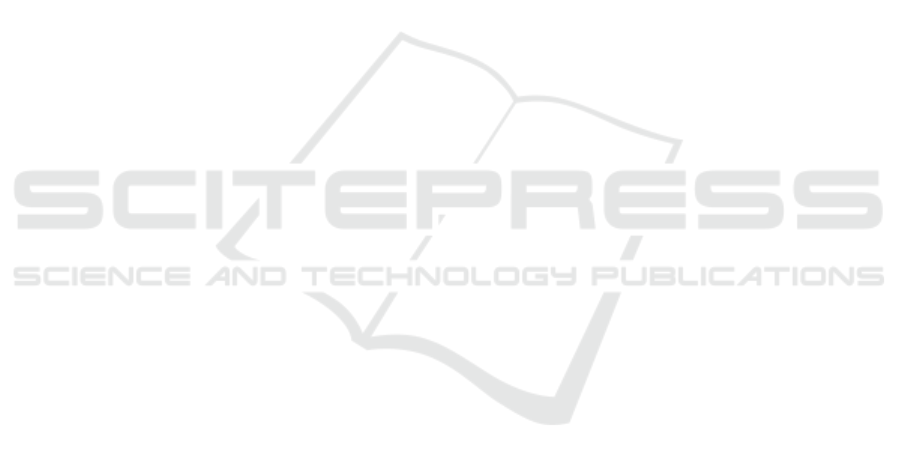
Hodan, T., Michel, F., Brachmann, E., Kehl, W., GlentBuch,
A., Kraft, D., Drost, B., Vidal, J., Ihrke, S., Zabulis,
X., et al. (2018). Bop: Benchmark for 6D object pose
estimation. In Proceedings of the European Confer-
ence on Computer Vision (ECCV), pages 19–34.
Hou, Q., Cheng, M.-M., Hu, X., Borji, A., Tu, Z., and
Torr, P. H. (2017). Deeply supervised salient object
detection with short connections. In Proceedings of
the IEEE Conference on Computer Vision and Pattern
Recognition, pages 3203–3212.
Krizhevsky, A., Sutskever, I., and Hinton, G. E. (2012). Im-
agenet classification with deep convolutional neural
networks. In Advances in neural information process-
ing systems, pages 1097–1105.
Lai, K., Bo, L., Ren, X., and Fox, D. (2011a). A large-
scale hierarchical multi-view RGB-D object dataset.
In 2011 IEEE international conference on robotics
and automation, pages 1817–1824. IEEE.
Lai, K., Bo, L., Ren, X., and Fox, D. (2011b). Sparse dis-
tance learning for object recognition combining rgb
and depth information. In 2011 IEEE International
Conference on Robotics and Automation, pages 4007–
4013. IEEE.
Liu, H., Li, F., Xu, X., and Sun, F. (2018). Multi-modal lo-
cal receptive field extreme learning machine for object
recognition. Neurocomputing, 277:4–11.
Liu, W., Anguelov, D., Erhan, D., Szegedy, C., Reed, S.,
Fu, C.-Y., and Berg, A. C. (2016). SSD: Single shot
multibox detector. In European conference on com-
puter vision, pages 21–37. Springer.
Marcon, M., Spezialetti, R., Salti, S., Silva, L., and Di Ste-
fano, L. (2019). Boosting object recognition in point
clouds by saliency detection. In International Confer-
ence on Image Analysis and Processing, pages 321–
331. Springer.
Ouadiay, F. Z., Zrira, N., Bouyakhf, E. H., and Himmi,
M. M. (2016). 3d object categorization and recogni-
tion based on deep belief networks and point clouds.
In Proceedings of the 13th International Conference
on Informatics in Control, Automation and Robotics
- Volume 2: ICINCO,, pages 311–318. INSTICC,
SciTePress.
Park, J., Zhou, Q.-Y., and Koltun, V. (2017). Colored point
cloud registration revisited. In Proceedings of the
IEEE International Conference on Computer Vision,
pages 143–152.
Redmon, J., Divvala, S., Girshick, R., and Farhadi, A.
(2016). You only look once: Unified, real-time object
detection. In Proceedings of the IEEE conference on
computer vision and pattern recognition, pages 779–
788.
Rusu, R. B., Blodow, N., and Beetz, M. (2009). Fast point
feature histograms (FPFH) for 3D registration. In
2009 IEEE international conference on robotics and
automation, pages 3212–3217. IEEE.
Rusu, R. B., Blodow, N., Marton, Z. C., and Beetz, M.
(2008). Aligning point cloud views using persistent
feature histograms. In 2008 IEEE/RSJ international
conference on intelligent robots and systems, pages
3384–3391. IEEE.
Salti, S., Tombari, F., and Di Stefano, L. (2014). SHOT:
Unique signatures of histograms for surface and tex-
ture description. Computer Vision and Image Under-
standing, 125:251–264.
Sandler, M., Howard, A., Zhu, M., Zhmoginov, A., and
Chen, L.-C. (2018). Mobilenetv2: Inverted residu-
als and linear bottlenecks. In Proceedings of the IEEE
conference on computer vision and pattern recogni-
tion, pages 4510–4520.
Schwarz, M., Schulz, H., and Behnke, S. (2015). RGB-
D object recognition and pose estimation based on
pre-trained convolutional neural network features. In
2015 IEEE international conference on robotics and
automation (ICRA), pages 1329–1335. IEEE.
Simonyan, K. and Zisserman, A. (2015). Very deep con-
volutional networks for large-scale image recognition.
In International Conference on Learning Representa-
tions.
Szegedy, C., Liu, W., Jia, Y., Sermanet, P., Reed, S.,
Anguelov, D., Erhan, D., Vanhoucke, V., and Rabi-
novich, A. (2015). Going deeper with convolutions.
In Proceedings of the IEEE conference on computer
vision and pattern recognition, pages 1–9.
Szegedy, C., Vanhoucke, V., Ioffe, S., Shlens, J., and Wo-
jna, Z. (2016). Rethinking the inception architecture
for computer vision. In Proceedings of the IEEE con-
ference on computer vision and pattern recognition,
pages 2818–2826.
Tan, M. and Le, Q. (2019). Efficientnet: Rethinking model
scaling for convolutional neural networks. In Interna-
tional Conference on Machine Learning, pages 6105–
6114.
Tan, M., Pang, R., and Le, Q. V. (2020). Efficientdet: Scal-
able and efficient object detection. In Proceedings of
the IEEE/CVF Conference on Computer Vision and
Pattern Recognition, pages 10781–10790.
Vock, R., Dieckmann, A., Ochmann, S., and Klein, R.
(2019). Fast template matching and pose estimation
in 3D point clouds. Computers & Graphics, 79:36–
45.
Xie, S., Girshick, R., Doll
´
ar, P., Tu, Z., and He, K. (2017).
Aggregated residual transformations for deep neural
networks. In Proceedings of the IEEE conference on
computer vision and pattern recognition, pages 1492–
1500.
Zaki, H. F., Shafait, F., and Mian, A. (2019). Viewpoint in-
variant semantic object and scene categorization with
RGB-D sensors. Autonomous Robots, 43(4):1005–
1022.
Zhou, Q.-Y., Park, J., and Koltun, V. (2016). Fast global
registration. In European Conference on Computer
Vision, pages 766–782. Springer.
Zia, S., Yuksel, B., Yuret, D., and Yemez, Y. (2017). RGB-
D object recognition using deep convolutional neural
networks. In Proceedings of the IEEE International
Conference on Computer Vision Workshops, pages
896–903.
VISAPP 2021 - 16th International Conference on Computer Vision Theory and Applications
174