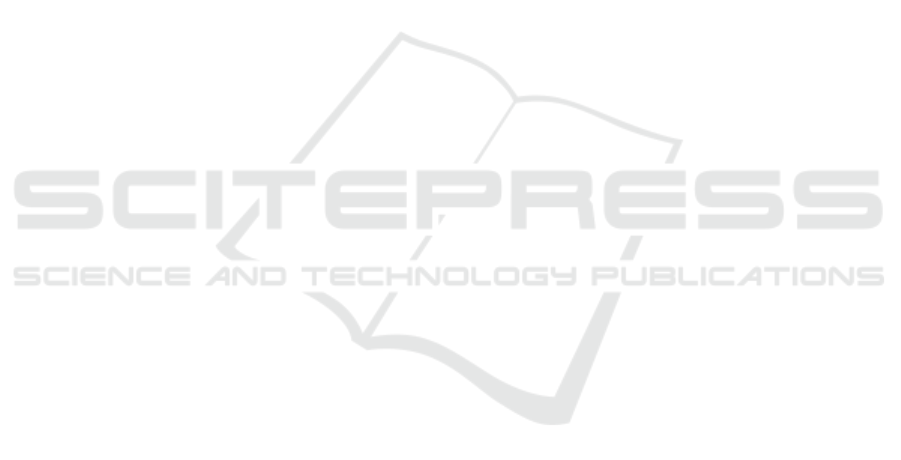
REFERENCES
Aaron, C. G. I. (2010). Dise
˜
no y construcci
´
on de un sistema
para la detecci
´
on de se
˜
nales electromiogr
´
aficas. PhD
thesis, Universidad Aut
´
onoma de Yucat
´
an, Mexico.
Anderson, J. A. (1995). An introduction to neural networks.
MIT press.
Birkedal, L., Collet, T., Dagilis, S., Delavernhe, G., and
Emborg (2002). Pattern recognition of upper-body
electromyography for control of lower limb prosthe-
ses. Institute of Electronic Systems, Aalborg Univer-
sity.
Cabrera, J. S. and Jaramillo, H. F. (2010). Mejora de pro-
cesos para el desarrollo de dispositivos prost
´
eticos de
mano. Ingenium, (21).
Carre
˜
no, I. and Vuskovic, M. (2005). Wavelet-based fea-
ture extraction from prehensile emg signals. 13th
NordicBaltic on Biomedical Engineering and Medical
Physics (NBC’05 UMEA), pages 13–17.
Daniel, L. (2015). Un ejemplo sencillo sobre an
´
alisis de
componentes principales (pca, principal component
analysis). https://dlegorreta.wordpress.com.
Durgesh, K. S. and Lekha, B. (2010). Data classification
using support vector machine. Journal of Theoretical
and Applied Information Technology, 12(1):1–7.
Ferguson, S. and Dunlop, G. R. (2002). Grasp recog-
nition from myoelectric signals. In Proceedings of
the Australasian Conference on Robotics and Automa-
tion, Auckland, New Zealand, volume 1.
Gerdle, B., Karlsson, S., Day, S., and Djupsj
¨
obacka, M.
(1999). Acquisition, processing and analysis of the
surface electromyogram. In Modern techniques in
neuroscience research, pages 705–755. Springer.
Gomero, F. N. C., Campos, F. D. R., and Ch
´
avez,
S. J. Z. (2009). M
´
odulo de detecci
´
on, reg-
istro y presentaci
´
on de se
˜
nales electrooculogr
´
aficas.
Electr
´
onica-UNMSM, (24):3–12.
Gupta, T., Yadav, J., Chaudhary, S., and Agarwal, U.
(2017). Emg pattern classification using neural net-
works. In The International Symposium on Intelligent
Systems Technologies and Applications, pages 232–
242. Springer.
Haihua, L., Xinhao, C., and Yaguang, C. (2005). Wavelet
transform analyzing and real-time learning method for
myoelectric signal in motion discrimination. In Neu-
ral Interface and Control, 2005. Proceedings. 2005
First International Conference on, pages 127–130.
IEEE.
