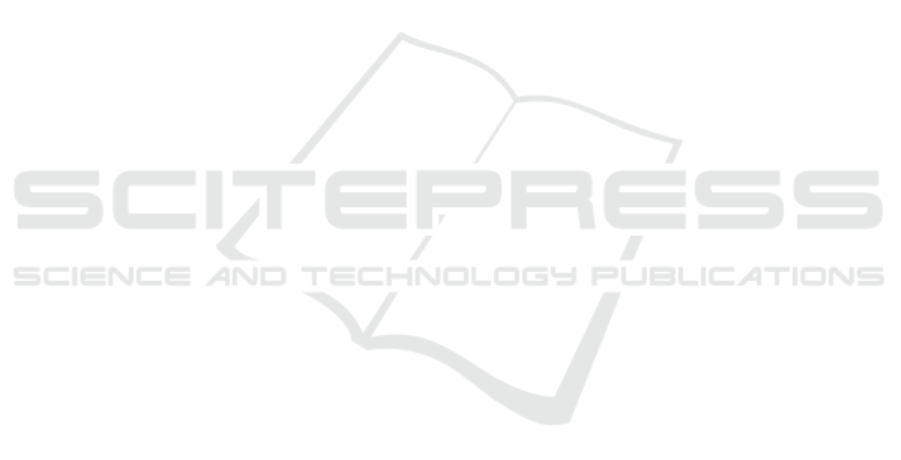
Chen, Z., Fu, Y., Zhang, Y., Jiang, Y., Xue, X., and Sigal,
L. (2019). Multi-level semantic feature augmentation
for one-shot learning. IEEE Transactions on Image
Processing, pages 1–1.
Dai, B., Zhang, Y., and Lin, D. (2017). Detecting visual
relationships with deep relational networks. In Pro-
ceedings of the IEEE Conference on Computer Vision
and Pattern Recognition (CVPR).
Gorniak, P. and Roy, D. (2004). Grounded semantic com-
position for visual scenes. The Journal of Artificial
Intelligence Research, 21:429–470.
G
¨
ardenfors, P. (2000). Conceptual Spaces: The Geometry
of Thought. MIT Press, Cambridge, MA, USA.
He, X., Qiao, P., Dou, Y., and Niu, X. (2019). Spatial atten-
tion network for few-shot learning. In ICANN 2019:
Deep Learning, pages 567–578, Cham. Springer In-
ternational Publishing.
Hotz, L., Neumann, B., Terzi
´
c, K., and
ˇ
Sochman, J.
(2007). Feedback between low-level and high-level
image processing. Technical Report Report FBI-HH-
B-278/07, University of Hamburg, Hamburg.
Hudson, D. A. and Manning, C. D. (2019). Gqa: A new
dataset for real-world visual reasoning and composi-
tional question answering. Conference on Computer
Vision and Pattern Recognition (CVPR).
Jin, X., Du, J., Sadhu, A., Nevatia, R., and Ren, X.
(2020). Visually grounded continual learning of com-
positional phrases.
Johnson, J., Hariharan, B., Van Der Maaten, L., Hoffman,
J., Fei-Fei, L., Zitnick, C. L., and Girshick, R. (2017).
Inferring and executing programs for visual reasoning.
In 2017 IEEE International Conference on Computer
Vision (ICCV), pages 3008–3017.
Karlinsky, L., Shtok, J., Harary, S., Schwartz, E., Aides, A.,
Feris, R., Giryes, R., and Bronstein, A. M. (2019).
Repmet: Representative-based metric learning for
classification and few-shot object detection. In Pro-
ceedings of the IEEE/CVF Conference on Computer
Vision and Pattern Recognition (CVPR).
Kovashka, A., Parikh, D., and Grauman, K. (2015). Whit-
tlesearch: Interactive image search with relative at-
tribute feedback. In International Journal of Com-
puter Vision (IJCV).
Kreutzmann, A., Terzi
´
c, K., and Neumann, B. (2009).
Context-aware classification for incremental scene in-
terpretation. In Workshop on Use of Context in Vision
Processing, Boston.
Krishna, R., Chami, I., Bernstein, M., and Fei-Fei, L.
(2018). Referring relationships. In IEEE Conference
on Computer Vision and Pattern Recognition.
Liang, K., Guo, Y., Chang, H., and Chen, X. (2018). Visual
relationship detection with deep structural ranking. In
AAAI.
Liang, X., Lee, L., and Xing, E. P. (2017). Deep variation-
structured reinforcement learning for visual relation-
ship and attribute detection. In CVPR, pages 4408–
4417.
Liao, W., Rosenhahn, B., Shuai, L., and Ying Yang, M.
(2019). Natural language guided visual relationship
detection. In Proceedings of the IEEE/CVF Con-
ference on Computer Vision and Pattern Recognition
(CVPR) Workshops.
Lu, C., Krishna, R., Bernstein, M., and Fei-Fei, L. (2016).
Visual relationship detection with language priors. In
ECCV.
Luo, R. and Shakhnarovich, G. (2017). Comprehension-
guided referring expressions. In Proceedings of the
IEEE Conference on Computer Vision and Pattern
Recognition (CVPR).
Neumann, B. and Terzi
´
c, K. (2010). Context-based proba-
bilistic scene interpretation. In Proc. Third IFIP Int.
Conf. on Artificial Intelligence in Theory and Prac-
tice, pages 155–164, Brisbane.
Parsons, T. (1991). Events in the Semantics of English.
Peyre, J., Laptev, I., Schmid, C., and Sivic, J. (2017).
Weakly-supervised learning of visual relations. In
ICCV.
Peyre, J., Laptev, I., Schmid, C., and Sivic, J. (2019). De-
tecting unseen visual relations using analogies. In The
IEEE International Conference on Computer Vision
(ICCV).
Redmon, J. and Farhadi, A. (2018). Yolov3: An incremental
improvement. arXiv.
Richter, M., Lins, J., Schneegans, S., and Sch
¨
oner, G.
(2014). A neural dynamic architecture resolves
phrases about spatial relations in visual scenes. In
Artificial Neural Networks and Machine Learning –
ICANN 2014, pages 201–208.
Sohn, K. (2016). Improved deep metric learning with multi-
class n-pair loss objective. In NIPS.
Sur
´
ıs, D., Epstein, D., Ji, H., Chang, S.-F., and Vondrick, C.
(2019). Learning to learn words from visual scenes.
arXiv preprint arXiv:1911.11237.
Xu, F. and Tenenbaum, J. B. (2000). Word learning as
bayesian inference. In In Proceedings of the 22nd
Annual Conference of the Cognitive Science Society,
pages 517–522. Erlbaum.
Yu, R., Li, A., Morariu, V. I., and Davis, L. S. (2017). Vi-
sual relationship detection with internal and external
linguistic knowledge distillation. In 2017 IEEE In-
ternational Conference on Computer Vision (ICCV),
pages 1068–1076.
Zhang, J., Zhao, C., Ni, B., Xu, M., and Yang, X. (2019).
Variational few-shot learning. In The IEEE Interna-
tional Conference on Computer Vision (ICCV).
VISAPP 2021 - 16th International Conference on Computer Vision Theory and Applications
156