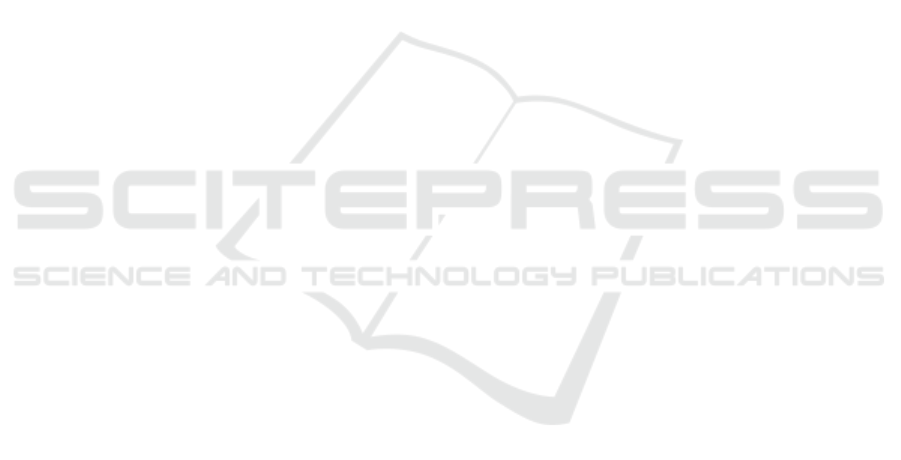
contrary to what has been reported in related works.
In Serigo et al.’s study, the time interval between typ-
ing events was longer when the valence was high
compared with when the valence was low (Salmeron-
Majadas et al., 2014). Our experiment reported this
time as being shorter. This observation can be at-
tributed to the specific experimental design imple-
mented. Serigo et al. introduced a time limit for
task completion to create stress on the participants,
thus impacting their affective states. In our study, the
participants’ affective states were impacted through
a certain communication stress, such as strict replies
from the reviewer, to simulate the understanding that
the stress is usually caused by the actual content of the
communication. Hence, the results obtained through
our experimental design are useful in understanding
the impact on affective state during CMC.
The features related to vibration amplitude met a
significant level (p<0.05) for classifying high or low
valence and arousal in several cases. Thus, it was es-
tablished that the features related to typing force are
effective in the estimation of arousal.
7 CONCLUSION
In this paper, we proposed a method to estimate va-
lence and arousal using keyboard input and typing vi-
bration information. Effective features were selected
through statistical tests, and the unlearned partici-
pants’ data were classified to investigate versatility.
This time, the average accuracies and standard devi-
ations were 69.8% ± 4.8% for valence and 71.1% ±
5.8% for arousal. Thus, it was established that it is
possible to estimate valence and arousal with high ac-
curacy for the unlearned participants’ data by specify-
ing the features and using keyboard input and typing
vibration information.
In future study, it is necessary to further improve
the accuracy by selecting features specifically suitable
for each individual. Further, the determination of es-
sential features that are common across keyboards is
required since each keyboards have different charac-
teristics.
It is expected that the findings of this study will
facilitate smooth computer-mediated communication
in the near future, avoiding misinterpretation of other
people’s messages.
REFERENCES
Bixler, R. and D’Mello, S. (2013). Detecting boredom and
engagement during writing with keystroke analysis,
task appraisals, and stable traits. In Proceedings of
the 2013 international conference on Intelligent user
interfaces, pages 225–234.
Bos, D. O. et al. (2006). Eeg-based emotion recognition.
The Influence of Visual and Auditory Stimuli, 56(3):1–
17.
Felipe, D. A. M., Gutierrez, K. I. N., Quiros, E. C. M., and
Vea, L. A. (2012). Towards the development of in-
telligent agent for novice c/c++ programmers through
affective analysis of event logs. In Proc. Int. Multi-
Conference Eng. Comput. Sci, volume 1, page 2012.
Citeseer.
Hassib, M., Buschek, D., Wozniak, P. W., and Alt, F. (2017).
Heartchat: Heart rate augmented mobile chat to sup-
port empathy and awareness. In Proceedings of the
2017 CHI Conference on Human Factors in Comput-
ing Systems, pages 2239–2251.
Hernandez, J., Paredes, P., Roseway, A., and Czerwinski,
M. (2014). Under pressure: sensing stress of computer
users. In Proceedings of the SIGCHI conference on
Human factors in computing systems, pages 51–60.
Khan, I. A., Brinkman, W.-P., and Hierons, R. (2013). To-
wards estimating computer users’ mood from interac-
tion behaviour with keyboard and mouse. Frontiers of
Computer Science, 7(6):943–954.
Khanna, P. and Sasikumar, M. (2010). Recognising emo-
tions from keyboard stroke pattern. International jour-
nal of computer applications, 11(9):1–5.
Kruger, J., Epley, N., Parker, J., and Ng, Z.-W. (2005). Ego-
centrism over e-mail: Can we communicate as well as
we think? Journal of personality and social psychol-
ogy, 89(6):925.
Lin, Y.-P., Wang, C.-H., Jung, T.-P., Wu, T.-L., Jeng, S.-K.,
Duann, J.-R., and Chen, J.-H. (2010). Eeg-based emo-
tion recognition in music listening. IEEE Transactions
on Biomedical Engineering, 57(7):1798–1806.
Lv, H.-R., Lin, Z.-L., Yin, W.-J., and Dong, J. (2008). Emo-
tion recognition based on pressure sensor keyboards.
In 2008 IEEE International Conference on Multime-
dia and Expo, pages 1089–1092. IEEE.
Russell, J. A. (1980). A circumplex model of affect. Journal
of personality and social psychology, 39(6):1161.
Salmeron-Majadas, S., Santos, O. C., and Boticario, J. G.
(2014). An evaluation of mouse and keyboard inter-
action indicators towards non-intrusive and low cost
affective modeling in an educational context. Proce-
dia Computer Science, 35:691–700.
Slack, I. (2019). Slack, Inc. https://slack.com/ (reference
date, October 1st ,2020).
Wang, H., Prendinger, H., and Igarashi, T. (2004). Commu-
nicating emotions in online chat using physiological
sensors and animated text. In CHI’04 extended ab-
stracts on Human factors in computing systems, pages
1171–1174.
Wu, G., Liu, G., and Hao, M. (2010). The analysis of emo-
tion recognition from gsr based on pso. In 2010 Inter-
national symposium on intelligence information pro-
cessing and trusted computing, pages 360–363. IEEE.
BIOSIGNALS 2021 - 14th International Conference on Bio-inspired Systems and Signal Processing
242