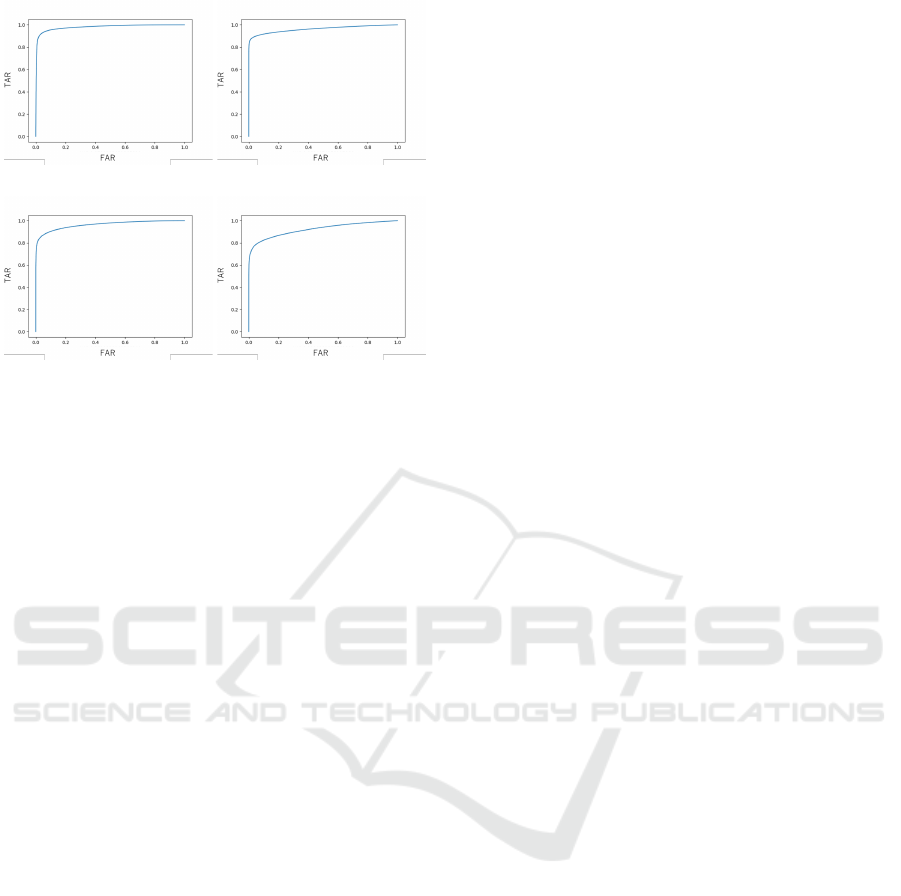
(a) multispectral BTF (b) ordinary BTF
(c) multispectral image (d) RGB image
Figure 13: ROC curves for (a) multispectral BTF, (b) ordi-
nary BTF, (c) RGB image and (d) multispectral image.
In these results, we can confirm that the best dis-
crimination results are obtained with the proposed
method. Thesed results indicate that the classification
performance by the proposed method is higher than
other results using the other features. These results
confirm that the multispectral BTF proposed in this
paper can adequately represent the coating character-
istics of industrial products.
6 CONCLUSION
In this study, a multispectral BTF was proposed to
represent the reflective properties of fine surfaces for
the inspection of industrial products. In addition, a
method for 1-class identification using deep learning
for product inspection using multispectral BTF is pre-
sented. Furthermore, one-class discrimination exper-
iments of actual products were conducted using the
proposed method, and it was confirmed that the mul-
tispectral BTF is effective for the inspection of coat-
ings. In future work, we plan to study not only the
identification of products but also a method for detect-
ing partial abnormalities such as scratches and dirt.
REFERENCES
Akcay, S., Atapour-Abarghouei, A., and Breckon, T. P.
(2018). Ganomaly: Semi-supervised anomaly detec-
tion via adversarial training. In Asian conference on
computer vision, pages 622–637. Springer.
Akc¸ay, S., Atapour-Abarghouei, A., and Breckon, T. P.
(2019). Skip-ganomaly: Skip connected and adversar-
ially trained encoder-decoder anomaly detection. In
2019 International Joint Conference on Neural Net-
works (IJCNN), pages 1–8. IEEE.
An, J. and Cho, S. (2015). Variational autoencoder based
anomaly detection using reconstruction probability.
Special Lecture on IE, 2(1):1–18.
Bergman, L. and Hoshen, Y. (2019). Classification-based
anomaly detection for general data. In International
Conference on Learning Representations.
Bergmann, P., L
¨
owe, S., Fauser, M., Sattlegger, D., and Ste-
ger, C. (2019). Improving unsupervised defect seg-
mentation by applying structural similarity to autoen-
coders.
Cook, R. L. and Torrance, K. E. (1981). A reflectance model
for computer graphics. SIGGRAPH Comput. Graph.,
15(3):307–316.
Dana, K. J., van Ginneken, B., Nayar, S. K., and Koen-
derink, J. J. (1999). Reflectance and texture of real-
world surfaces. ACM Trans. Graph., 18(1):1–34.
Dehaene, D., Frigo, O., Combrexelle, S., and Eline, P.
(2019). Iterative energy-based projection on a normal
data manifold for anomaly localization. In Interna-
tional Conference on Learning Representations.
DOERSCH, C. (2016). Tutorial on variational autoen-
coders. stat, 1050:13.
Goodfellow, I., Pouget-Abadie, J., Mirza, M., Xu, B.,
Warde-Farley, D., Ozair, S., Courville, A., and Ben-
gio, Y. (2014). Generative adversarial nets. In
Advances in neural information processing systems,
pages 2672–2680.
He, K., Zhang, X., Ren, S., and Sun, J. (2016). Deep resid-
ual learning for image recognition. In Proceedings of
the IEEE Conference on Computer Vision and Pattern
Recognition (CVPR).
Matt Pharr and Greg Humphreys (2004). Physically Based
Rendering: From Theory to Implementation. Morgan
Kaufmann.
Minhas, M. S. and Zelek, J. S. (2019). Anomaly detection
in images. CoRR, abs/1905.13147.
Perera, P. and Patel, V. M. (2019). Learning deep features
for one-class classification. IEEE Transactions on Im-
age Processing, 28(11):5450–5463.
Phong, B. T. (1975). Illumination for computer generated
pictures. Commun. ACM, 18(6):311–317.
Radford, A., Metz, L., and Chintala, S. (2015). Unsu-
pervised representation learning with deep convolu-
tional generative adversarial networks. arXiv preprint
arXiv:1511.06434.
Schlegl, T., Seeb
¨
ock, P., Waldstein, S. M., Schmidt-Erfurth,
U., and Langs, G. (2017). Unsupervised anomaly de-
tection with generative adversarial networks to guide
marker discovery. In International conference on in-
formation processing in medical imaging, pages 146–
157. Springer.
Zhang, J., Xie, Y., Liao, Z., Pang, G., Verjans, J., Li,
W., Sun, Z., He, J., Li, Y., Shen, C., et al. (2020).
Viral pneumonia screening on chest x-ray images
using confidence-aware anomaly detection. arXiv:
2003.12338.
VISAPP 2021 - 16th International Conference on Computer Vision Theory and Applications
162