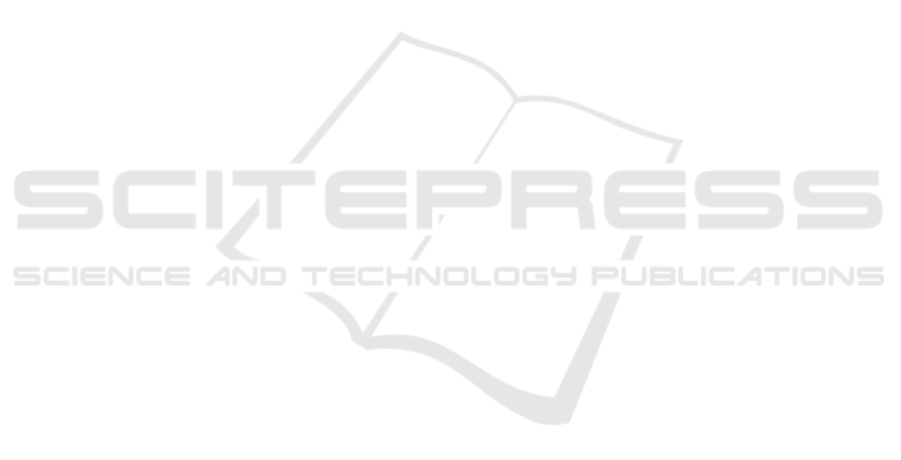
de Campos, T. E., Babu, B. R., and Varma, M. (2009). Char-
acter recognition in natural images. In Proceedings
of the International Conference on Computer Vision
Theory and Applications, Lisbon, Portugal.
Deng, J., Dong, W., Socher, R., Li, L.-J., Li, K., and Fei-
Fei, L. (2009). Imagenet: A large-scale hierarchical
image database. In 2009 IEEE conference on com-
puter vision and pattern recognition, pages 248–255.
Ieee.
Deng, L. (2012). The mnist database of handwritten digit
images for machine learning research [best of the
web]. IEEE Signal Processing Magazine, 29(6):141–
142.
Di Flumeri, G., Borghini, G., Aric
`
o, P., Sciaraffa, N., Lanzi,
P., Pozzi, S., Vignali, V., Lantieri, C., Bichicchi, A.,
Simone, A., et al. (2018). EEG-based mental work-
load neurometric to evaluate the impact of different
traffic and road conditions in real driving settings.
Frontiers in human neuroscience, 12:509.
Dong, X. and Shen, J. (2018). Triplet loss in siamese net-
work for object tracking. In Proceedings of the Euro-
pean Conference on Computer Vision (ECCV), pages
459–474.
Gao, Y., Lee, H. J., and Mehmood, R. M. (2015). Deep
learninig of eeg signals for emotion recognition. In
2015 IEEE International Conference on Multimedia
& Expo Workshops (ICMEW), pages 1–5. IEEE.
Ghimatgar, H., Kazemi, K., Helfroush, M. S., and Aarabi,
A. (2019). An automatic single-channel eeg-based
sleep stage scoring method based on hidden markov
model. Journal of neuroscience methods, page
108320.
He, Y., Eguren, D., Azor
´
ın, J. M., Grossman, R. G., Luu,
T. P., and Contreras-Vidal, J. L. (2018). Brain–
machine interfaces for controlling lower-limb pow-
ered robotic systems. Journal of neural engineering,
15(2):021004.
Huth, A. G., Lee, T., Nishimoto, S., Bilenko, N. Y., Vu,
A. T., and Gallant, J. L. (2016). Decoding the seman-
tic content of natural movies from human brain activ-
ity. Frontiers in Systems Neuroscience, 10:81.
Jolly, B. L. K., Aggrawal, P., Nath, S. S., Gupta, V., Grover,
M. S., and Shah, R. R. (2019). Universal eeg en-
coder for learning diverse intelligent tasks. In 2019
IEEE Fifth International Conference on Multimedia
Big Data (BigMM), pages 213–218. IEEE.
Kapoor, A., Shenoy, P., and Tan, D. (2008). Combining
brain computer interfaces with vision for object cat-
egorization. In 2008 IEEE Conference on Computer
Vision and Pattern Recognition, pages 1–8. IEEE.
Kaya, M. and Bilge, H. S¸. (2019). Deep metric learning: A
survey. Symmetry, 11(9):1066.
Koelstra, S., Muhl, C., Soleymani, M., Lee, J.-S., Yazdani,
A., Ebrahimi, T., Pun, T., Nijholt, A., and Patras, I.
(2011). Deap: A database for emotion analysis; using
physiological signals. IEEE transactions on affective
computing, 3(1):18–31.
Kumar, P., Saini, R., Roy, P. P., Sahu, P. K., and Dogra, D. P.
(2018). Envisioned speech recognition using eeg sen-
sors. Personal and Ubiquitous Computing, 22(1):185–
199.
Linden, D. E. (2005). The p300: where in the brain is it
produced and what does it tell us? The Neuroscientist,
11(6):563–576.
Mehmood, R. M. and Lee, H. J. (2016). Towards human
brain signal preprocessing and artifact rejection meth-
ods. In Int’l Conf. Biomedical Engineering and Sci-
ences, pages 26–31.
Nishimoto, S., Vu, A. T., Naselaris, T., Benjamini, Y., Yu,
B., and Gallant, J. L. (2011). Reconstructing vi-
sual experiences from brain activity evoked by natural
movies. Current Biology, 21(19):1641–1646.
Oweis, R. J. and Abdulhay, E. W. (2011). Seizure classifi-
cation in eeg signals utilizing hilbert-huang transform.
Biomedical engineering online, 10(1):38.
Parekh, V., Subramanian, R., Roy, D., and Jawahar, C.
(2017). An eeg-based image annotation system. In
National Conference on Computer Vision, Pattern
Recognition, Image Processing, and Graphics, pages
303–313. Springer.
Schirrmeister, R. T., Springenberg, J. T., Fiederer, L. D. J.,
Glasstetter, M., Eggensperger, K., Tangermann, M.,
Hutter, F., Burgard, W., and Ball, T. (2017). Deep
learning with convolutional neural networks for eeg
decoding and visualization. Human brain mapping,
38(11):5391–5420.
Simanova, I., Van Gerven, M., Oostenveld, R., and Hagoort,
P. (2010). Identifying object categories from event-
related eeg: toward decoding of conceptual represen-
tations. PloS one, 5(12):e14465.
Spampinato, C., Palazzo, S., Kavasidis, I., Giordano, D.,
Shah, M., and Souly, N. (2016). Deep learning hu-
man mind for automated visual classification. CoRR,
abs/1609.00344.
Stytsenko, K., Jablonskis, E., and Prahm, C. (2011). Eval-
uation of consumer eeg device emotiv epoc. In MEi:
CogSci Conference 2011, Ljubljana.
Tirupattur, P., Rawat, Y. S., Spampinato, C., and Shah, M.
(2018). Thoughtviz: Visualizing human thoughts us-
ing generative adversarial network. New York, NY,
USA. Association for Computing Machinery.
Wang, C., Xiong, S., Hu, X., Yao, L., and Zhang, J. (2012).
Combining features from erp components in single-
trial eeg for discriminating four-category visual ob-
jects. Journal of neural engineering, 9(5):056013.
EEG Classification for Visual Brain Decoding via Metric Learning
167