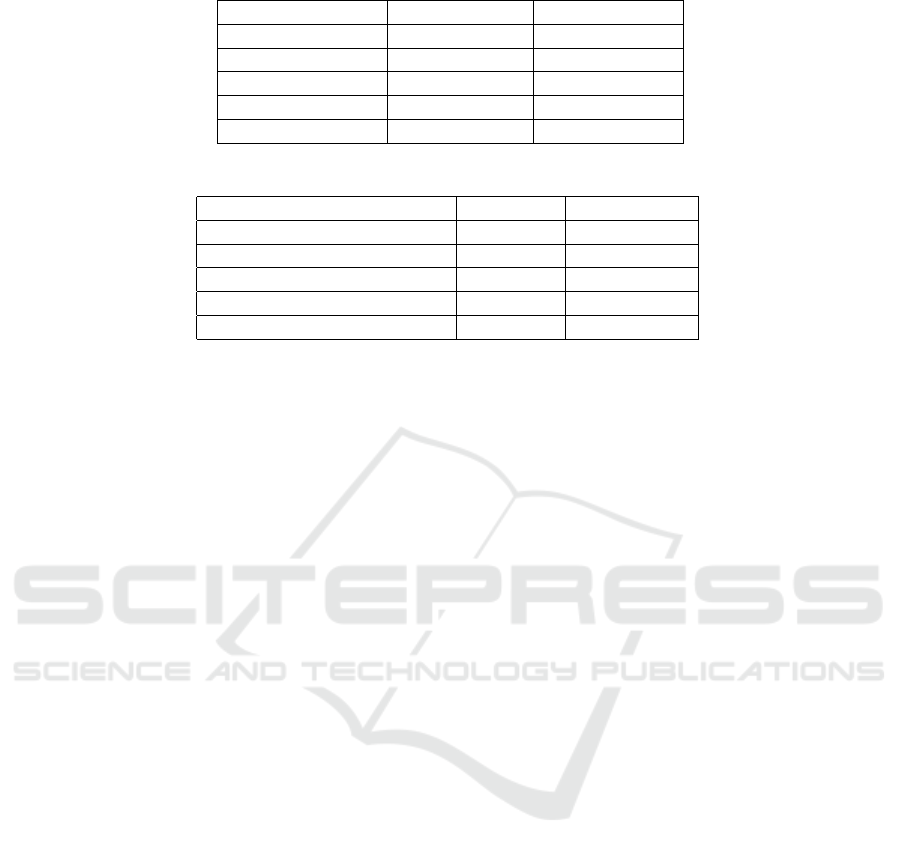
Table 3: Comparison Between Different Methods for the Classification QRS task.
Method Sensitivity (Se) Specificity (+P)
Baseline Vendor 1 0.687 0.961
Baseline Vendor 2 0.318 0.955
Neural Network 0.873 0.997
NN + GBDT 0.917 0.999
Cardiologist 0.872 0.999
Table 4: QRS detection performance comparison on the MIT-BIH arrhythmia database.
Work Recall (%) Precision (%)
Pan and Tompkins (1985) 90.95 99.56
Elgendi et al. (2009) 87.90 97.60
Chouakri et al. (2011) 98.68 97.24
Rodriguez-Jorge et al. (2014) 96.28 99.71
NN + GBDT (our work) 98.11 99.91
6 CONCLUSIONS
In this paper we present a novel heartbeat detection
and heartbeat classification (narrow or wide) method
for the two-channel long-term ECGs. We propose a
channel-wise CNN architecture and combine it with
the GBDT model that can employ patient-wise fea-
tures. Furthermore, we demonstrate on the set of 291
ambulatory 2-lead ECG 24-hour recordings that our
method significantly outperforms two commercially
available software packages widely used by the car-
diologists for these tasks in the country, approaching
the quality level of experienced radiologists.
As a future work, we intend to conduct prospec-
tive clinical trials for confirmation of clinical signifi-
cance of this model, as well as enhancing our model
for the detection and interpretation of more complex
components of the heartbeat.
REFERENCES
Bond, R., Novotny, T., Andrsova, T., Koc, L., Sisakova, M.,
Finlay, D., and Malik, M. (2018). Automation bias
in medicine: The influence of automated diagnoses
on interpreter accuracy and uncertainty when reading
electrocardiograms. Journal of Electrocardiology.
Essam, H., Kilany, M., and A.Hassanien (2017). ECG sig-
nals classification: a review. International Journal of
Medical Engineering and Informatics.
Estes, N. (2013). Computerized interpretation of ECGs:
supplement not a substitute. Circulation. Arrhythmia
and Electrophysiology.
Everss-Villalba, E., Melgarejo-Meseguer, F., Blanco-
Velasco, M., Gimeno-Blanes, F., Sala-Pla, S., Rojo-
´
Alvarez, J., and Garc
´
ıa-Alberola, A. (2017). Noise
Maps for Quantitative and Clinical Severity To-
wards Long-Term ECG Monitoring. Sensors (Basel,
Switzerland).
Hong, S., Zhou, Y., Shang, J., Xiao, C., and Sun, J.
(2019). Opportunities and Challenges in Deep Learn-
ing Methods on Electrocardiogram Data: A System-
atic Review. arXiv:2001.01550.
Kaplan, B., Uysal, A., Gunal, E. S., Ergin, S., Gunal, S., and
Gulmezoglu, M. (2018). A survey on ECG analysis.
Biomedical Signal Processing and Control.
Ke, G., Meng, Q., Finley, T., Wng, T., Chen, W., Ma, W.,
Ye, Q., and Liu, T. (2017). LightGBM: A Highly Ef-
ficient Gradient Boosting Decision Tree. Advances in
Neural Information Processing Systems.
Li, Q., Mark, R., and Clifford, G. (2008). Robust heart rate
estimation from multiple asynchronous noisy sources
using signal quality indices and a Kalman filter.
SPhysiol.
Lindow, T., Kron, J., Thulesius, H., Ljungstr
¨
om, E., and
Pahlm, O. (2019). Erroneous computer-based inter-
pretations of atrial fibrillation and atrial flutter in a
Swedish primary health care setting. Biomedical Sig-
nal Processing and Control.
Moody, G. and Mark, G. (2001). The impact of the MIT-BIH
Arrhythmia Database. IEEE Engineering in Medicine
and Biology Magazine.
Osowski, S. and Linh, T. (2001). ECG beat recognition
using fuzzy hybrid neural network. IEEE Transactions
on Biomedical Engineering.
Rajpurkar, P., Hannun, A., Haghpanahi, M., Bourn, C.,
and Ng, A. (2017). Cardiologist-Level Arrhyth-
mia Detection with Convolutional Neural Networks.
arXiv:1707.01836.
Rodriguez, H., Mexicano, R., Bila, A., Ponce, J., Cervantes,
R., and Salvador (2014). Feature Extraction of Elec-
trocardiogram Signals by Applying Adaptive Thresh-
old and Principal Component Analysis. Journal of
Applied Research and Technology.
Salamon, J. and Bello, J. (2017). Deep Convolutional Neu-
Noise-resilient Automatic Interpretation of Holter ECG Recordings
213