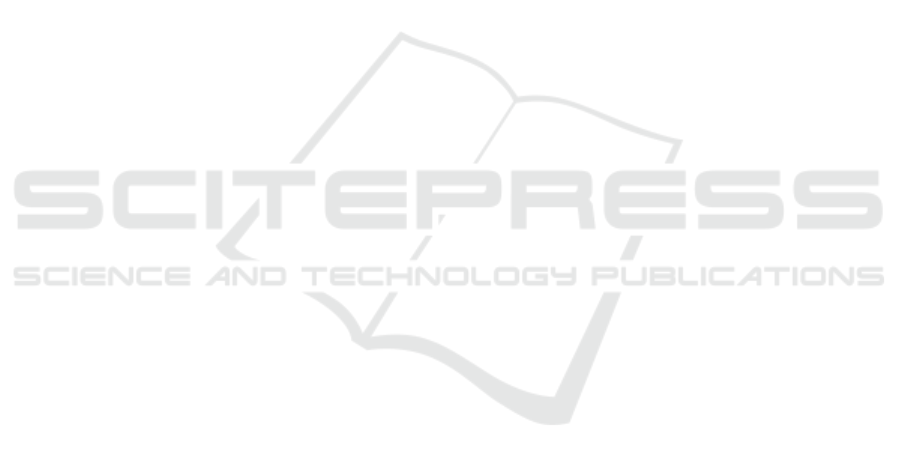
Multi-view score fusion for content-based mammo-
gram retrieval. In Proceedings of SPIE 9875, Eighth
International Conference on Machine Vision, page
987515.
Gao, X., Mu, T., Goulermas, J. Y., Thiyagalingam, J.,
and Wang, M. (2020). An interpretable deep archi-
tecture for similarity learning built upon hierarchical
concepts. IEEE Transactions On Image Processing,
29:3911–3926.
Gu, Y., Vyas, K., Yang, J., and Yang, G.-Z. (2017). Unsu-
pervised feature learning for endomicroscopy image
retrieval. In International Conference on Medical Im-
age Computing and Computer-Assisted Intervention,
pages 64–71.
Hao, X., Bao, Y., Guo, Y., Yu, M., Zhang, D., Risacher,
S. L., Saykin, A. J., Yao, X., Shen, L., and for the
Alzheimer’s Disease Neuroimaging Initiative (2020).
Multi-modal neuroimaging feature selection with con-
sistent metric constraint for diagnosis of alzheimer’s
disease. Medical Image Analysis, 60:101625.
Heath, M., Bowyer, K., Kopans, D., Jr, P. K., Moore, R.,
Chang, K., and Munishkumaran, S. (1998). Current
status of the digital database for screening mammog-
raphy. Digital Mammography Computational Imag-
ing and Vision, 13:457—-460.
Jouirou, A., Ba
ˆ
azaoui, A., and Barhoumi, W. (2019). Multi-
view information fusion in mammograms: A compre-
hensive overview. Information Fusion, 52:308–321.
Jouirou, A., Ba
ˆ
azaoui, A., Barhoumi, W., and Zagrouba,
E. (2015). Curvelet-based locality sensitive hash-
ing for mammogram retrieval in large-scale datasets.
In 12th ACS/IEEE International Conference on Com-
puter Systems and Applications (AICCSA), pages 1–8.
IEEE.
Khan, H. N., Shahid, A. R., Raza, B., Dar, A. H., and
Alquhayz, H. (2019). Multi-view feature fusion based
four views model for mammogram classification us-
ing convolutional neural network. IEEE Access,
7:165724–165733.
Kiruthika, K., Vijayan, D., and R, L. (2019). Retrieval
driven classification for mammographic masses. In
International Conference on Communication and Sig-
nal Processing, page 18619401. IEEE.
Krishnasamy, G. and Paramesran, R. (2019). Multiview
laplacian semisupervised feature selection by leverag-
ing shared knowledge among multiple tasks. Signal
Processing: Image Communication, 70:68–78.
