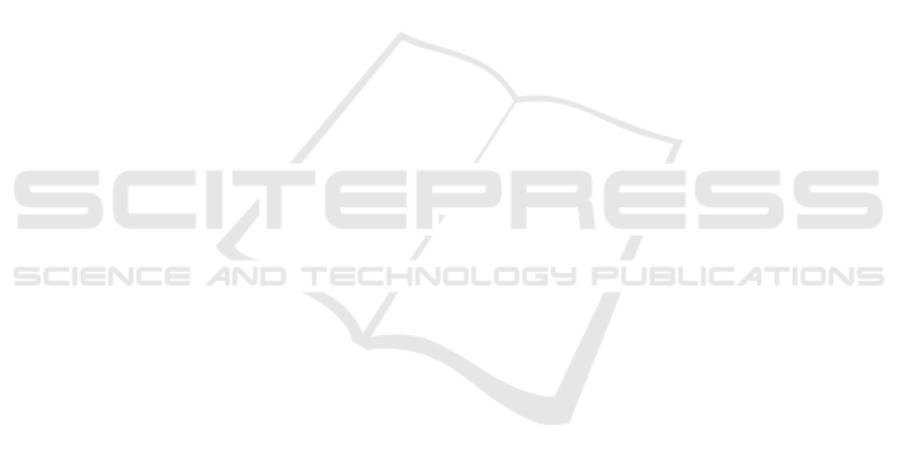
REFERENCES
Babenko, A., Slesarev, A., Chigorin, A., and Lempitsky,
V. (2014). Neural codes for image retrieval. In Euro-
pean Conference on Computer Vision, pages 584–599.
Springer.
Bernini, N., Bertozzi, M., Castangia, L., Patander, M., and
Sabbatelli, M. (2014). Real-time obstacle detection
using stereo vision for autonomous ground vehicles:
A survey. In 17th International IEEE Conference on
Intelligent Transportation Systems (ITSC), pages 873–
878.
Chaker, A., Kaaniche, M., and Benazza-Benyahia, A.
(2015). Disparity based stereo image retrieval through
univariate and bivariate models. Signal Processing:
Image Communication, 31:174–184.
Feng, Y., Ren, J., and Jiang, J. (2011). Generic framework
for content-based stereo image/video retrieval. Elec-
tronics Letters, 47(2):97–98.
Ghodhbani, E., Kaaniche, M., and Benazza-Benyahia, A.
(2019). Depth-based color stereo images retrieval us-
ing joint multivariate statistical models. Signal Pro-
cessing: Image Communication, 76:272–282.
Hara, K., Kataoka, H., and Satoh, Y. (2017). Learning
spatio-temporal features with 3d residual networks for
action recognition. In Proceedings of the IEEE Inter-
national Conference on Computer Vision Workshops,
pages 3154–3160.
Hara, K., Kataoka, H., and Satoh, Y. (2018). Can spa-
tiotemporal 3d CNNs retrace the history of 2d cnns
and imagenet. In Proceedings of the IEEE conference
on Computer Vision and Pattern Recognition, pages
6546–6555.
He, K., Zhang, X., Ren, S., and Sun, J. (2016). Deep resid-
ual learning for image recognition. In Proceedings of
the IEEE Conference on Computer Vision and Pattern
Recognition, pages 770–778.
Ji, Y., Zhang, H., and Wu, Q. J. (2018). Salient object detec-
tion via multi-scale attention CNN. Neurocomputing,
322:130–140.
Kalantidis, Y., Mellina, C., and Osindero, S. (2016). Cross-
dimensional weighting for aggregated deep convolu-
tional features. In European conference on computer
vision, pages 685–701. Springer.
Karpathy, A., Toderici, G., Shetty, S., Leung, T., Suk-
thankar, R., and Fei-Fei, L. (2014). Large-scale video
classification with convolutional neural networks. In
Proceedings of the IEEE Conference on Computer Vi-
sion and Pattern Recognition, pages 1725–1732.
Kim, M., Lee, J., and Whon, K. (2014). Sparogram:
the spatial augmented reality holographic display for
3d visualization and exhibition. In IEEE VIS In-
ternational Workshop on 3DVis, pages 81–86, Paris,
France.
Kingma, D. P. and Ba, J. (2014). Adam: A
method for stochastic optimization. arXiv preprint
arXiv:1412.6980.
LeCun, Y., Bengio, Y., and Hinton, G. (2015). Deep learn-
ing. Nature, 521(7553):436–444.
Long, J., Shelhamer, E., and Darrell, T. (2015). Fully con-
volutional networks for semantic segmentation. In
Proceedings of the IEEE Conference on Computer Vi-
sion and Pattern Recognition, pages 3431–3440.
Ma, C., Guo, Y., Yang, J., and An, W. (2018). Learn-
ing multi-view representation with lstm for 3-d shape
recognition and retrieval. IEEE Transactions on Mul-
timedia, 21(5):1169–1182.
Mayer, N., Ilg, E., Hausser, P., Fischer, P., Cremers, D.,
Dosovitskiy, A., and Brox, T. (2016). A large dataset
to train convolutional networks for disparity, optical
flow, and scene flow estimation. In Proceedings of
the IEEE Conference on Computer Vision and Pattern
Recognition, pages 4040–4048.
Peng, F., Wang, L., Gong, J., and Wu, H. (2015). Devel-
opment of a framework for stereo image retrieval with
both height and planar features. IEEE Journal of Se-
lected Topics in Applied Earth Observation and Re-
mote Sensing, 8(2):800–815.
Sdiri, B., Kaaniche, M., Cheikh, F. A., Beghdadi, A., and
Elle, O. J. (2019). Efficient enhancement of stereo
endoscopic images based on joint wavelet decompo-
sition and binocular combination. IEEE Transactions
on Medical Imaging, 38(1):33–45.
Simonyan, K. and Zisserman, A. (2015). Very deep con-
volutional networks for large-scale image recognition.
In International Conference on Learning Representa-
tions.
Su, H., Maji, S., Kalogerakis, E., and Learned-Miller, E.
(2015). Multi-view convolutional neural networks for
3d shape recognition. In Proceedings of the IEEE
International Conference on Computer Vision, pages
945–953.
Tolias, G., Sicre, R., and J
´
egou, H. (2015). Particular object
retrieval with integral max-pooling of cnn activations.
arXiv preprint arXiv:1511.05879.
Tran, D., Ray, J., Shou, Z., Chang, S.-F., and Paluri, M.
(2017). Convnet architecture search for spatiotempo-
ral feature learning. arXiv preprint arXiv:1708.05038.
Zhang, B., Wang, L., Wang, Z., Qiao, Y., and Wang, H.
(2018). Real-time action recognition with deeply
transferred motion vector cnns. IEEE Transactions on
Image Processing, 27(5):2326–2339.
Zheng, L., Huang, Y., Lu, H., and Yang, Y. (2019). Pose-
invariant embedding for deep person re-identification.
IEEE Transactions on Image Processing, 28(9):4500–
4509.
An Effective 3D ResNet Architecture for Stereo Image Retrieval
387