Hargrove, L. J., Englehart, K., and Hudgins, B. (2007). A
comparison of surface and intramuscular myoelectric
signal classification. IEEE transactions on biomedical
engineering, 54(5):847–853.
Hermens, H. and Freriks, B. (1997). The state of the art on
sensors and sensor placement procedures for surface
electromyography: a proposal for sensor placement
procedures. Deliverable of the SENIAM Project.
Hudgins, B., Parker, P., and Scott, R. N. (1993). A new
strategy for multifunction myoelectric control. IEEE
Transactions on Biomedical Engineering, 40(1):82–
94.
Irastorza-Landa, N., Sarasola-Sanz, A., Shiman, F., L
´
opez-
Larraz, E., Klein, J., Valencia, D., Belloso, A.,
Morin, F., Birbaumer, N., and Ramos-Murguialday,
A. (2017). Emg discrete classification towards a my-
oelectric control of a robotic exoskeleton in motor re-
habilitation. In Converging Clinical and Engineering
Research on Neurorehabilitation II, pages 159–163.
Springer.
Kazamel, M. and Warren, P. P. (2017). History of elec-
tromyography and nerve conduction studies: A trib-
ute to the founding fathers. Journal of Clinical Neu-
roscience, 43:54–60.
Kehri, V., Ingle, R., Awale, R., and Oimbe, S. (2016). Tech-
niques of emg signal analysis and classification of
neuromuscular diseases. In International Conference
on Communication and Signal Processing 2016 (IC-
CASP 2016). Atlantis Press.
Kilby, J. and Hosseini, H. G. (2004). Wavelet analysis of
surface electromyography signals. In Engineering in
Medicine and Biology Society, 2004. IEMBS’04. 26th
Annual International Conference of the IEEE, vol-
ume 1, pages 384–387. IEEE.
Kurkin, S. A., Khorev, V., Pitsik, E. N., Maksimenko, V. A.,
and Hramov, A. E. (2019). The approach to the detec-
tion of the movement precursor by electromyographic
signals. In ICINCO (1), pages 276–280.
Liu, Y. (2017). Statistical inference in principal component
analysis based on statistical theory. Italian Journal of
Pure and Applied Mathematics, (37):565–574.
Mallat, S. (1991). Zero-crossings of a wavelet trans-
form. IEEE Transactions on Information theory,
37(4):1019–1033.
Merletti, R. and Parker, P. A. (2004). Electromyography:
physiology, engineering, and non-invasive applica-
tions, volume 11. John Wiley & Sons.
Mora Rubio, A., Alzate Grisales, J. A., Tabares-Soto,
R., Orozco-Arias, S., Jim
´
enez Var
´
on, C. F., and
Padilla Buritic’a, J. I. (2020). Identification
of hand movements from electromyographic sig-
nals using machine learning. Preprints. DOI:
10.20944/preprints202002.0443.v1.
MyoWare. (2015). Myoware
TM
muscle sensor (at-04-001)
Datasheet. https://cdn.sparkfun.com/datasheets/
Sensors/Biometric/MyowareUserManualAT-04-001.
pdf.
Quantstart. (2014). Support vector machines: A guide
for beginners. https://www.quantstart.com/articles/
Support-Vector-Machines-A-Guide-for-Beginners/.
Quinay
´
as-Burgos, C. A. and Gaviria-L
´
opez, C. A. (2015).
Sistema de identificaci
´
on de intenci
´
on de movimiento
para el control mioel
´
ectrico de una pr
´
otesis de mano
rob
´
otica. Ingenier
´
ıa y Universidad, 19(1).
Satapathy, S. C., Raju, K. S., Shyamala, K., Krishna, D. R.,
and Favorskaya, M. N. (2019). Advances in Decision
Sciences, Image Processing, Security and Computer
Vision: International Conference on Emerging Trends
in Engineering (ICETE), Vol. 1, volume 3. Springer.
Torrence, C. and Compo, G. P. (1998). A practical guide to
wavelet analysis. Bulletin of the American Meteoro-
logical society, 79(1):61–78.
BIOSIGNALS 2021 - 14th International Conference on Bio-inspired Systems and Signal Processing
250