top Entries. https://competitions.
codalab.org/competitions/20395#learn the details-
top-entries-of-the- challenge.
SBILab (2020). Signal processing and Bio-medical Imag-
ing Lab. http://sbilab.iiitd.edu.in/.
Shafique, S. and Tehsin, S. (2018). Acute lymphoblastic
leukemia detection and classification of its subtypes
using pretrained deep convolutional neural networks.
Technology in Cancer Research & Treatment, 17.
Shah, S., Nawaz, W., Jalil, B., and Khan, H. A. (2019).
Classification of normal and leukemic blast cells in
B-ALL cancer using a combination of convolutional
and recurrent neural networks. In Lecture Notes in
Bioengineering, pages 23–31. Springer Singapore.
Simonyan, K. and Zisserman, A. (2014). Very deep convo-
lutional networks for large-scale image recognition.
Verma, E. and Singh, V. (2019). ISBI challenge 2019: Con-
volution neural networks for b-ALL cell classification.
In Lecture Notes in Bioengineering, pages 131–139.
Springer Singapore.
Xiao, F., Kuang, R., Ou, Z., and Xiong, B. (2019).
DeepMEN: Multi-model ensemble network for b-
lymphoblast cell classification. In Lecture Notes in
Bioengineering, pages 83–93. Springer Singapore.
VISAPP 2021 - 16th International Conference on Computer Vision Theory and Applications
692