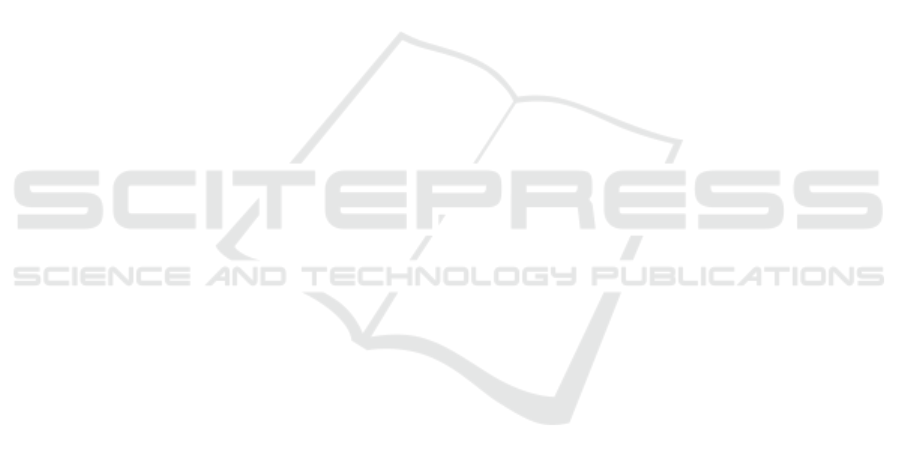
airfoils on the annual energy production of wind tur-
bines. Renewable energy, 115:817–823.
ISO25178 (2012). Iso25178(2012) geometrical product
specifications (gps) — surface texture: Areal — part
2: Terms, definitions and surface texture parame-
ters. https://www.iso.org/obp/ui/#iso:std:iso:25178:
-2:ed-1:v1:en. Accessed: 2020-08-03.
ISO4287 (1997). Iso4287(1997) geometrical product spec-
ifications (gps) — surface texture: Profile method
— terms, definitions and surface texture parameters.
https://www.iso.org/standard/10132.html. Accessed:
2020-08-03.
ISO6344 (1998). Iso6344(1998) coated abrasives — grain
size analysis parts 1-3. https://www.iso.org/standard/
12643.html. Accessed: 2020-08-03.
Langel, C. M., Chow, R., Hurley, O. F., Van Dam, C.
C. P., Maniaci, D. C., Ehrmann, R. S., and White,
E. B. (2015). Analysis of the Impact of Leading Edge
Surface Degradation on Wind Turbine Performance.
In 33rd Wind Energy Symposium, Reston, Virginia.
American Institute of Aeronautics and Astronautics.
Langel, C. M., Chow, R., Van Dam, C., and Maniaci, D. C.
(2017). Rans based methodology for predicting the
influence of leading edge erosion on airfoil perfor-
mance. Technical report, Sandia National Lab.(SNL-
NM), Albuquerque, NM (United States).
Lowe, D. G. (2004). Distinctive image features from scale-
invariant keypoints. International journal of computer
vision, 60(2):91–110.
Martin, R. W., Sabato, A., Schoenberg, A., Giles, R. H., and
Niezrecki, C. (2018). Comparison of nondestructive
testing techniques for the inspection of wind turbine
blades’ spar caps. Wind Energy, 21(11):980–996.
Marzuki, O. F., Rafie, A. S. M., Romli, F. I., and Ahmad,
K. A. (2018). Magnus wind turbine: the effect of
sandpaper surface roughness on cylinder blades. Acta
Mechanica, 229(1):71–85.
Moreno, S., Pe
˜
na, M., Toledo, A., Trevi
˜
no, R., and Ponce,
H. (2018). A new vision-based method using deep
learning for damage inspection in wind turbine blades.
In 2018 15th International Conference on Electrical
Engineering, Computing Science and Automatic Con-
trol (CCE), pages 1–5. IEEE.
Nielsen, M., Nikolov, I., Kruse, E., Garnæs, J., and Madsen,
C. (2020). High-resolution structure-from-motion for
quantitative measurement of leading-edge roughness.
Energies, 13(15).
Nikolov, I. and Madsen, C. (2016). Benchmarking close-
range structure from motion 3d reconstruction soft-
ware under varying capturing conditions. In Euro-
Mediterranean Conference, pages 15–26. Springer.
Nikolov, I. and Madsen, C. (2020). Rough or noisy? metrics
for noise estimation in sfm reconstructions. Sensors,
20(19):5725.
Osada, R., Funkhouser, T., Chazelle, B., and Dobkin, D.
(2002). Shape distributions. ACM Transactions on
Graphics (TOG), 21(4):807–832.
Ozyesil, O., Voroninski, V., Basri, R., and Singer, A.
(2017). A survey of structure from motion. arXiv
preprint arXiv:1701.08493.
Peng, L. and Liu, J. (2018). Detection and analysis of large-
scale wt blade surface cracks based on uav-taken im-
ages. IET Image Processing, 12(11):2059–2064.
Rublee, E., Rabaud, V., Konolige, K., and Bradski, G.
(2011). Orb: An efficient alternative to sift or surf.
In 2011 International conference on computer vision,
pages 2564–2571. Ieee.
Schramm, M., Rahimi, H., Stoevesandt, B., and Tangager,
K. (2017). The Influence of Eroded Blades on Wind
Turbine Performance Using Numerical Simulations.
Energies, 10(9):1420.
Shihavuddin, A., Chen, X., Fedorov, V., Nymark Chris-
tensen, A., Andre Brogaard Riis, N., Branner, K.,
Bjorholm Dahl, A., and Reinhold Paulsen, R. (2019).
Wind turbine surface damage detection by deep
learning aided drone inspection analysis. Energies,
12(4):676.
Triggs, B., McLauchlan, P. F., Hartley, R. I., and Fitzgibbon,
A. W. (1999). Bundle adjustment—a modern synthe-
sis. In International workshop on vision algorithms,
pages 298–372. Springer.
Wang, L. and Zhang, Z. (2017). Automatic Detection of
Wind Turbine Blade Surface Cracks Based on UAV-
Taken Images. IEEE Transactions on Industrial Elec-
tronics, 64(9):7293–7303.
Weinmann, M., Jutzi, B., Hinz, S., and Mallet, C. (2015).
Semantic point cloud interpretation based on optimal
neighborhoods, relevant features and efficient classi-
fiers. ISPRS Journal of Photogrammetry and Remote
Sensing, 105:286–304.
Xu, D., Wen, C., and Liu, J. (2019). Wind turbine blade sur-
face inspection based on deep learning and uav-taken
images. Journal of Renewable and Sustainable En-
ergy, 11(5):053305.
Zhang, D., Burnham, K., Mcdonald, L., Macleod, C., Do-
bie, G., Summan, R., and Pierce, G. (2017a). Remote
inspection of wind turbine blades using uav with pho-
togrammetry payload. In 56th Annual British Confer-
ence of Non-Destructive Testing-NDT 2017.
Zhang, D., Watson, R., Dobie, G., MacLeod, C., Khan,
A., and Pierce, G. (2020). Quantifying impacts on
remote photogrammetric inspection using unmanned
aerial vehicles. Engineering Structures, page 109940.
Zhang, Y., Yuan, X., Fang, Y., and Chen, S. (2017b).
Uav low altitude photogrammetry for power line
inspection. ISPRS International Journal of Geo-
Information, 6(1):14.
Zhou, Q.-Y., Park, J., and Koltun, V. (2018). Open3D:
A modern library for 3D data processing.
arXiv:1801.09847.
VISAPP 2021 - 16th International Conference on Computer Vision Theory and Applications
808