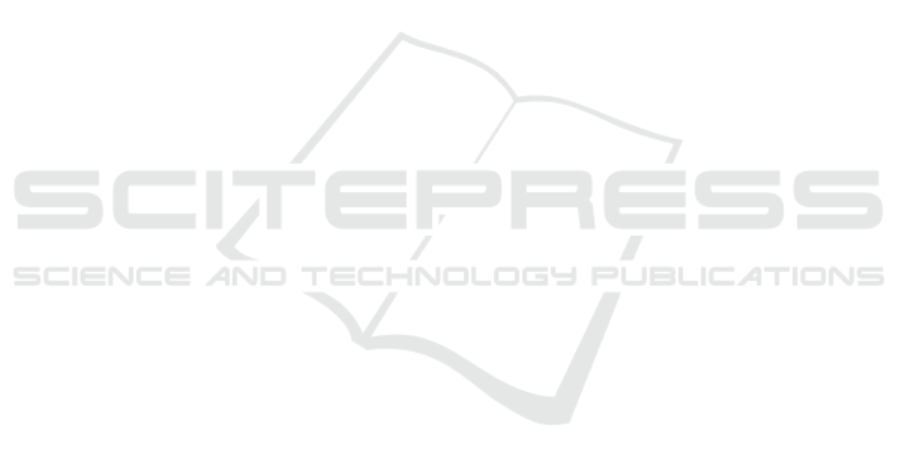
by-tracking. In 2008 IEEE Conference on computer
vision and pattern recognition, pages 1–8. IEEE.
Bergamini, L., Porrello, A., Dondona, A. C., Del Negro, E.,
Mattioli, M., D’alterio, N., and Calderara, S. (2018).
Multi-views embedding for cattle re-identification. In
2018 14th International Conference on Signal-Image
Technology & Internet-Based Systems (SITIS), pages
184–191. IEEE.
Bergamini, L., Trachtman, A. R., Palazzi, A., Negro,
E. D., Capobianco Dondona, A., Marruchella, G., and
Calderara, S. (2019). Segmentation guided scoring
of pathological lesions in swine through cnns. In
New Trends in Image Analysis and Processing - ICIAP
2019 - ICIAP International Workshops, pages 352–
360. Springer.
Bernardin, K. and Stiefelhagen, R. (2008). Evaluating mul-
tiple object tracking performance: the clear mot met-
rics. EURASIP Journal on Image and Video Process-
ing, 2008:1–10.
Bolkensteyn, D. (2016). Vaticjs. https://github.com/
dbolkensteyn/vatic.js.
Bolme, D. S., Beveridge, J. R., Draper, B. A., and Lui, Y. M.
(2010). Visual object tracking using adaptive corre-
lation filters. In 2010 IEEE computer society con-
ference on computer vision and pattern recognition,
pages 2544–2550. IEEE.
Br
¨
unger, J., Gentz, M., Traulsen, I., and Koch, R. (2020).
Panoptic instance segmentation on pigs. arXiv
preprint arXiv:2005.10499.
Chen, C., Zhu, W., Ma, C., Guo, Y., Huang, W., and
Ruan, C. (2017). Image motion feature extraction
for recognition of aggressive behaviors among group-
housed pigs. Computers and Electronics in Agricul-
ture, 142:380–387.
Costa, A., Ismayilova, G., Borgonovo, F., Viazzi, S., Berck-
mans, D., and Guarino, M. (2014). Image-processing
technique to measure pig activity in response to cli-
matic variation in a pig barn. Animal Production Sci-
ence, 54(8):1075–1083.
Cowton, J., Kyriazakis, I., and Bacardit, J. (2019). Au-
tomated individual pig localisation, tracking and be-
haviour metric extraction using deep learning. IEEE
Access, 7:108049–108060.
D’Eath, R. B., Jack, M., Futro, A., Talbot, D., Zhu, Q., Bar-
clay, D., and Baxter, E. M. (2018). Automatic early
warning of tail biting in pigs: 3d cameras can detect
lowered tail posture before an outbreak. PloS one,
13(4):e0194524.
Deng, J., Dong, W., Socher, R., Li, L.-J., Li, K., and Fei-
Fei, L. (2009). Imagenet: A large-scale hierarchical
image database. In 2009 IEEE conference on com-
puter vision and pattern recognition, pages 248–255.
