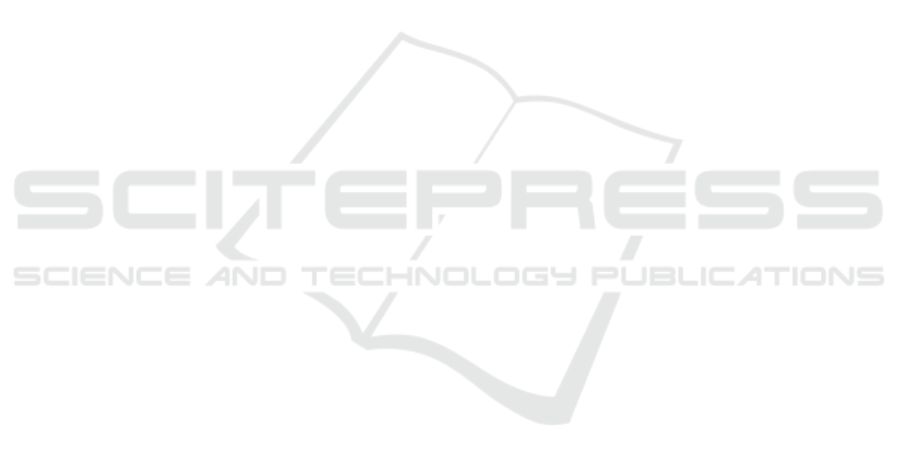
videos. Our pattern theory representation also forms
the basis for unsupervised temporal video segmenta-
tion. Through extensive experiments, we demonstrate
that we obtain state-of-the-art performance on the un-
supervised gaze prediction task and provide compet-
itive performance on the unsupervised temporal seg-
mentation task on egocentric videos.
ACKNOWLEDGEMENT
This research was supported in part by the US Na-
tional Science Foundation grant IIS 1955230.
REFERENCES
Aakur, S., de Souza, F., and Sarkar, S. (2019). Generating
open world descriptions of video using common sense
knowledge in a pattern theory framework. Quarterly
of Applied Mathematics, 77(2):323–356.
Aakur, S. N. and Sarkar, S. (2019). A perceptual prediction
framework for self supervised event segmentation. In
Proceedings of the IEEE Conference on Computer Vi-
sion and Pattern Recognition, pages 1197–1206.
Brox, T. and Malik, J. (2010). Large displacement optical
flow: descriptor matching in variational motion esti-
mation. IEEE Transactions on Pattern Analysis and
Machine Intelligence, 33(3):500–513.
Bruce, N. and Tsotsos, J. (2006). Saliency based on infor-
mation maximization. In Advances in Neural Infor-
mation Processing Systems, pages 155–162.
Fathi, A., Li, Y., and Rehg, J. M. (2012). Learning to recog-
nize daily actions using gaze. In European Conference
on Computer Vision, pages 314–327. Springer.
Grenander, U. (1996). Elements of pattern theory. JHU
Press.
Harel, J., Koch, C., and Perona, P. (2007). Graph-based
visual saliency. In Advances in Neural Information
Processing Systems, pages 545–552.
Horstmann, G. and Herwig, A. (2015). Surprise attracts
the eyes and binds the gaze. Psychonomic Bulletin &
Review, 22(3):743–749.
Horstmann, G. and Herwig, A. (2016). Novelty biases at-
tention and gaze in a surprise trial. Attention, Percep-
tion, & Psychophysics, 78(1):69–77.
Hossein Khatoonabadi, S., Vasconcelos, N., Bajic, I. V., and
Shan, Y. (2015). How many bits does it take for a stim-
ulus to be salient? In Proceedings of the IEEE Con-
ference on Computer Vision and Pattern Recognition,
pages 5501–5510.
Hou, X., Harel, J., and Koch, C. (2011). Image signature:
Highlighting sparse salient regions. IEEE Transac-
tions on Pattern Analysis and Machine Intelligence,
34(1):194–201.
Huang, C.-M., Andrist, S., Saupp
´
e, A., and Mutlu, B.
(2015). Using gaze patterns to predict task intent in
collaboration. Frontiers in Psychology, 6:1049.
Huang, Y., Cai, M., Li, Z., and Sato, Y. (2018). Predicting
gaze in egocentric video by learning task-dependent
attention transition. In Proceedings of the European
Conference on Computer Vision (ECCV), pages 754–
769.
Itti, L. and Baldi, P. F. (2006). Bayesian surprise attracts
human attention. In Advances in Neural Information
Processing Systems, pages 547–554.
Itti, L. and Koch, C. (2000). A saliency-based search mech-
anism for overt and covert shifts of visual attention.
Vision Research, 40(10-12):1489–1506.
Ji, S., Xu, W., Yang, M., and Yu, K. (2012). 3d convolu-
tional neural networks for human action recognition.
IEEE transactions on pattern analysis and machine
intelligence, 35(1):221–231.
Jiang, M., Huang, S., Duan, J., and Zhao, Q. (2015). Sali-
con: Saliency in context. In Proceedings of the IEEE
Conference on Computer Vision and Pattern Recogni-
tion, pages 1072–1080.
Lea, C., Vidal, R., Reiter, A., and Hager, G. D. (2016).
Temporal convolutional networks: A unified approach
to action segmentation. In European Conference on
Computer Vision, pages 47–54. Springer.
Leboran, V., Garcia-Diaz, A., Fdez-Vidal, X. R., and Pardo,
X. M. (2016). Dynamic whitening saliency. IEEE
Transactions on Pattern Analysis and Machine Intel-
ligence, 39(5):893–907.
Li, Y., Fathi, A., and Rehg, J. M. (2013). Learning to predict
gaze in egocentric video. In Proceedings of the IEEE
International Conference on Computer Vision, pages
3216–3223.
Redmon, J., Divvala, S., Girshick, R., and Farhadi, A.
(2016). You only look once: Unified, real-time object
detection. In Proceedings of the IEEE Conference on
Computer Vision and Pattern Recognition, pages 779–
788.
Riche, N., Duvinage, M., Mancas, M., Gosselin, B., and
Dutoit, T. (2013). Saliency and human fixations:
State-of-the-art and study of comparison metrics. In
Proceedings of the IEEE International Conference on
Computer Vision, pages 1153–1160.
Singh, S., Arora, C., and Jawahar, C. (2016). First person
action recognition using deep learned descriptors. In
Proceedings of the IEEE Conference on Computer Vi-
sion and Pattern Recognition, pages 2620–2628.
Soomro, K., Zamir, A. R., and Shah, M. (2012). Ucf101:
A dataset of 101 human actions classes from videos in
the wild. arXiv preprint arXiv:1212.0402.
Treisman, A. M. and Gelade, G. (1980). A feature-
integration theory of attention. Cognitive Psychology,
12(1):97–136.
Zacks, J. M., Tversky, B., and Iyer, G. (2001). Perceiv-
ing, remembering, and communicating structure in
events. Journal of Experimental Psychology: Gen-
eral, 130(1):29.
Zhang, M., Teck Ma, K., Hwee Lim, J., Zhao, Q., and Feng,
J. (2017). Deep future gaze: Gaze anticipation on ego-
centric videos using adversarial networks. In Proceed-
ings of the IEEE Conference on Computer Vision and
Pattern Recognition, pages 4372–4381.
VISAPP 2021 - 16th International Conference on Computer Vision Theory and Applications
942