Liu, Q., Dou, Q., Yu, L., and Heng, P. A. (2020a). Ms-net:
Multi-site network for improving prostate segmenta-
tion with heterogeneous mri data. IEEE Transactions
on Medical Imaging, 39(9):2713–2724.
Liu, W., ran Wei, Y., and qian Liu, C. (2020b). Mam-
mographic mass retrieval using multi-view informa-
tion and laplacian score feature selection. In Proceed-
ings of the 2nd International Conference on Intelligent
Medicine and Image Processing, pages 75–79.
Liu, W., Xu, W., Li, L., Li, S., Zhao, H., and Zhang, J.
(2011). Mammographic mass retrieval based on multi-
view information. In 5th International Conference on
Bioinformatics and Biomedical Engineering (iCBBE),
page 12072452.
Martens, R. M., Koopman, T., Lavini, C., Ali, M., Peeters,
C. F. W., Noij, D. P., Zwezerijnen, G., Marcus, J. T.,
Vergeer, M. R., Leemans, C. R., de Bree, R., de Graaf,
P., Boellaard, R., and Castelijns, J. A. (2020). Multi-
parametric functional mri and 18f-fdg-pet for survival
prediction in patients with head and neck squamous
cell carcinoma treated with (chemo)radiation. Euro-
pean Radiology, https://doi.org/10.1007/s00330-020-
07163-3.
Narvaez, F., Diaz, G., and Romero, E. (2011). Multi-view
information fusion for automatic bi-rads description
of mammographic masses. In Proceedings of SPIE
7963, Medical Imaging 2011: Computer-Aided Diag-
nosis, pages 84—-89.
Rasheed, A. S., Zabihzadeh, D., and Al-Obaidi, S. A. R.
(2020). Large-scale multi-modal distance metric
learning with application to content-based informa-
tion retrieval and image classification. International
Journal of Pattern Recognition and Artificial Intelli-
gence, https://doi.org/10.1142/S0218001420500342.
Rayen, S. and Subhashini, R. (2020). An effi-
cient mammogram image retrieval system using an
optimized classifier. Neural Processing Letters,
https://doi.org/10.1007/s11063-020-10254-3.
Sapate, S., Talbar, S., Mahajan, A., Sable, N., Desai, S.,
and Thakur, M. (2020). Breast cancer diagnosis using
abnormalities on ipsilateral views of digital mammo-
grams. Biocybernetics and Biomedical Engineering,
40(1):290–305.
Sbei, A., ElBedoui, K., Barhoumi, W., and Maktouf, C.
(2020). Gradient-based generation of intermediate
images for heterogeneous tumor segmentation within
hybrid pet/mri scans. Computers in Biology and
Medicine, 119:103669.
Singh, V. P., Srivastava, S., and Srivastava, R. (2018). Auto-
mated and effective content-based image retrieval for
digital mammography. Journal of X-Ray Science and
Technology, 26(1):29–49.
Soudani, A. and Barhoumi, W. (2019). An image-based
segmentation recommender using crowdsourcing and
transfer learning for skin lesion extraction. Expert Sys-
tems with Applications, 118:400–410.
Wei, G., Cao, H., Ma, H., Qi, S., Qian, W., and Ma, Z.
(2017). Content-based image retrieval for lung nodule
classification using texture features and learned dis-
tance metric. Journal of Medical Systems, 42(13):1–7.
Wu, W., Tao, D., Li, H., Yang, Z., and Cheng, J. (2020).
Deep features for person re-identification on metric
learning. Pattern Recognition, 110:107424.
Yang, L., Jin, R., Mummert, L., Sukthankar, R., Goode, A.,
Zheng, B., Hoi, S. C., and Satyanarayanan, M. (2010).
A boosting framework for visuality-preserving dis-
tance metric learning and its application to medical
image retrieval. IEEE Transactions On Pattern Anal-
ysis And Machine Intelligence, 32(1):30–44.
Shared Information-Based Late Fusion for Four Mammogram Views Retrieval using Data-driven Distance Selection
155