Ieee.
Fern
´
andez-Carri
´
on, E., Mart
´
ınez-Avil
´
es, M., Ivorra, B.,
Mart
´
ınez-L
´
opez, B., Ramos,
´
A. M., and S
´
anchez-
Vizca
´
ıno, J. M. (2017). Motion-based video monitor-
ing for early detection of livestock diseases: The case
of african swine fever. PloS one, 12(9):e0183793.
Fleuret, F., Berclaz, J., Lengagne, R., and Fua, P. (2007).
Multicamera people tracking with a probabilistic oc-
cupancy map. IEEE transactions on pattern analysis
and machine intelligence, 30(2):267–282.
Girshick, R., Donahue, J., Darrell, T., and Malik, J. (2014).
Rich feature hierarchies for accurate object detec-
tion and semantic segmentation. In Proceedings of
the IEEE conference on computer vision and pattern
recognition, pages 580–587.
He, K., Gkioxari, G., Doll
´
ar, P., and Girshick, R. (2017).
Mask r-cnn. In Proceedings of the IEEE international
conference on computer vision, pages 2961–2969.
He, K., Zhang, X., Ren, S., and Sun, J. (2016). Deep resid-
ual learning for image recognition. In Proceedings of
the IEEE conference on computer vision and pattern
recognition, pages 770–778.
Kuhn, H. W. (1955). The hungarian method for the as-
signment problem. Naval research logistics quarterly,
2(1-2):83–97.
Li, D., Chen, Y., Zhang, K., and Li, Z. (2019). Mounting
behaviour recognition for pigs based on deep learning.
Sensors, 19(22):4924.
Li, D., Zhang, K., Li, Z., and Chen, Y. (2020). A spatiotem-
poral convolutional network for multi-behavior recog-
nition of pigs. Sensors, 20(8):2381.
Liu, C., Zhang, R., and Guo, L. (2019a). Part-pose guided
amur tiger re-identification. In iccvw.
Liu, N., Zhao, Q., Zhang, N., Cheng, X., and Zhu, J.
(2019b). Pose-guided complementary features learn-
ing for amur tiger re-identification. In iccvw.
Liu, W., Anguelov, D., Erhan, D., Szegedy, C., Reed, S.,
Fu, C.-Y., and Berg, A. C. (2016). Ssd: Single shot
multibox detector. In European conference on com-
puter vision, pages 21–37. Springer.
Milan, A., Leal-Taix
´
e, L., Reid, I., Roth, S., and Schindler,
K. (2016). Mot16: A benchmark for multi-object
tracking. arXiv preprint arXiv:1603.00831.
Ming-Kuei Hu (1962). Visual pattern recognition by mo-
ment invariants. IRE Transactions on Information
Theory, 8(2):179–187.
Mittek, M., Psota, E. T., Carlson, J. D., P
´
erez, L. C.,
Schmidt, T., and Mote, B. (2017). Tracking of group-
housed pigs using multi-ellipsoid expectation max-
imisation. IET Computer Vision, 12(2):121–128.
Nasirahmadi, A., Edwards, S. A., and Sturm, B. (2017).
Implementation of machine vision for detecting be-
haviour of cattle and pigs. Livestock Science, 202:25–
38.
Norouzzadeh, M. S., Nguyen, A., Kosmala, M., Swanson,
A., Palmer, M. S., Packer, C., and Clune, J. (2018).
Automatically identifying, counting, and describing
wild animals in camera-trap images with deep learn-
ing. Proceedings of the National Academy of Sciences,
page 201719367.
Pini, S., Cornia, M., Bolelli, F., Baraldi, L., and Cucchiara,
R. (2019). M-VAD Names: a Dataset for Video Cap-
tioning with Naming. Multimedia Tools and Applica-
tions, 78(10):14007–14027.
Pol Marquer, Teresa Rabade, R. F. (2020). Pig farming
in the European Union: considerable variations from
one Member State to another. https://ec.europa.eu/
eurostat/statistics-explained/pdfscache/3688.pdf.
VISAPP 2021 - 16th International Conference on Computer Vision Theory and Applications
532