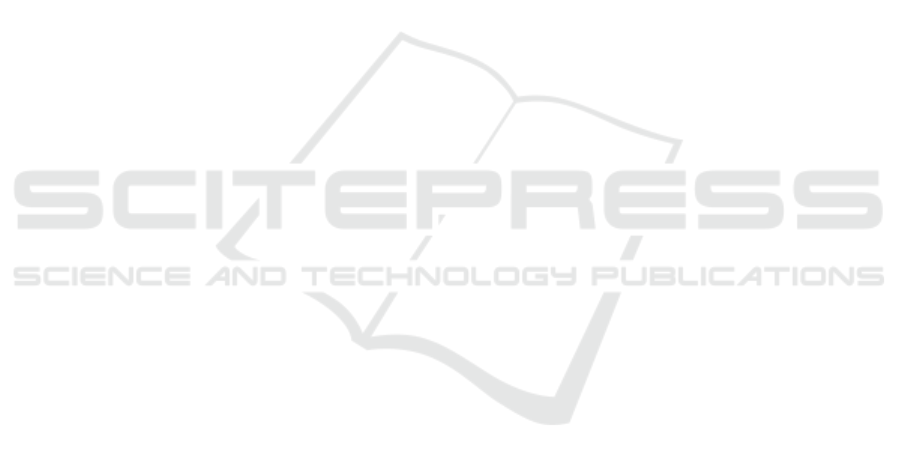
the physiological parameters (HR and HRV) and the
subjective data (difficulty felt) evolved in the same
way. Then, similarly to (Gateau et al., 2018), higher
physiological responses were found in real flight com-
pared to simulated conditions. However, comparisons
between real and virtual reality flights disclosed that
these physiological responses followed a similar pat-
tern between the two conditions.
This study thus mainly contributed to the imple-
mentation of an experimental protocol and the acqui-
sition of experience for real flight and VR measure-
ments. The future experiments will also be conducted
with an eye tracker in both conditions to compare the
pilot’s scan pattern. Indeed, pilot’s attention is a key
issue for flight safety (Peysakhovich et al., 2018; De-
hais et al., 2008; Dehais et al., 2020) and there is a
need to measure to what extent VR can affect it. For
instance, we plan to use portable eye tracking sys-
tem both in the VR environment (Tobii eye tracking
VR system) and real flight environment (Tobii pro
glasses 2). Our goal is to perform basic eye move-
ment (fixation and saccades) as well area of interest
(AOIs) based analyses to compare pilot’s scanning in
the two conditions. Moreover, flight parameters will
also be studied more closely to provide other per-
formance metrics (eg. comparison of the moments
of action on flap management according to the flight
phases, maintaining speed and altitude, ...). Eventu-
ally, other subjective measures (stress, mental work-
load, fatigue) should be collected in the future to bet-
ter interpret the findings.
In order to achieve an almost perfect simulation,
the problem of the hard-to-read anemometer will have
to be solved. This can be a modification of the flight
simulator or a higher resolution helmet (expensive).
The ground texture will have to be richer if future re-
search requires flying under VFR navigation.
ACKNOWLEDGEMENTS
The authors would like to thank Sebastien Scan-
nella, Research Engineer in neuroergonomics at the
DCAS Department of ISAE-SUPAERO, for his pre-
cious help in the analysis of cardiac activity. Many
thanks to St
´
ephane Juaneda, the safety pilot, for his
availability to perform flights and his precious know-
how in flight experimentation. Special thanks to Fab-
rice Bazelot, Franck Yvars and Beno
ˆ
ıt Momier, all
LFCL mechanics, for their help during the configura-
tion of the experiments. A special thanks to all the pi-
lots who accepted to fly under very hot temperatures.
REFERENCES
Banos, R., Botella, C., Perpina, C., Alcaniz, M., Lozano,
J., Osma, J., and Gallardo, M. (2002). Virtual reality
treatment of flying phobia. IEEE Transactions on In-
formation Technology in Biomedicine, 6(3):206–212.
Cha, M., Han, S., Lee, J., and Choi, B. (2012). A virtual
reality based fire training simulator integrated with fire
dynamics data. Fire Safety Journal, 50:12 – 24.
Dehais, F., Causse, M., and Pastor, J. (2008). Embedded
eye tracker in a real aircraft: new perspectives on
pilot/aircraft interaction monitoring. In Proceedings
from The 3rd International Conference on Research
in Air Transportation. Fairfax, USA: Federal Aviation
Administration.
Dehais, F., Dupr
`
es, A., Blum, S., Drougard, N., Scannella,
S., Roy, R. N., and Lotte, F. (2019). Monitoring pilot’s
mental workload using erps and spectral power with a
six-dry-electrode eeg system in real flight conditions.
Sensors, 19(6):1324.
Dehais, F., Juaneda, S., and Peysakhovich, V. (2020). Mon-
itoring eye movements in real flight conditions for
flight training purpose. In 1st International Workshop
on Eye-Tracking in Aviation.
Durantin, G., Gagnon, J.-F., Tremblay, S., and Dehais, F.
(2014). Using near infrared spectroscopy and heart
rate variability to detect mental overload. Behavioural
brain research, 259:16–23.
Gateau, T., Ayaz, H., and Dehais, F. (2018). In silico vs.
over the clouds: on-the-fly mental state estimation of
aircraft pilots, using a functional near infrared spec-
troscopy based passive-bci. Frontiers in human neu-
roscience, 12:187.
Grantcharov, T. P., Kristiansen, V. B., Bendix, J., Bardram,
L., Rosenberg, J., and Funch-Jensen, P. (2004). Ran-
domized clinical trial of virtual reality simulation
for laparoscopic skills training. British Journal of
Surgery, 91(2):146–150.
Hays, R. T., Jacobs, J. W., Prince, C., and Salas, E.
(1992). Flight simulator training effectiveness: A
meta-analysis. Military Psychology, 4(2):63–74.
Hodges, L., Watson, B., Kessler, G., Rothbaum, B., and
Opdyke, D. (1996). Virtually conquering fear of
flying. IEEE Computer Graphics and Applications,
16(6):42–49.
Ismail, A. (2012). Int
´
er
ˆ
et de la variabilit
´
e du rythme car-
diaque comme marqueur de risque. PhD thesis.
Kozak, J. J., Hancock, P. A., Arthur, E. J., and Chrysler,
S. T. (1993). Transfer of training from virtual reality.
Ergonomics, 36(7):777–784.
Labedan, P., Dehais, F., and Peysakhovich, V. (2018). Eval-
uation de l’exp
´
erience de pilotage d’un avion l
´
eger en
r
´
ealit
´
e virtuelle. In ERGO’IA.
Lawrynczyk, A. (2018). Exploring Virtual Reality Flight
Training as a Viable Alternative to Traditional Simu-
lator Flight Training. PhD thesis, Carleton University.
Meshkati, N. (1988). Heart rate variability and men-
tal workload assessment. In Hancock, P. A. and
Meshkati, N., editors, Human Mental Workload, vol-
Virtual Reality for Pilot Training: Study of Cardiac Activity
87