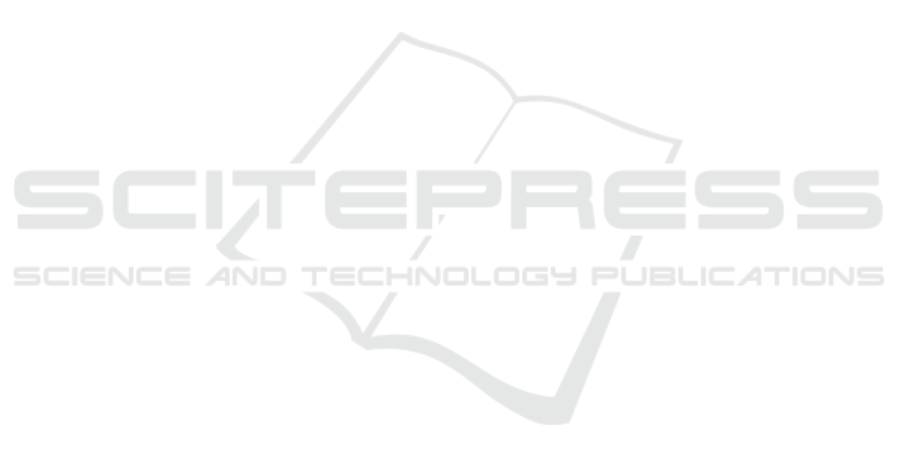
5 CONCLUSION
In this work, we propose a deep upsampling atten-
tion network (UAN) for accurate SISR. Specifically,
the residual attention groups (RAGs) based structure
allows UAN to capture the structure and frequency in-
formation by rescaling spatial- and channel-wise fea-
tures. Meanwhile, RAG allows abundant residual in-
formation to be bypassed through non-local skip con-
nections, making the network more effective. Further-
more, in addition to improve the ability of our model,
we propose an upsampling attention block (UAB) to
adaptively combine parallel upsampled features by
considering correlations among them. Experimental
results on standard benchmarks show that our UAN
achieves better accuracy and visual quality over state-
of-the-art methods.
ACKNOWLEDGEMENTS
This work is funded by the National Key Research
and Development Program of China under the pro-
gram number 2017YFC0810200.
REFERENCES
Freeman, W.T., Pasztor, E.C., and Carmichael, O.T. (2000).
Learning low-level vision. In International Journal of
Computer Vision (IJCV), pages 25–47. Springer.
Dong, C., Loy, C., He, K., and Tang, X. (2014). Learn-
ing a deep convolutional network for image super-
resolution. In European Conference on Computer Vi-
sion (ECCV), pages 184–199. Springer.
Kim, J., Lee, J., and Lee, K. (2016). Accurate image super-
resolution using very deep convolutional networks. In
Conference on Computer Vision and Pattern Recogni-
tion (CVPR), pages 1646–1654. IEEE.
Lai, W., Huang, J., Ahuja, N., and Yang, M. (2017). Deep
laplacian pyramid networks for fast and accurate su-
perresolution. In Conference on Computer Vision and
Pattern Recognition (CVPR), pages 624–632. IEEE.
Dong, C., Loy, C., and Tang, X. (2016). Deeply recursive
convolutional network for image super-resolution. In
European Conference on Computer Vision (ECCV),
pages 1637–1645. Springer.
He, K., Zhang, X., Ren, S., and Sun, J. (2016). Deep
residual learning for image recognition. In Conference
on Computer Vision and Pattern Recognition (CVPR),
pages 770–778. IEEE.
Lim, B., Son, S., Kim, H., Nah, S., and Lee, K. (2017).
Enhanced deep residual networks for single image
super-resolution. In Computer Society Conference on
Computer Vision and Pattern Recognition Workshops
(CVPRW), pages 136–144. IEEE.
Muhammad, H., Shakhnarovich, G., and Ukita, N. (2018).
Deep back-projection networks for super-resolution.
In Conference on Computer Vision and Pattern Recog-
nition (CVPR), pages 1664–1673. IEEE.
Zhang, Y., Li, K., Li, K., Wang, L., Zhong, B., and Fu, Y.
(2018). Image super-resolution using very deep resid-
ual channel attention networks. In European Con-
ference on Computer Vision (ECCV), pages 286–301.
Springer.
Dai, T., Cai, J., Zhang, Y., Xia, S., and Zhang, L.
(2019). Second-order attention network for single im-
age super-resolution. In Conference on Computer Vi-
sion and Pattern Recognition (CVPR), pages 11065–
11074. IEEE.
Shi, W., Caballero, J., Huszar, F., Totz, J., Aitken, A.,
Bishop, R., Rueckert, D., and Wang, Z. (2016). Real-
time single image and video super-resolution using an
efficient sub-pixel convolutional neural network. In
Conference on Computer Vision and Pattern Recogni-
tion (CVPR), pages 1874–1883. IEEE.
Hu, J., Shen, L., and Sun, G. (2018). Squeeze-and-
excitation networks. In Conference on Computer Vi-
sion and Pattern Recognition (CVPR), pages 7132–
7141. IEEE.
Woo, S., Park, J., Lee, J., and I.S., K. (2018). Cbam:
Convolutional block attention module. In European
Conference on Computer Vision (ECCV), pages 3–19.
Springer.
Timofte, R., Agustsson, E., Gool, L., Yang, M., and Zhang,
L. (2017). Ntire 2017 challenge on single image
super-resolution: Methods and results. In Computer
Society Conference on Computer Vision and Pattern
Recognition Workshops (CVPRW), pages 114–125.
IEEE.
Bevilacqua, M., Roumy, A., Guillemot, C., and Morel,
A. (2012). Low-complexity single-image super-
resolution based on nonnegative neighbor embedding.
In The British Machine Vision Conference (BMVC),
pages 135.1–135.1. Springer.
Zeyde, R., Elad, M., and Protter, M. (2010). On single im-
age scale-up using sparse-representations. In 7th Int.
Conf. Curves Surf., pages 711–730.
Martin, D., Fowlkes, C., Tal, D., and Malik, J. (2002). A
database of human segmented natural images and its
application to evaluating segmentation algorithms and
measuring ecological statistics. In International Con-
ference on Computer Vision (ICCV), pages 416–423.
IEEE.
Huang, J., Singh, A., and Ahuja, N. (2015). Single image
super-resolution from transformed self-exemplars. In
Conference on Computer Vision and Pattern Recogni-
tion (CVPR), pages 5197–5206. IEEE.
VISAPP 2021 - 16th International Conference on Computer Vision Theory and Applications
406